Continuous Action Strategy Optimization of Intelligent Driving Vehicle Based on I-DDPG
2023 International Conference on Artificial Intelligence Innovation (ICAII)(2023)
摘要
Currently, the decision-making algorithms in the field of intelligent driving are mainly based on deep reinforcement learning, with DDPG as an example. In the intelligent driving domain, the DDPG algorithm suffers from issues such as the significant impact of reward function design with changing environmental conditions, unclear criteria for determining positive and negative rewards, low exploration efficiency during model training, and a lack of safety assessment criteria. Therefore, this paper proposes an I-DDPG (Imitation and Deep Deterministic Policy Gradient) model for vehicle autonomous driving decision-making based on a deterministic deep reinforcement learning framework. The SDACM (State-Decision-Action Coordination Making) framework, based on the Markov decision process trained by the model, is established using the I-DDPG algorithm. The reward function in I-DDPG is designed specifically to address driving efficiency and vehicle safety concerns. Expert data is added to the experience replay pool using imitation learning to enhance the model's learning efficiency. Experimental results show that the I-DDPG model exhibits higher safety levels. When the distance between the vehicle and the front obstacle is less than 10m, it decelerates or stops, whereas the DDPG model encounters collisions or overtaking situations. The I-DDPG model demonstrates better stability, with a steadily increasing and stable reward curve. When training with the I-DDPG model, the average reward per epoch is nearly 120 higher than that of DDPG. In terms of learning efficiency, the I-DDPG model outperforms DDPG, with an average step reduction of nearly 32 per epoch.
更多查看译文
关键词
intelligent driving,driving decision,deep reinforcement learning,agent,continuous action
AI 理解论文
溯源树
样例
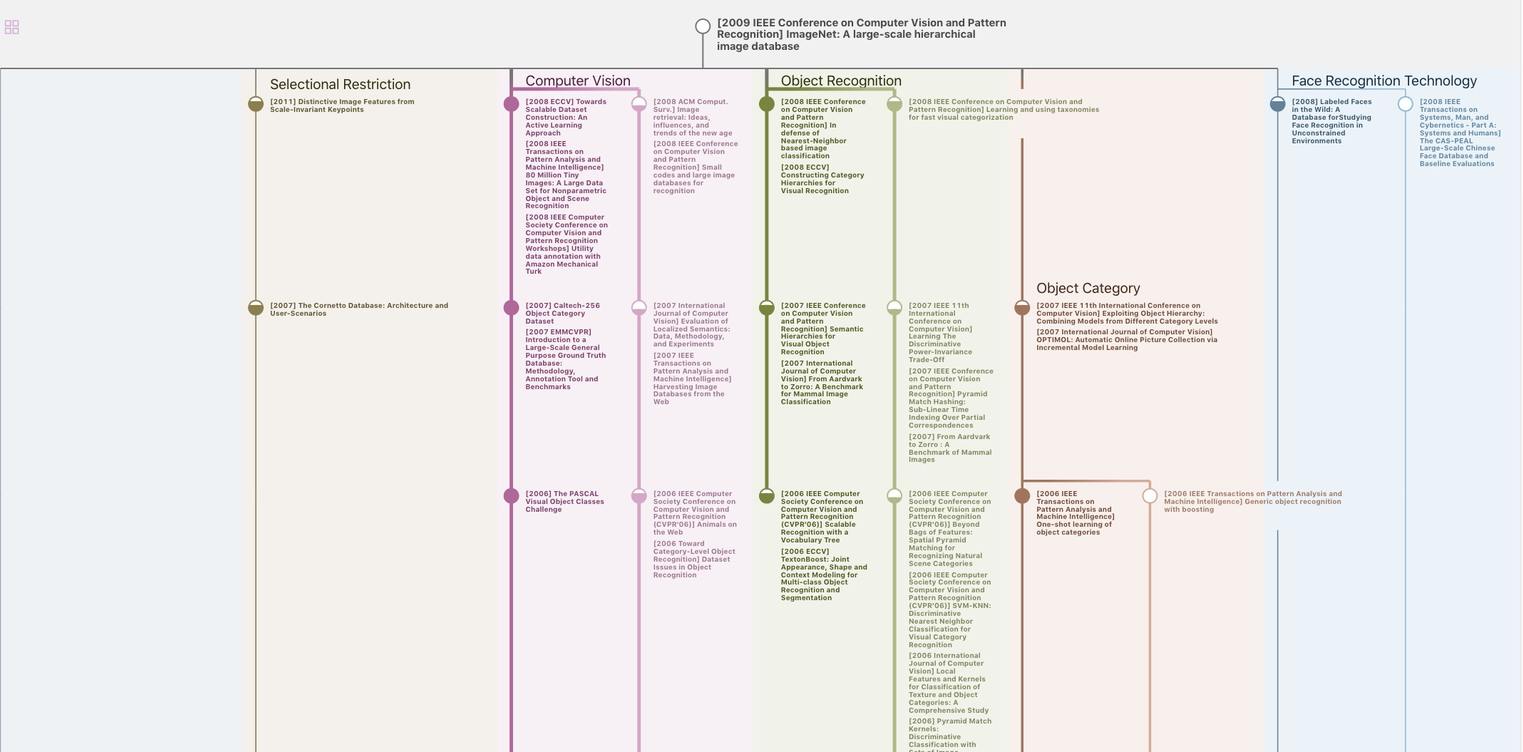
生成溯源树,研究论文发展脉络
Chat Paper
正在生成论文摘要