An Introduction to Bilevel Optimization: Foundations and applications in signal processing and machine learning
IEEE Signal Processing Magazine(2024)
摘要
Recently, bilevel optimization (BLO) has taken center stage in some very exciting developments in the area of signal processing (SP) and machine learning (ML). Roughly speaking, BLO is a classical optimization problem that involves two levels of hierarchy (i.e., upper and lower levels), wherein obtaining the solution to the upper-level problem requires solving the lower-level one. BLO has become popular largely because it is powerful in modeling problems in SP and ML, among others, that involve optimizing nested objective functions. Prominent applications of BLO range from resource allocation for wireless systems to adversarial ML. In this work, we focus on a class of tractable BLO problems that often appear in SP and ML applications. We provide an overview of some basic concepts of this class of BLO problems, such as their optimality conditions, standard algorithms (including their optimization principles and practical implementations) as well as how they can be leveraged to obtain state-of-the-art results for several key SP and ML applications. Further, we discuss some recent advances in BLO theory and its implications for applications, and we point out some limitations of the state of the art that require significant future research efforts. We hope that this article, together with the associated open source BLO toolbox we developed for algorithm benchmarking, can serve to accelerate the adoption of BLO as a generic tool to model, analyze, and innovate on a wide array of emerging SP and ML applications.
更多查看译文
关键词
Machine Learning Applications,Bilevel Optimization,Optimization Problem,Objective Function,Wireless,Class Of Problems,Generative Adversarial Networks,Standard Algorithm,Bellman Equation,Machine Learning Problems,Implications For Applications,Value Function,Machine Learning Models,Stochastic Gradient,Model Weights,Training Loss,Linear Constraints,Stochastic Algorithm,Interior Point Method,Adversarial Attacks,Robust Training,Strongly Convex,General Constraints,Minimax Optimization,Implicit Function Theorem,Stochastic Approximation,Invariant Representation,Deterministic Optimization,Pilot Symbols,Fine-tuned Model
AI 理解论文
溯源树
样例
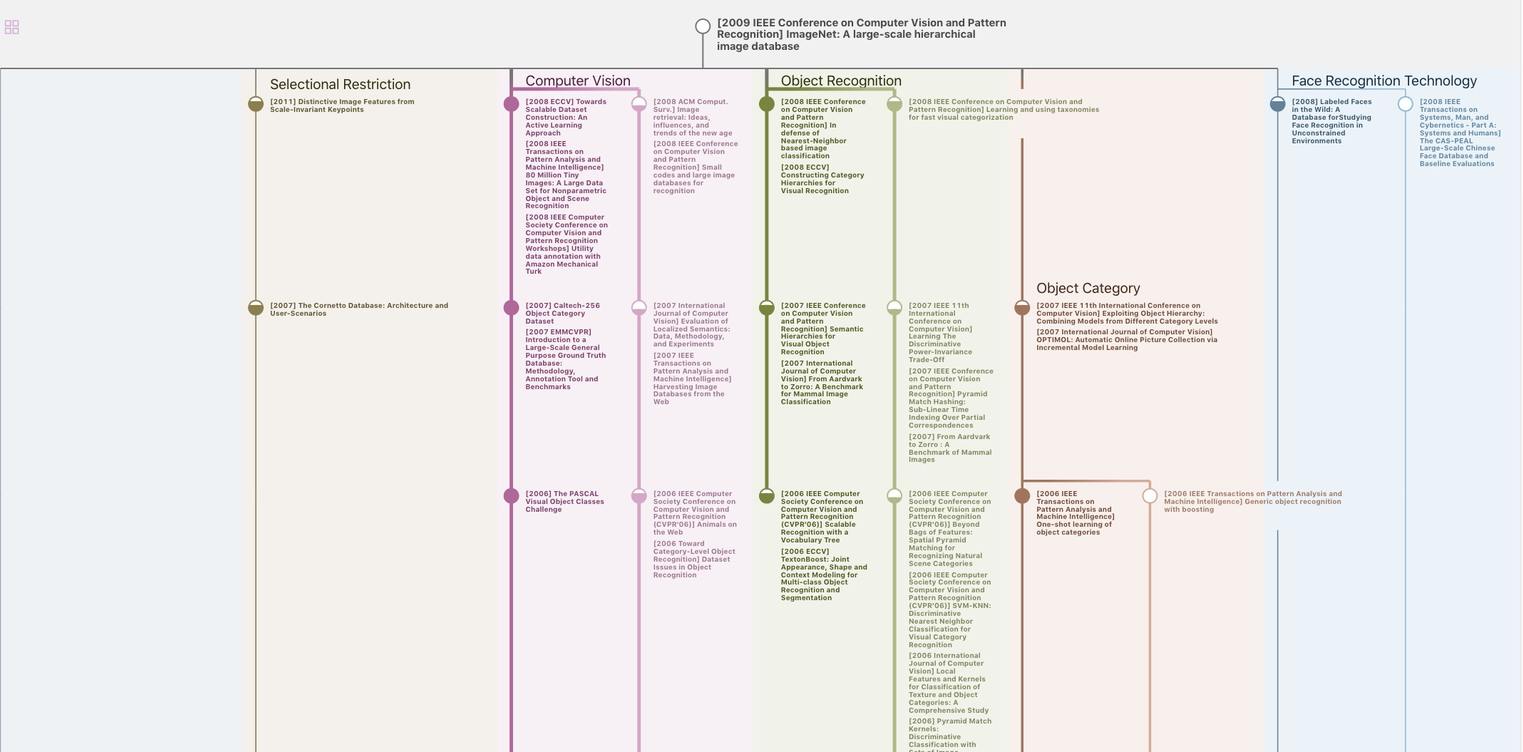
生成溯源树,研究论文发展脉络
Chat Paper
正在生成论文摘要