Short-Term Load Forecasting with TDCI: Temporal Decomposition and Channel Independence
2023 7th International Conference on Electrical, Mechanical and Computer Engineering (ICEMCE)(2023)
摘要
Given the substantial load fluctuations, pronounced stochasticity, and non-linearity influenced by factors like weather and temperature in power load forecasting, we present a short-term load forecasting model based on TDCI. This model introduces several enhancements to boost forecasting accuracy. Firstly, TDCI employs temporal decomposition, separating time-series data into seasonal and trend components, enabling the capture of inherent seasonal patterns and long-term trends that significantly impact load forecasting accuracy. Additionally, the incorporation of locality-sensitive hashing attention efficiently processes relevant temporal patterns, enhancing model performance while reducing computational complexity. Lastly, a channel independence approach is applied to each variable, allowing tailored processing based on individual feature characteristics. This adaptive feature handling ensures effective capture of distinct properties, thereby enhancing forecasting accuracy. Experimental evaluations on a real-world power load dataset demonstrate the superior forecasting precision of our proposed model compared to existing models.
更多查看译文
关键词
short-term load forecasting,sequence decomposition,locality-sensitive hashing attention,channel independence
AI 理解论文
溯源树
样例
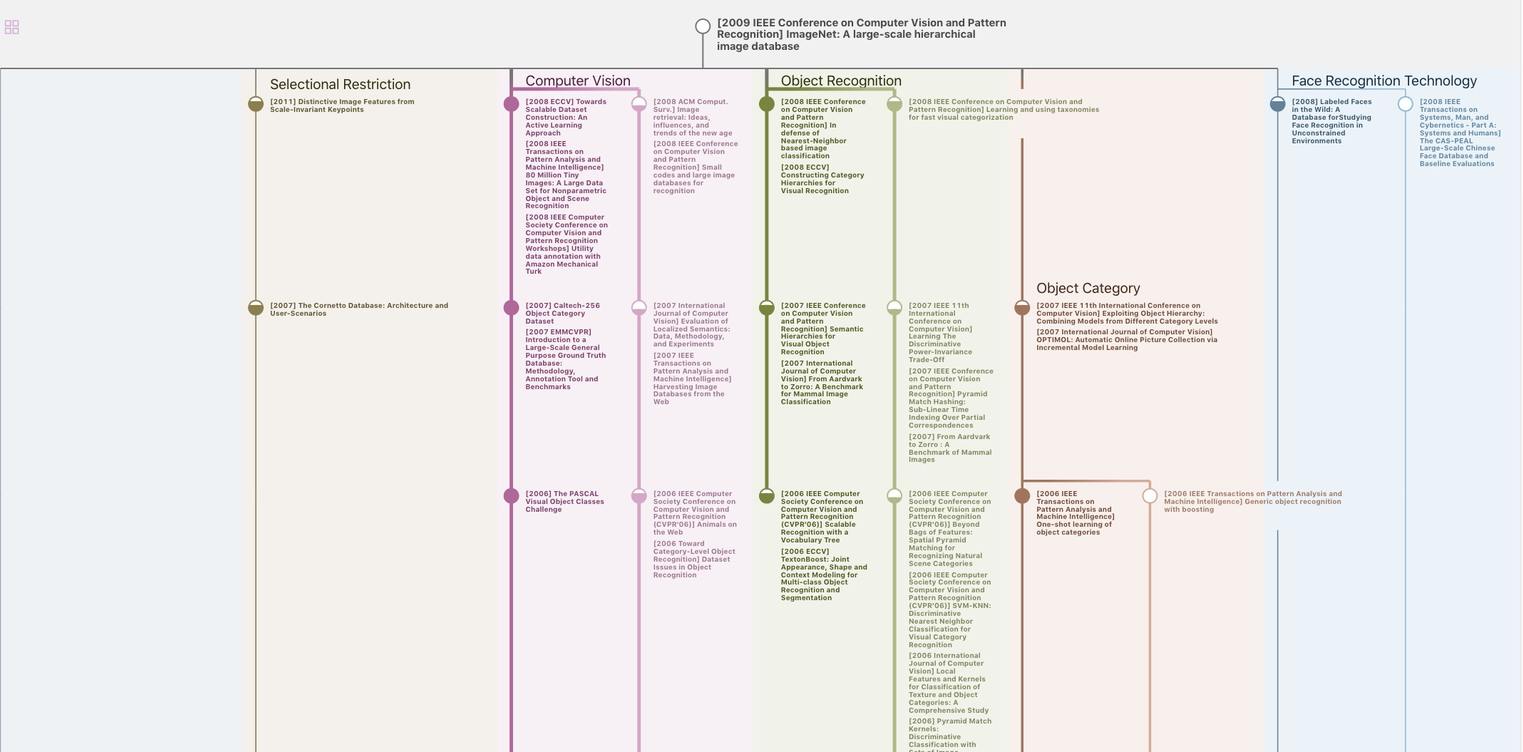
生成溯源树,研究论文发展脉络
Chat Paper
正在生成论文摘要