Entity-Relation Guided Random Walk for Link Prediction in Knowledge Graphs
IEEE Transactions on Computational Social Systems(2024)
摘要
Knowledge graphs (KGs) are structured knowledge bases that represent information as a collection of interconnected entities and relations. Link prediction in KGs aims to infer missing or potential links between entities based on triple facts. Among different link prediction methods, knowledge graph embedding (KGE) has gained widespread popularity, with the goal of learning low-dimensional representations for KGs. However, most present KGE methods struggle to capture both local and global neighborhood information efficiently. Additionally, many hybrid methods have limitations in modeling and capturing interactions between triples. In this article, we propose an entity-relation-guided random walk (ERGRW) method for link prediction in KGs. Unlike conventional approaches that solely focus on entity-based walks, ERGRW creatively introduces relations as objects to walk as well. Inspired by distance-based methods, we design novel random walk rules based on the translation principle within triples. Thus, the ERGRW not only captures local and global neighborhood information but also discovers potential semantic relationships and interactions in the KGs. Furthermore, the encoder–decoder framework of ERGRW is able to learn comprehensive representation and improve link prediction performance. Extensive experiments conducted on four standard datasets demonstrate the superiority of ERGRW for link prediction.
更多查看译文
关键词
Graph neural networks,knowledge graph embedding (KGE),link prediction,random walk
AI 理解论文
溯源树
样例
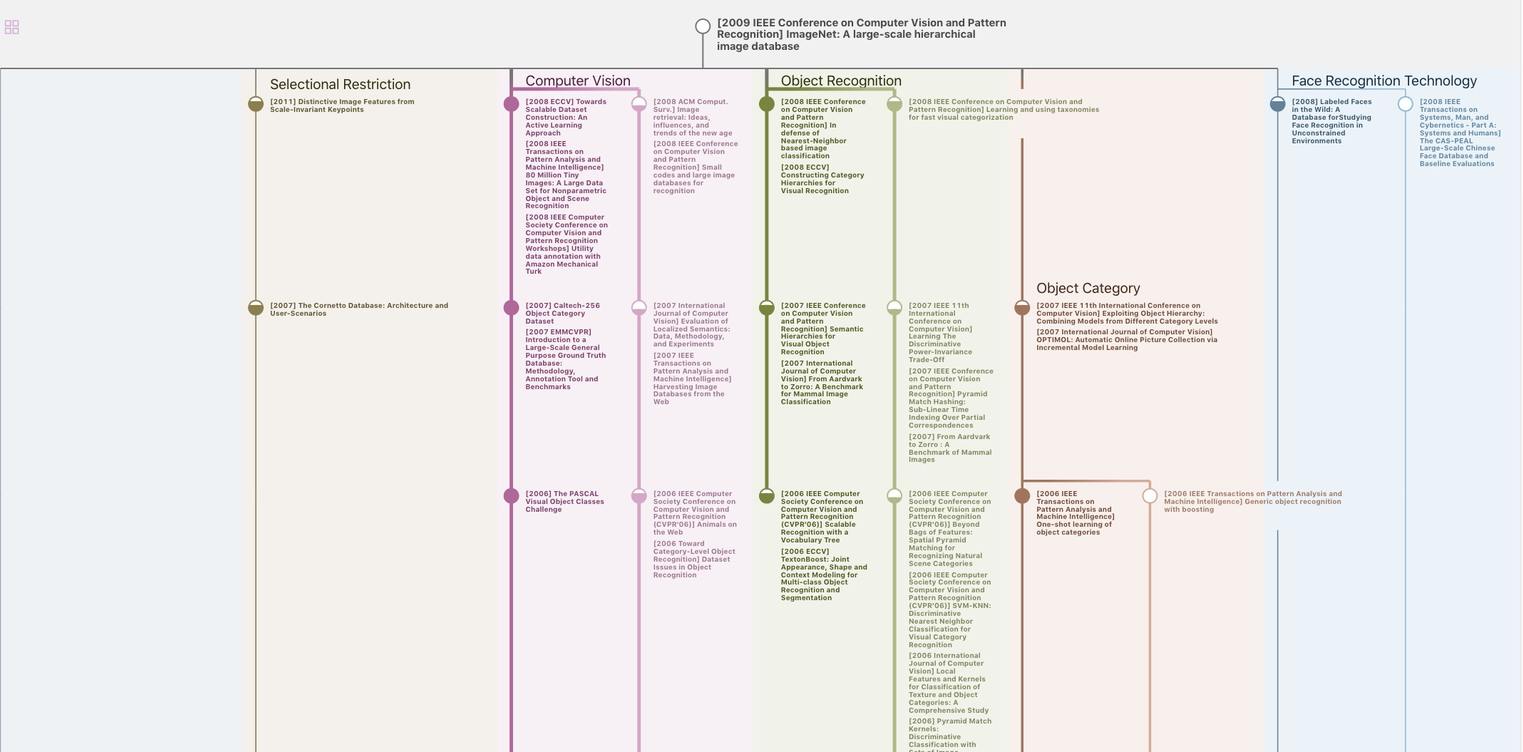
生成溯源树,研究论文发展脉络
Chat Paper
正在生成论文摘要