Personalized Meta-Federated Learning for IoT-Enabled Health Monitoring
IEEE Transactions on Computer-Aided Design of Integrated Circuits and Systems(2024)
摘要
Federated learning (FL) has been widely adopted in IoT-enabled health monitoring on biosignals thanks to its advantages in data privacy preservation. However, the global model trained from FL generally performs unevenly across subjects since biosignal data is inherent with complex temporal dynamics. The morphological characteristics of biosignals with the same label can vary significantly among different subjects (i.e., inter-subject variability) while biosignals with varied temporal patterns can be collected on the same subject (i.e., intra-subject variability). To address the challenges, we present the Personalized Meta-Federated learning (PMFed) framework for personalized IoT-enabled health monitoring. Specifically, in the federated learning stage, a novel momentum-based model aggregating strategy is introduced to aggregate clients’ models based on domain similarity in the meta-federated learning paradigm to obtain a well-generalized global model while speeding up the convergence. In the model personalizing stage, an adaptive model personalization mechanism is devised to adaptively tailor the global model based on the subject-specific biosignal features while preserving the learned cross-subject representations. We develop an IoT-enabled computing framework to evaluate the effectiveness of PMFed over three real-world health monitoring tasks. Experimental results show that the PMFed excels at detection performances in terms of F1 and accuracy by up to 9.4% and 8.7%, and reduces training overhead and throughput by up to 56.3% and 63.4% when compared with the SOTA federated learning algorithms.
更多查看译文
关键词
Federated learning,personal,embedded system
AI 理解论文
溯源树
样例
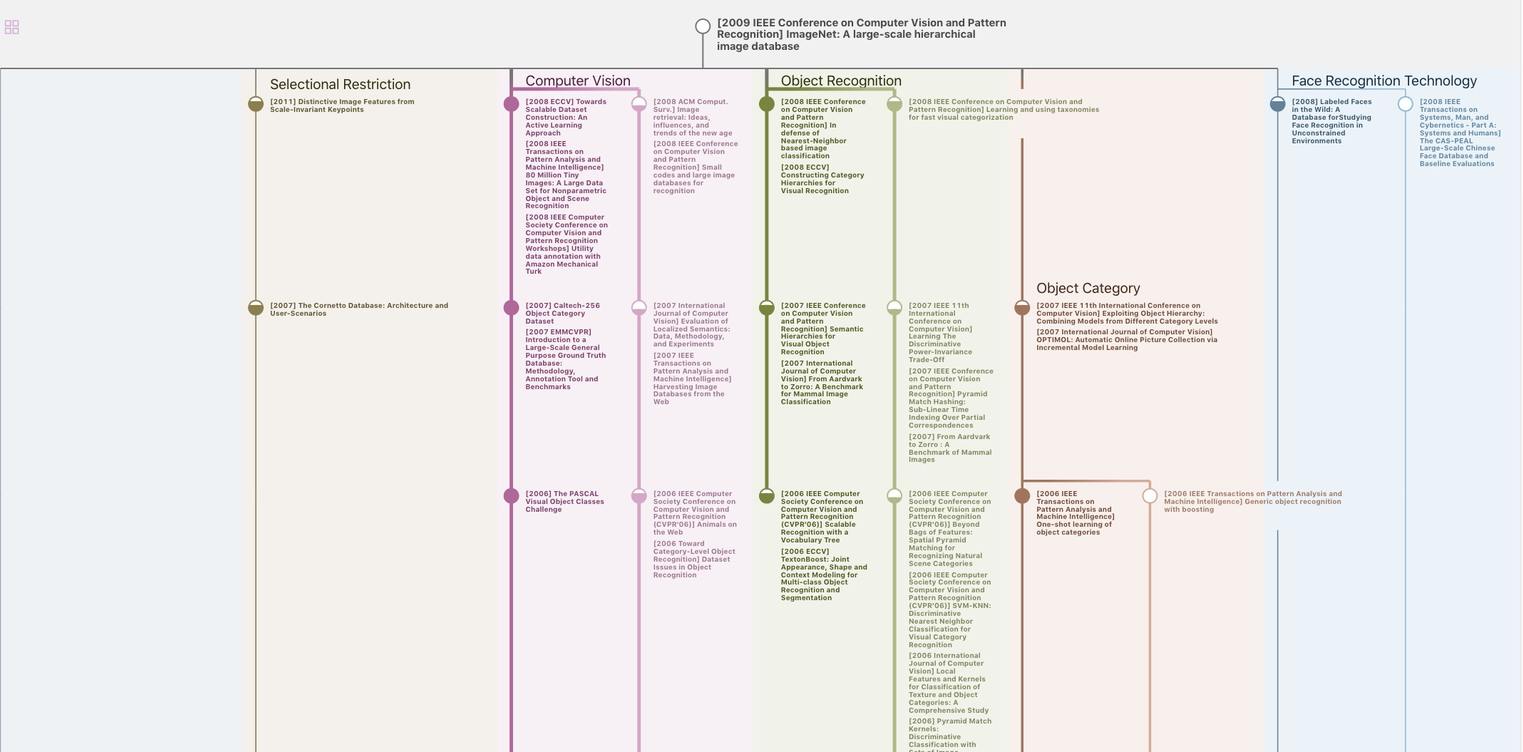
生成溯源树,研究论文发展脉络
Chat Paper
正在生成论文摘要