Privacy-Preserving Heterogeneous Personalized Federated Learning with Knowledge
IEEE Transactions on Network Science and Engineering(2024)
摘要
Personalized federated learning (PFL) has gained increasing attention due to its success in handling the statistical heterogeneity of participants' local data by building distinct local models for participants. However, existing PFL schemes require the identical architecture and size of participants' models, e.g., the same number of layers in convolutional neural networks (CNN). In addition, the growing privacy issues (e.g., local update leakage to the curious server in model aggregation) have not been resolved in PFL. The utilization of identical model architectures among participants reduces the cost of privacy attacks since only one uniform attack method is required to extract private information, exacerbating the privacy threat. This paper proposes a novel privacy-preserving PFL framework that supports heterogeneous model architectures and sizes in delivering personalized models for different participants. Specifically, we utilize participants' knowledge, i.e., the soft predictions of local models on a public dataset, to effectively identify participants with similar data distributions regardless of the specific model architectures used. Based on the participants' knowledge, and their computing and storage capabilities, we employ the affinity propagation (AP) algorithm to implement a multi-level participant clustering mechanism for enabling heterogeneous PFL. Since knowledge is independent of original data, it is considered privacy-preserving during the clustering process. We also devise the ring aggregation algorithm to guarantee participants' privacy during the federated training process. In this way, each participant benefits from other participants with similar data distributions privately and obtains a satisfying personalized model. Furthermore, the cross-cluster knowledge transfer method boosts the personalization performance of weak participants. Sufficient theoretical analyses prove the effectiveness and privacy-preserving capacity of the proposed scheme. Extensive experiments on three benchmark datasets also demonstrate the superiority of our proposed scheme in various settings while maintaining privacy protection, outperforming other state-of-the-art schemes.
更多查看译文
关键词
Federated Learning,Personalization,Knowledge,Heterogeneity
AI 理解论文
溯源树
样例
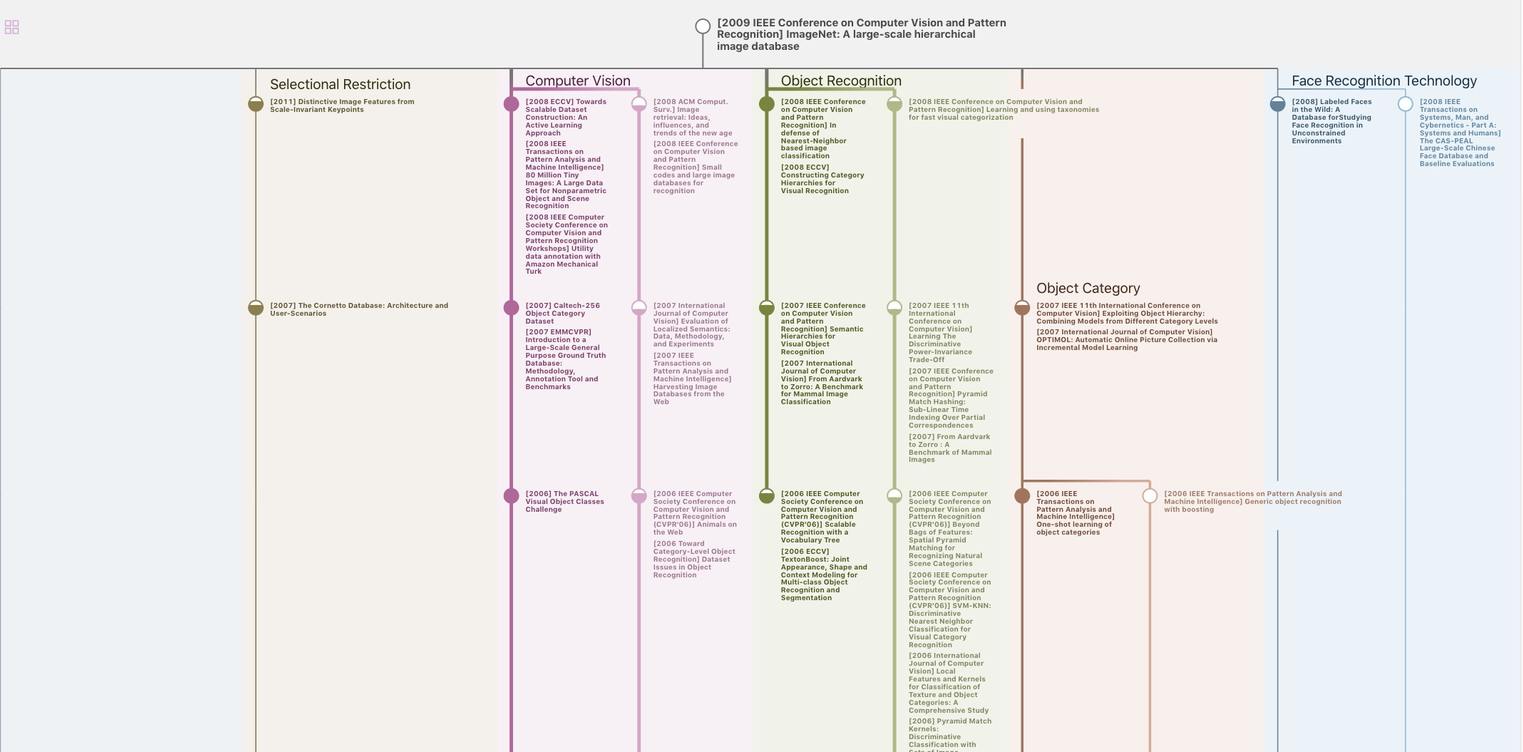
生成溯源树,研究论文发展脉络
Chat Paper
正在生成论文摘要