Accurate Strain Sensing with Fiber-Optic Fabry-Perot Sensors based on CNN-LSTM Model
IEEE Sensors Journal(2024)
摘要
In this work, we proposed a new method of demodulating the spectrum of fiber-optic FP strain sensors by employing convolutional neural networks (CNN) adding long short-term memory (LSTM) networks, hereinafter referred to as CNN-LSTM. The model was built to retrieved the spectra of different strains since the peak tracking algorithm may difficult to accurately find the peaks of spectra and some traditional algorithms have their own limitations, which may cause large errors of demodulation results. The spectra of strain will be normalized firstly and then sent to the model for training, the strain information can be directly extracted without any peak wavelength or cavity length as an intermediary quantity, which simplifies the demodulation process. Experimental results show that the accuracy represented by the coefficient of determination (
R
2
) better than 99.99%, and the root mean square error (RMSE) is about 0.524 με. Additionally, the model has comprehensive advantages over other models in terms of training cost and accuracy. The proposed model demonstrates good performance based on low-resolution spectra, showing its great potential in developing low-cost sensing systems.
更多查看译文
关键词
Fiber-optics FP sensor,CNN-LSTM model,Strain extraction
AI 理解论文
溯源树
样例
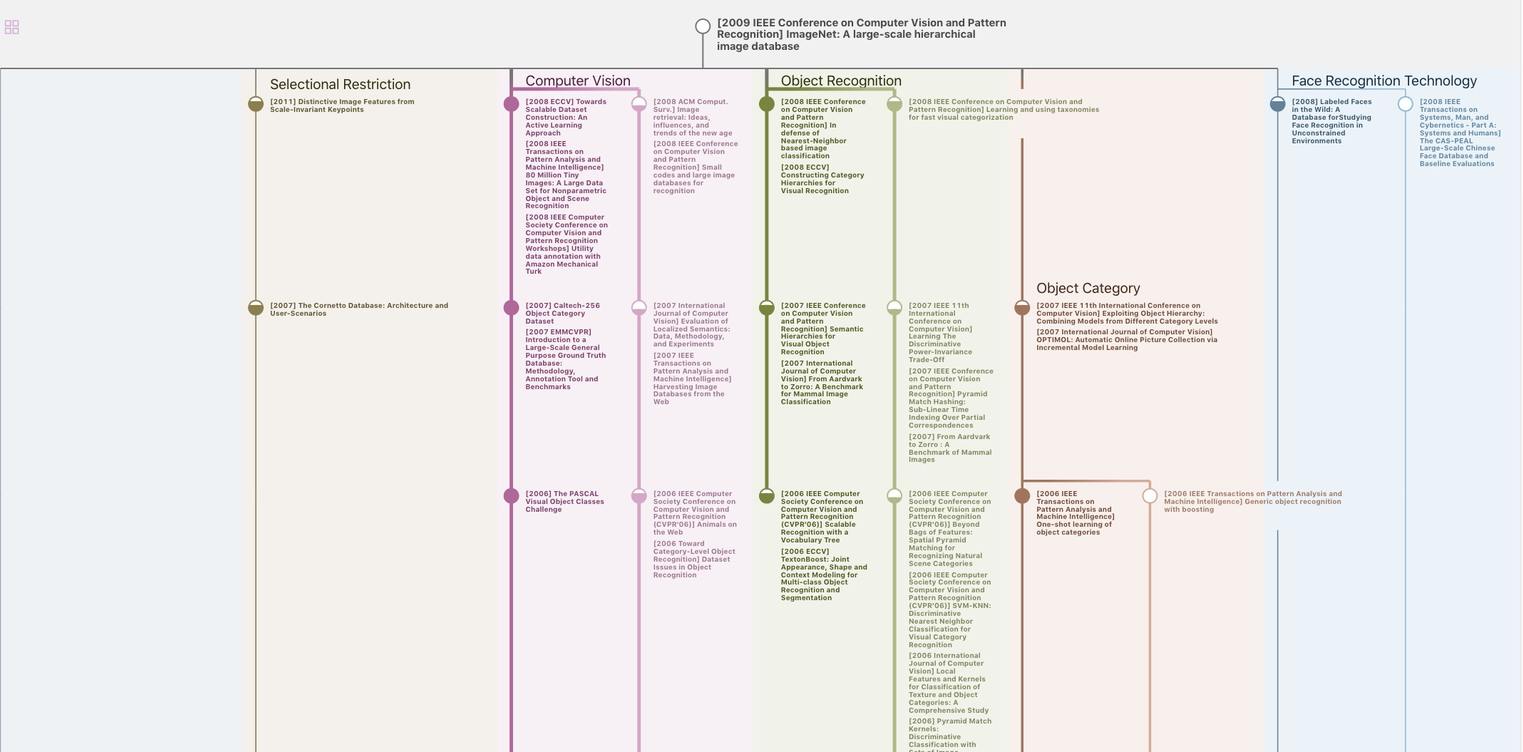
生成溯源树,研究论文发展脉络
Chat Paper
正在生成论文摘要