Discrete mathematical models for enhancing cybersecurity : A mathematical and statistical analysis of machine learning approaches in phishing attack detection
JOURNAL OF DISCRETE MATHEMATICAL SCIENCES & CRYPTOGRAPHY(2024)
摘要
This paper presents a discreate mathematical modelling of cybersecurity phishing attack detection methodologies, emphasizing the crucial role of continual advancements in detection methods amidst the pervasive threat of phishing attacks in the cybersecurity landscape. Leveraging mathematical modeling and machine learning algorithms, the study employs three distinct datasets-Mendeley, URL tokenized, and a merged dataset integrating both. Multiple machine learning algorithms, including Logistic Regression, k-Nearest Neighbors, Support Vector Machines, Random Forest, Gradient Boosting Machines, Neural Networks, CatBoost, and XGBoost, are systematically applied to evaluate their efficacy. In the original Mendeley dataset, XGBoost achieves a top accuracy of 97.24%, along with CatBoost and Random Forest exceeding 97%. Post-preprocessing, CatBoost leads with an accuracy of 97.28%, showcasing superior precision, sensitivity, and F-score. Despite slight accuracy reductions post-preprocessing, models consistently achieve over 94% accuracy on the preprocessed Mendeley dataset, highlighting the substantial impact of preprocessing. Tokenized URLs exhibit comparatively lower performance, with the highest accuracy at 91.95%, emphasizing the challenges associated with this approach. The combined dataset proves optimal for most models, with XGBoost and SVM achieving the highest overall accuracy at 97.68%. SVM excels in sensitivity and specificity, while XGBoost excels in precision. The merged dataset significantly enhances accuracy, sensitivity, specificity, and precision, underscoring its pivotal role in refining predictive capabilities for identifying phishing websites. The results section provides a detailed overview of machine learning model performance on different datasets. CatBoost emerges as a standout performer on the preprocessed Mendeley dataset. The tokenized URLs offer valuable insights into associated challenges, and the combined dataset proves effective for various models. Confusion matrices, ROC curves, and Precision-Recall curves provide nuanced perspectives on model behavior, emphasizing the need for ongoing refinement and investigation into misclassification patterns to enhance model effectiveness in combating phishing threats.
更多查看译文
关键词
Phishing detection,Discrete mathematical modelling,Cybersecurity,Security algorithms,Security analytics
AI 理解论文
溯源树
样例
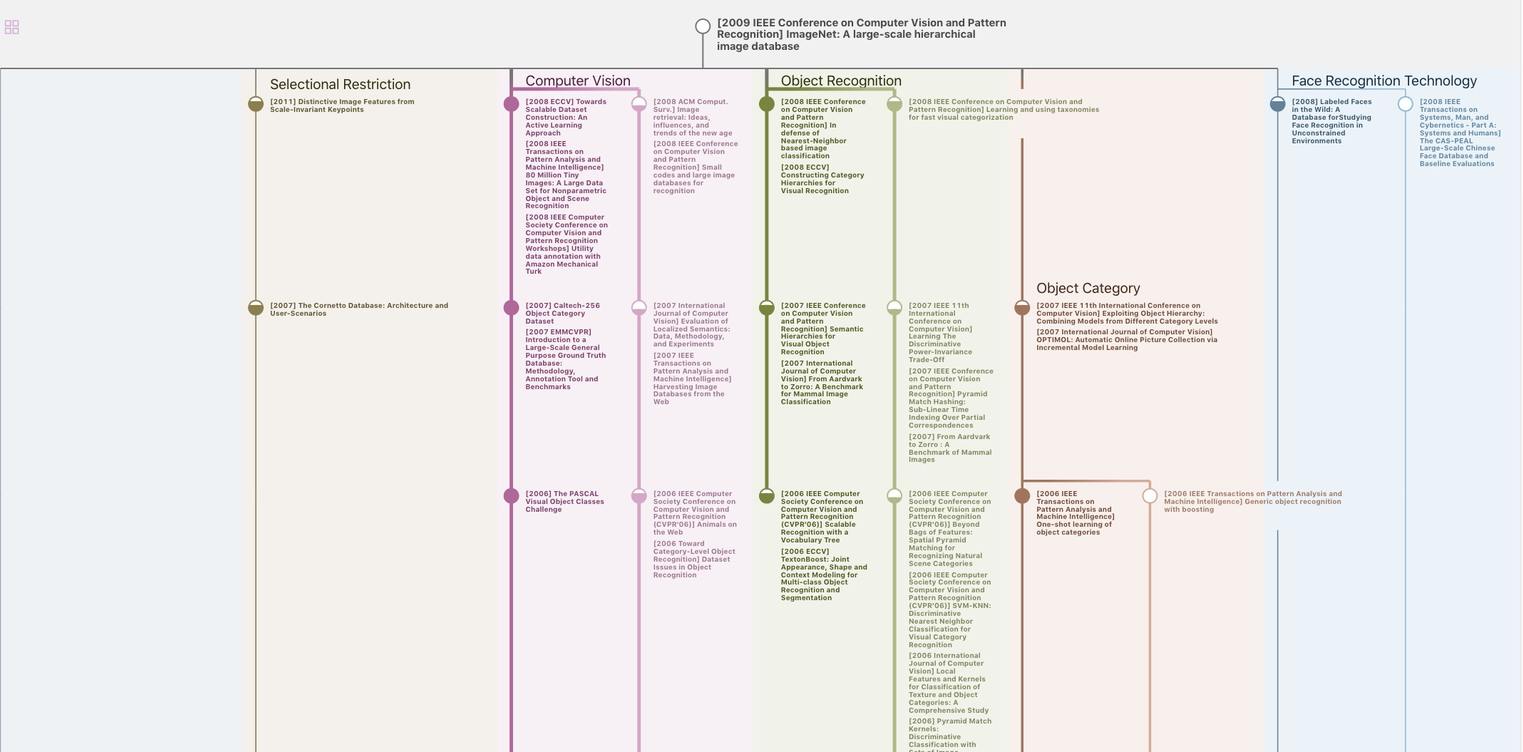
生成溯源树,研究论文发展脉络
Chat Paper
正在生成论文摘要