Multi-view cognition with path search for one-shot part labeling
Computer Vision and Image Understanding(2024)
摘要
The diagram is an abstract form of visual expression in the field of education, which is often used to express complex phenomena and convey logic relationships. In recent years, tasks such as diagram classification and textbook question answering have attracted attention and become a new benchmark for evaluating the complex reasoning ability of models. However, due to the lack of large corpora and the abstract and sparse visual expressions, it is difficult for research methods on natural images to achieve good results on diagrams. In order to solve the above challenges, the researchers consider using the one-shot setting for limited samples challenge and using part labeling to enhance the learning of relational structures. By definition, the one-shot part labeling task is to label multiple parts of an object in the query diagram given only a single support diagram of that category. Under this setting, we propose the Automated Search Multi-view Matching Network (Auto-MMN) which simulating human cognitive methods and process of set-to-set matching problem. We define three views operations based on the attention mechanism and multiplex graph, including the learning of global visual features (global-local view), the interaction between neighboring parts (local-local view), and the comparison of counterparts (cross-local view). We propose a novel learning path search technology to adaptively plan paths for the above three views, which can also increase the generalization performance of the model. We evaluate the Auto-MMN on three different datasets, that is, image-to-image, diagram-to-diagram, and image-to-diagram part labeling scenarios. Extensive experiments show that our model significantly outperforms other baselines on different scenarios and both the multi-view operations and the learning path search produce excellent results. We open source the core code in https://github.com/WayneWong97/Auto-MMN.
更多查看译文
关键词
Diagram understanding,One-shot learning,Part labeling
AI 理解论文
溯源树
样例
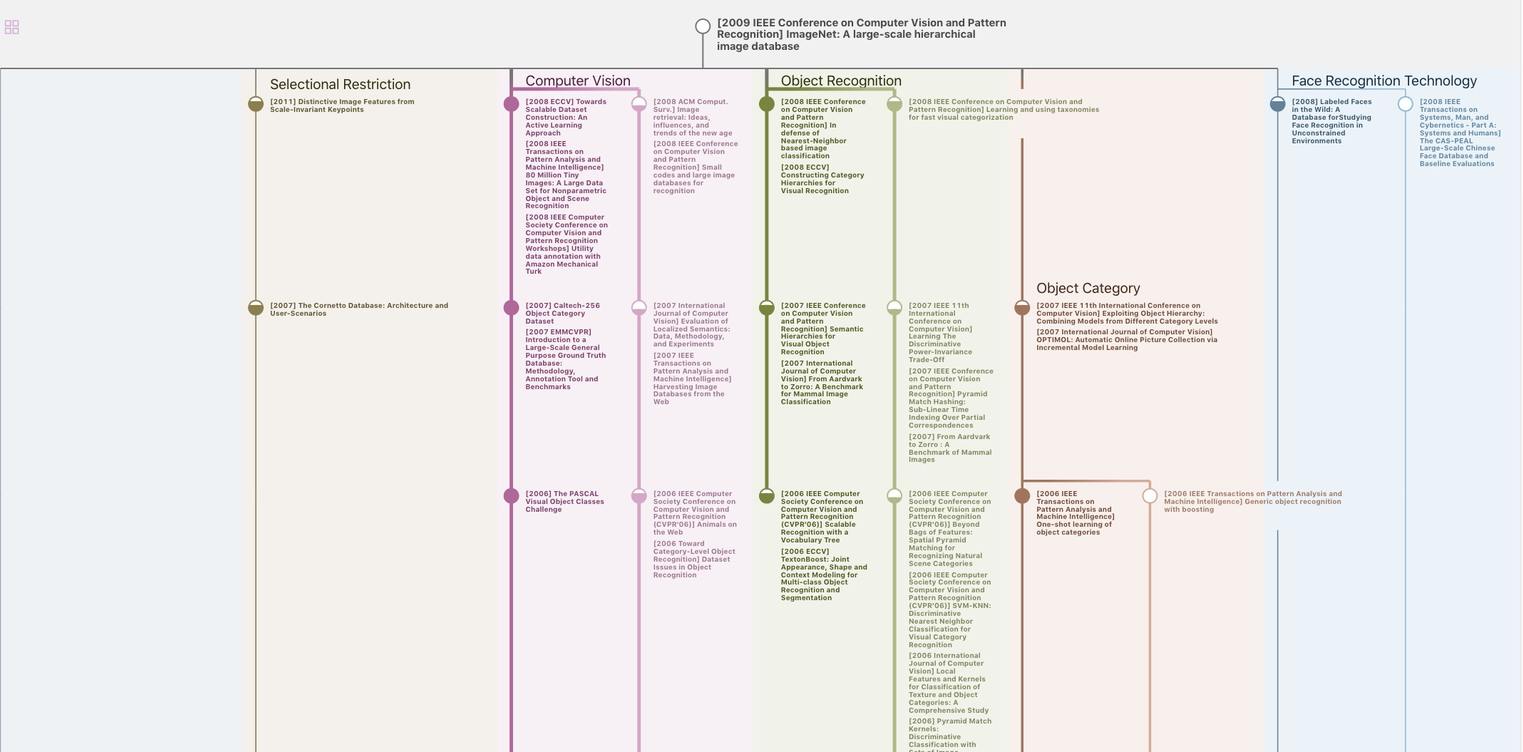
生成溯源树,研究论文发展脉络
Chat Paper
正在生成论文摘要