Privacy-Preserving Intrusion Detection using Convolutional Neural Networks
arxiv(2024)
摘要
Privacy-preserving analytics is designed to protect valuable assets. A common
service provision involves the input data from the client and the model on the
analyst's side. The importance of the privacy preservation is fuelled by legal
obligations and intellectual property concerns. We explore the use case of a
model owner providing an analytic service on customer's private data. No
information about the data shall be revealed to the analyst and no information
about the model shall be leaked to the customer. Current methods involve costs:
accuracy deterioration and computational complexity. The complexity, in turn,
results in a longer processing time, increased requirement on computing
resources, and involves data communication between the client and the server.
In order to deploy such service architecture, we need to evaluate the optimal
setting that fits the constraints. And that is what this paper addresses. In
this work, we enhance an attack detection system based on Convolutional Neural
Networks with privacy-preserving technology based on PriMIA framework that is
initially designed for medical data.
更多查看译文
AI 理解论文
溯源树
样例
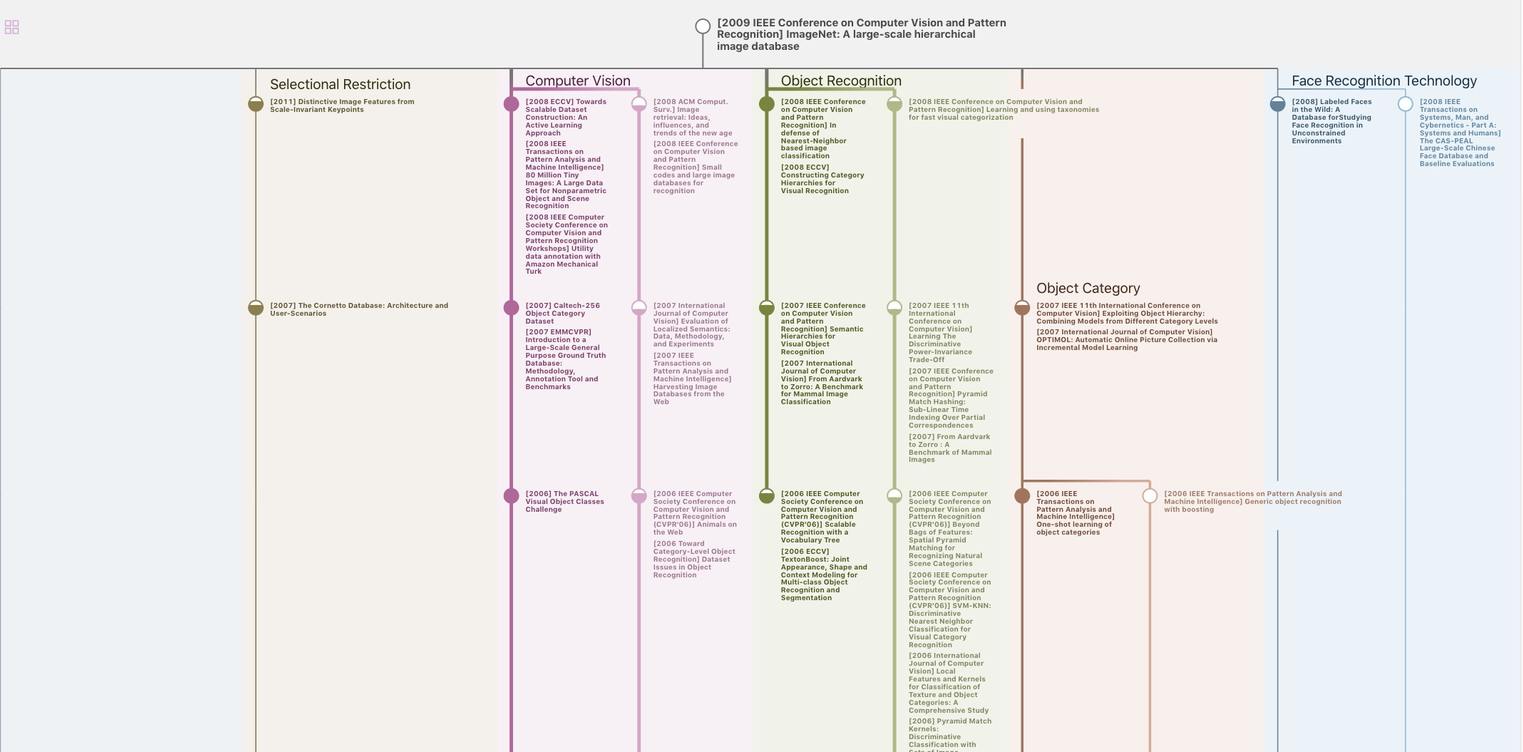
生成溯源树,研究论文发展脉络
Chat Paper
正在生成论文摘要