NGDE: A Niching-Based Gradient-Directed Evolution Algorithm for Nonconvex Optimization.
IEEE transactions on neural networks and learning systems(2024)
摘要
Nonconvex optimization issues are prevalent in machine learning and data science. While gradient-based optimization algorithms can rapidly converge and are dimension-independent, they may, unfortunately, fall into local optimal solutions or saddle points. In contrast, evolutionary algorithms (EAs) gradually adapt the population of solutions to explore global optimal solutions. However, this approach requires substantial computational resources to perform numerous fitness function evaluations, which poses challenges for high-dimensional optimization in particular. This study introduces a novel nonconvex optimization algorithm, the niching-based gradient-directed evolution (NGDE) algorithm, designed specifically for high-dimensional nonconvex optimization. The NGDE algorithm generates potential solutions and divides them into multiple niches to explore distinct areas within the feasible region. Subsequently, each individual creates candidate offspring using the gradient-directed mutation operator we designed. The convergence properties of the NGDE algorithm are investigated in two scenarios: accessing the full gradient and approximating the gradient with mini-batch samples. The experimental studies demonstrate the superior performance of the NGDE algorithm in minimizing multimodal optimization functions. Additionally, when applied to train the neural networks of LeNet-5, NGDE shows significantly improved classification accuracy, especially in smaller training sizes.
更多查看译文
关键词
Evolution algorithm (EA),gradient descent (GD) algorithm,neural networks,nonconvex optimization
AI 理解论文
溯源树
样例
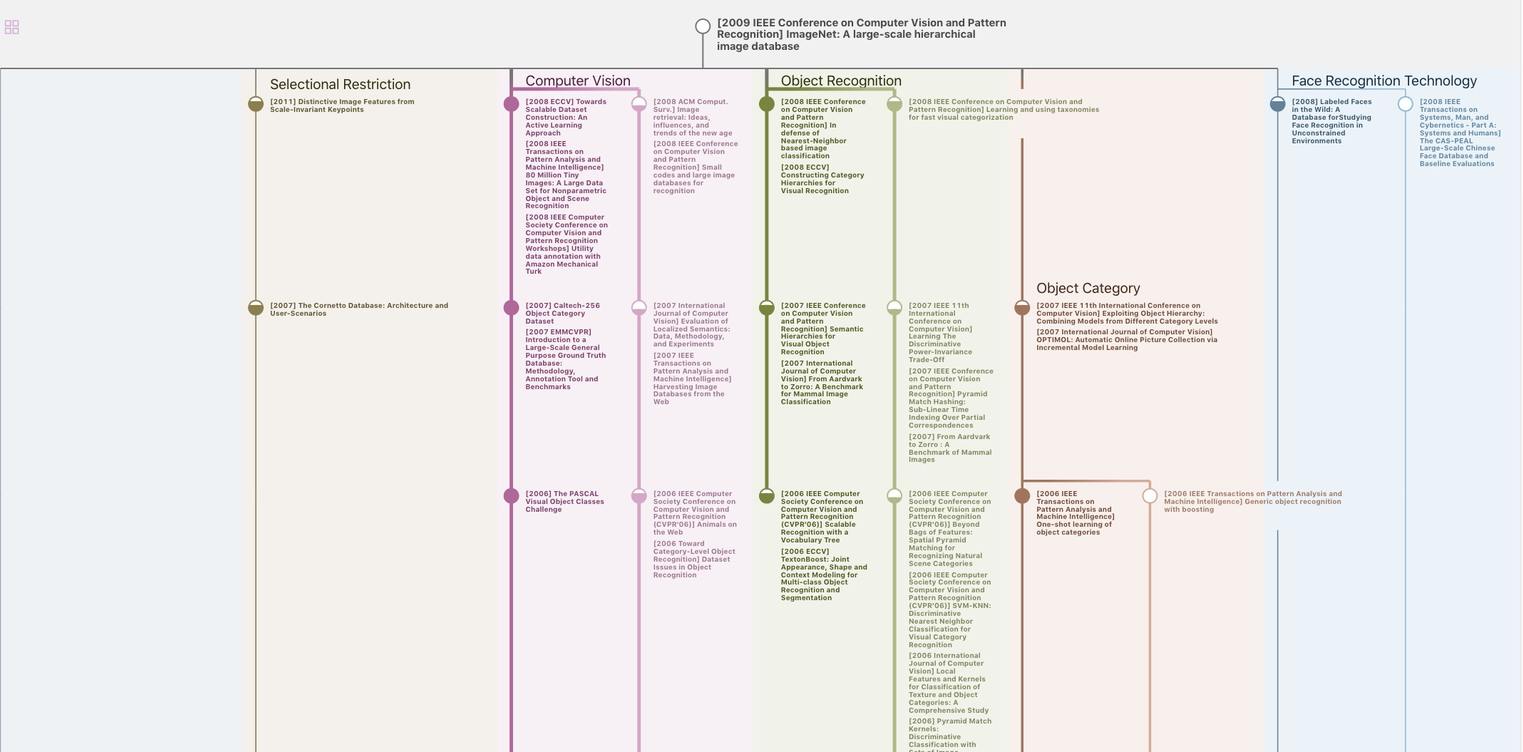
生成溯源树,研究论文发展脉络
Chat Paper
正在生成论文摘要