Vision transformers are active learners for image copy detection
Neurocomputing(2024)
摘要
Image Copy Detection (ICD) is developed to identify and track duplicated or manipulated images. The majority of existing methods rely on Convolutional Neural Networks (CNNs) and are trained using unsupervised learning techniques, which leads to subpar performance. We discover that by carefully designing the training process, Vision Transformer (ViT) backbones yield superior results. Specifically, directly training a ViT for ICD often leads to overfitting on the training images, which in turn results in poor generalization to unseen (test) images. Consequently, we initially train a CNN (such as ResNet-50), and during the ViT training, the distances between the features of CNN and ViT are regularized. We also incorporate an active learning method to further enhance performance. Notably, due to the visual discrepancy between auto-generated transformations and those used in the query set, we incorporate a small number (approximately 0.5% of unlabeled training images) of manually produced and labeled positive pairs. Training models on these pairs results in a significant performance boost though with little cost. Experimental findings demonstrate the effectiveness of our approach, and our method achieves state-of-the-art performance. Our code is available at: https://github.com/WangWenhao0716/ViT4ICD.
更多查看译文
关键词
Image copy detection,Vision transformer,Active learning
AI 理解论文
溯源树
样例
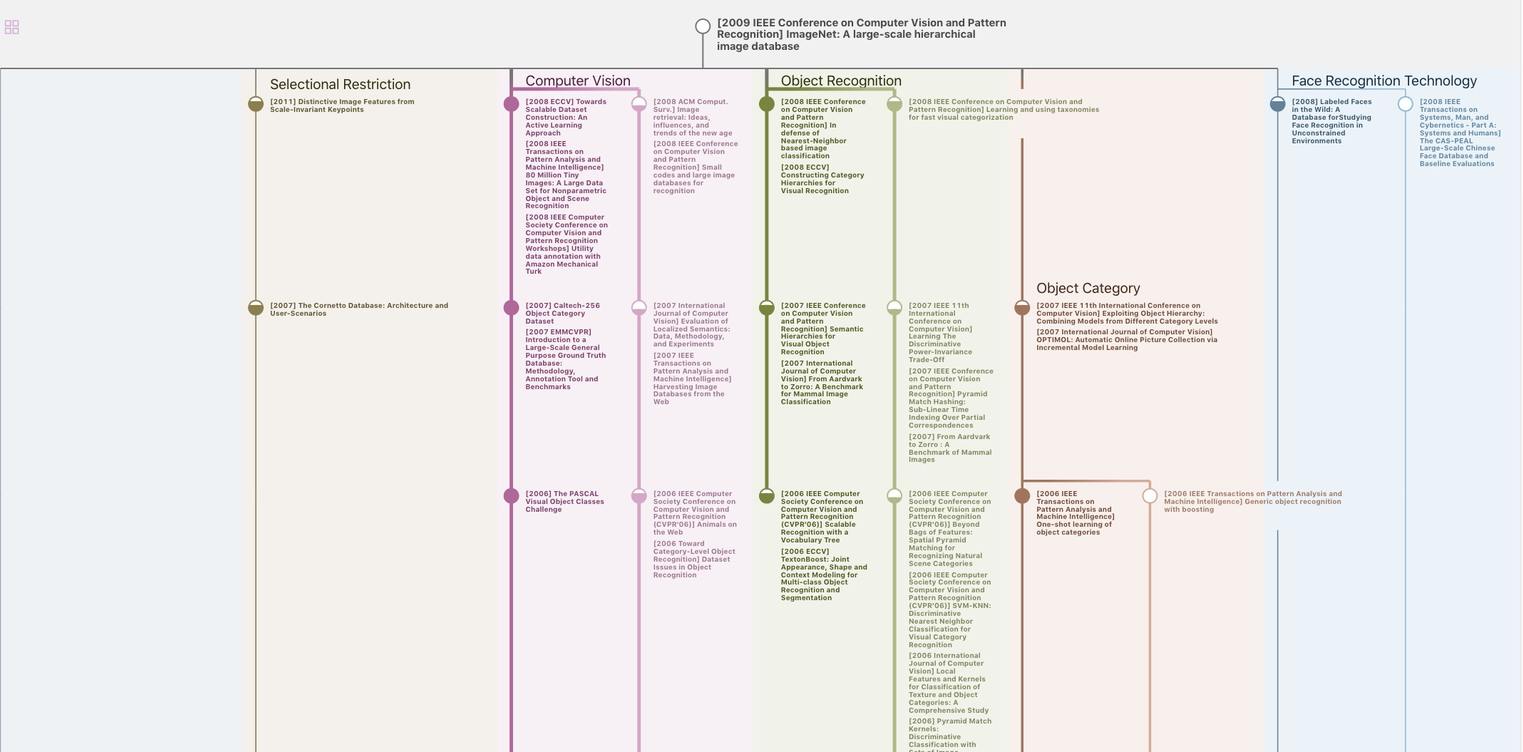
生成溯源树,研究论文发展脉络
Chat Paper
正在生成论文摘要