A Centrality Metrics Approach to Analyzing Temporal Spread Patterns of Misinformation in Social Networks
crossref(2024)
摘要
Abstract This study examines misinformation in social networks with well-known network science metrics. We utilize an already available dataset to analyze tweets related to COVID-19 vaccinations. The methodology we use in this study includes the creation of separate networks for retweets, replies, and mentions. We utilized various centrality metrics to assess the importance of individual tweets such as centrality metrics that primarily consider the network’s topology and connectivity, taking into account factors such as the number of connections, the importance of connections , information flow, and indirect influences. One of our objectives is to determine the length of time that tweets linked to unreliable sources exist within a network through reactions. The empirical results of our study reveal a noteworthy association: tweets that have longer durations tend to be posted by influential accounts within the network, in contrast to tweets that have shorter durations and demonstrate reduced influence. Our study contributes to the advancement of our understanding of the dynamics of misinformation spread, by our results regarding misinforma-tion persistence within social networks. Our observations show potential for informing approaches designed to address misinformation and disin-formation, especially in the setting of public health emergencies like as the current COVID-19 outbreak.
更多查看译文
AI 理解论文
溯源树
样例
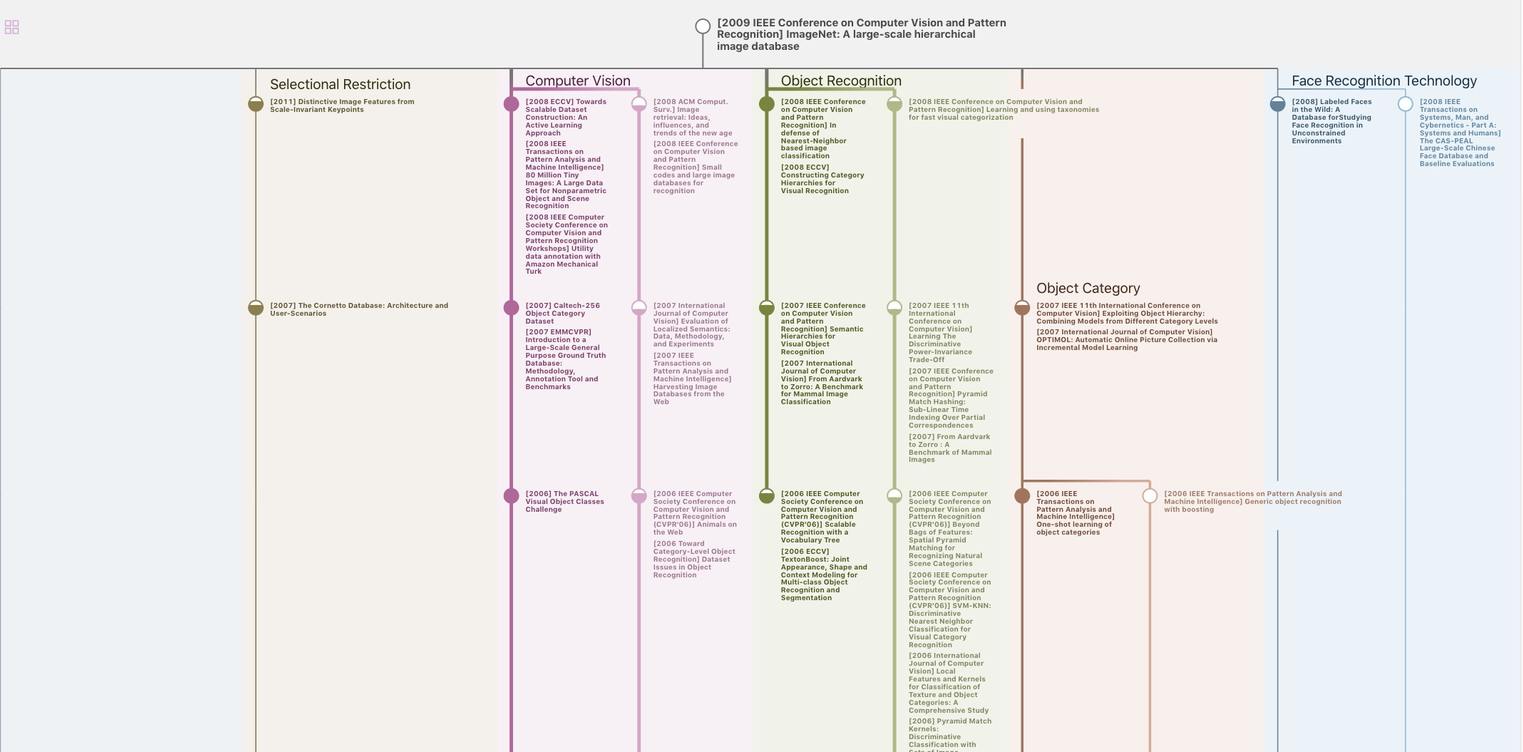
生成溯源树,研究论文发展脉络
Chat Paper
正在生成论文摘要