Generalizable inductive relation prediction with causal subgraph
World Wide Web(2024)
摘要
Inductive relation prediction is an important learning task for knowledge graph reasoning that aims to infer new facts from existing ones. Previous graph neural networks (GNNs) based methods have demonstrated great success in inductive relation prediction by capturing more subgraph information. However, they aggregate all reasoning paths which might introduces redundant information. Such redundant information changes with the context of entity and easily outside the training distribution making existing GNN-base methods suffer from poor generalization. In this work, we propose a novel causal knowledge graph reasoning (CKGR) framework for inductive relation prediction task with better generalization. We first take a causal view of inductive relation prediction and construct a structural causal model (SCM) that reveals the relationship between variables. With our assumption, CKGR extracts causal and shortcut subgraphs conditioned on query triplet. Then, we parameter the backdoor adjustment of causality theory by making intervention in representation space. In this way, CKGR can learn stable causal feature and alleviates the confounding effect of shortcut features that are spuriously correlated to relation prediction. Extensive experiments on various tasks with real-world and synthetic datasets demonstrate the effectiveness of CKGR.
更多查看译文
关键词
Knowledge graph,Knowledge graph reasoning,Inductive relation prediction,Causal inference,Graph neural networks
AI 理解论文
溯源树
样例
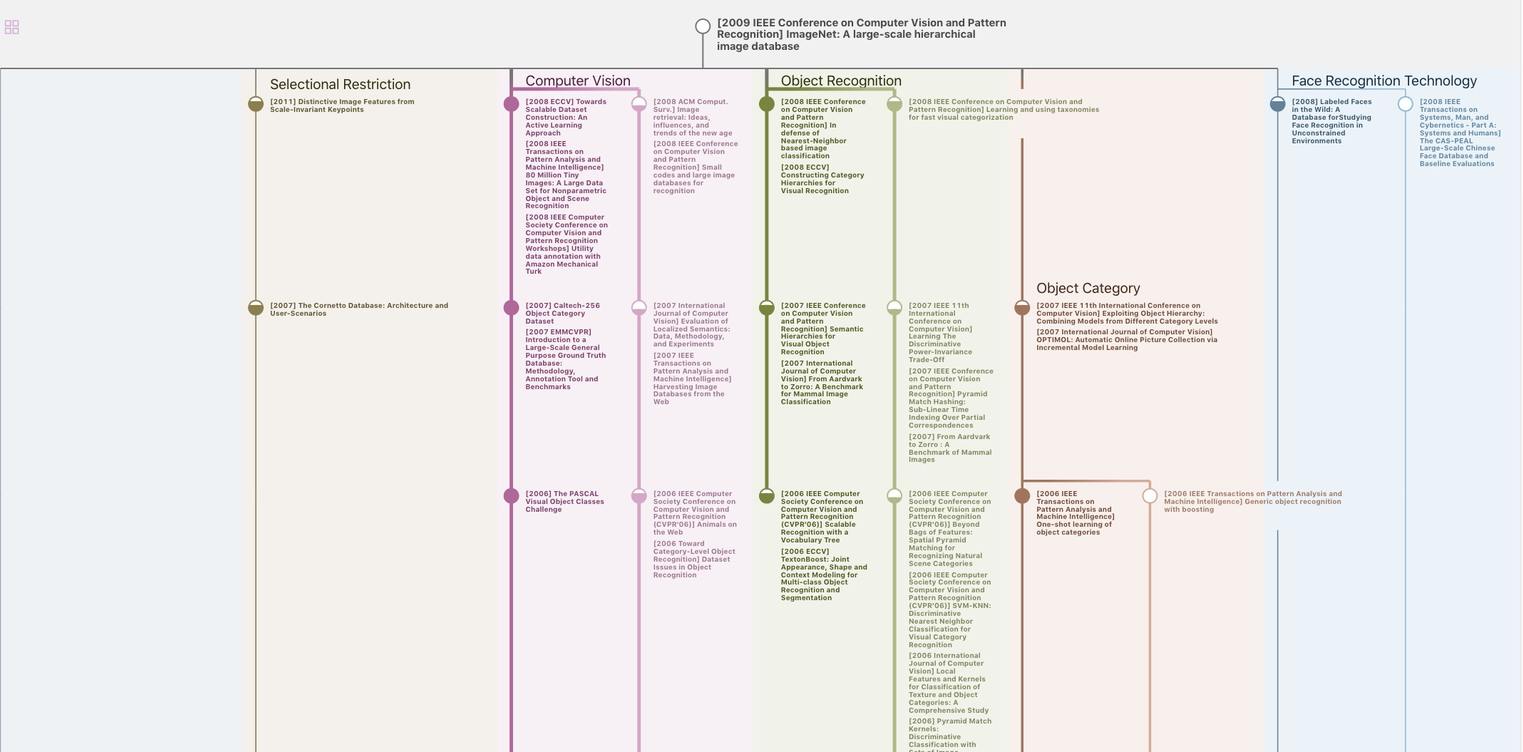
生成溯源树,研究论文发展脉络
Chat Paper
正在生成论文摘要