Geometrically-informed predictive modeling of melt pool depth in laser powder bed fusion using deep MLP-CNN and metadata integration
Journal of Manufacturing Processes(2024)
摘要
The laser powder bed fusion (LPBF) process has the ability to manufacture intricate geometries. However, the fabrication of complex geometries using the LPBF process for industrial applications remains challenging in terms of achieving consistent microstructure and mechanical properties. Experimental investigations show that in addition to process parameters, geometrical factors such as shape and size of parts impact the thermal history, microstructure, defect structure, and thus mechanical and fatigue properties. As a result, the standardization, qualification, and certification of additively manufactured (AM-ed) parts encounters substantial obstacles posed by the obscure impact of design parameters on component quality. In this paper, an artificial intelligence framework is proposed to predict the melt pool depth of the additively manufactured parts considering the geometrical information of the parts. More specifically, a deep Multilayer Perceptron and Convolutional Neural Network (MLP-CNN) framework is designed to predict melt pool depth for any part geometries when printing metallic parts via LPBF using metadata (i.e., numerical and image data). Quite often the melt pool depth generated during the AM processes is employed as a surrogate for thermal, defect- and micro-structural signatures. In melt pool depth prediction of complex geometries in LPBF, a digital twin environment using a finite element model (FEM) is developed and validated using experimental melt pool measurements for different geometries within multi-tracks and layers. The FEM was in agreement with experimentation with an error of less than 15%. Through the process simulation, a large dataset of melt pool depths was obtained for various part geometries and different process parameters. Next, the MLP-CNN framework was established to identify the parallel impact of part design and process parameters on melt pool depths. To enhance the performance and robustness of this model, data augmentation has been implemented by rotating and transferring geometries to artificially expand the dataset. Data augmentation helps to mitigate overfitting and promote better generalization, especially in the context of limited training data. After training, the proposed model is found to give accurate melt pool prediction even for new geometries not considered during training. The fusion of CNN and MLP has led the melt pool predictions for unseen geometries with an accuracy of 95%.
更多查看译文
关键词
LPBF,Finite element modeling,Multi-layer perceptron (MLP),Convolutional neural network (CNN),Various geometries,Melt pool depth,Metadata
AI 理解论文
溯源树
样例
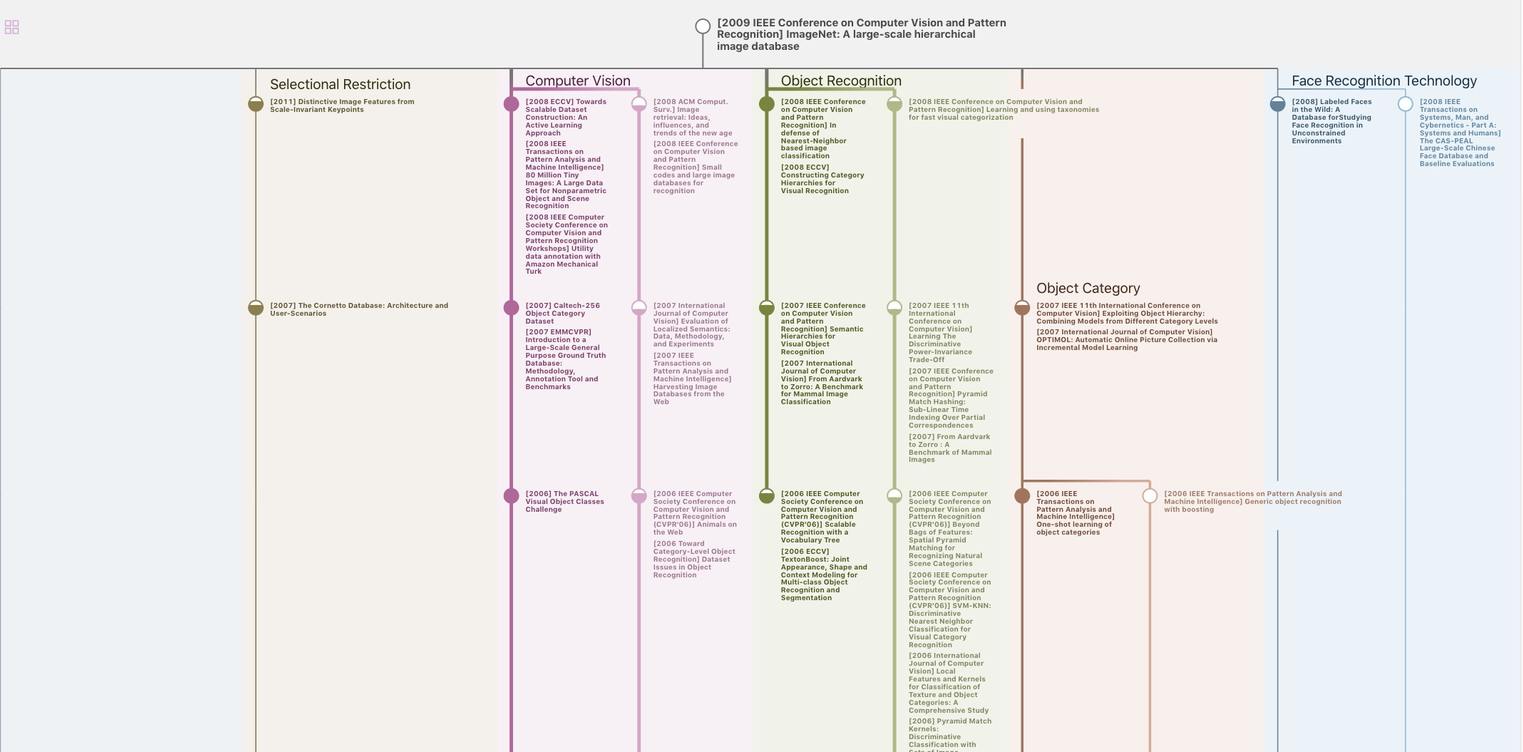
生成溯源树,研究论文发展脉络
Chat Paper
正在生成论文摘要