Partial-tuning Based Mixed-modal Prototypes for Few-shot Classification
IEEE Transactions on Multimedia(2024)
Abstract
The significant success of machine learning models is mainly based on a large amount of data for training iterations, but this limits their generalization for few-shot data. Some existing models utilize the extensive visual and textual modal knowledge of vision-language pre-trained models (VLPs) to compensate for the data scarcity problem. However, they may suffer from a classification bias problem during the fusion of multi-modal information since that they focus on the inter-modal matching while neglecting intra-modal recognition for few-shot images. In this paper, we propose a novel few-shot model with mixed-modal prototypes by partial-tuning the VLPs for better information fusion. It aims to yield a high-quality class prototype representation by integrating the abundant multi-modal knowledge of VLPs and the specific-task information of low-shot visual data. Specifically, we introduce an image-text alignment module to ensure the consistency of the few-shot visual representation and the textual knowledge of VLPs at the feature space. A self-similar learning module is designed to excavate the local and detailed characters of specific class, which is crucial under the data scarcity. Additionally, to preserve the generalizable pre-trained knowledge in the maximum extent, we partial-tune the parameters of VLPs to adapt for the few-shot tasks. To sum up, we mix multi-modal information at the feature representation level instead of fusing multi-modal matching similarities, which effectively mitigates classification bias and ultimately enhances the model performance for few-shot data. The extensive experiments are conducted to evaluate the effectiveness of our model on 11 benchmark datasets and the results show its promising.
MoreTranslated text
Key words
Few-shot learning,multi-modal learning,partial-tuning,and vision-language pre-trained models
AI Read Science
Must-Reading Tree
Example
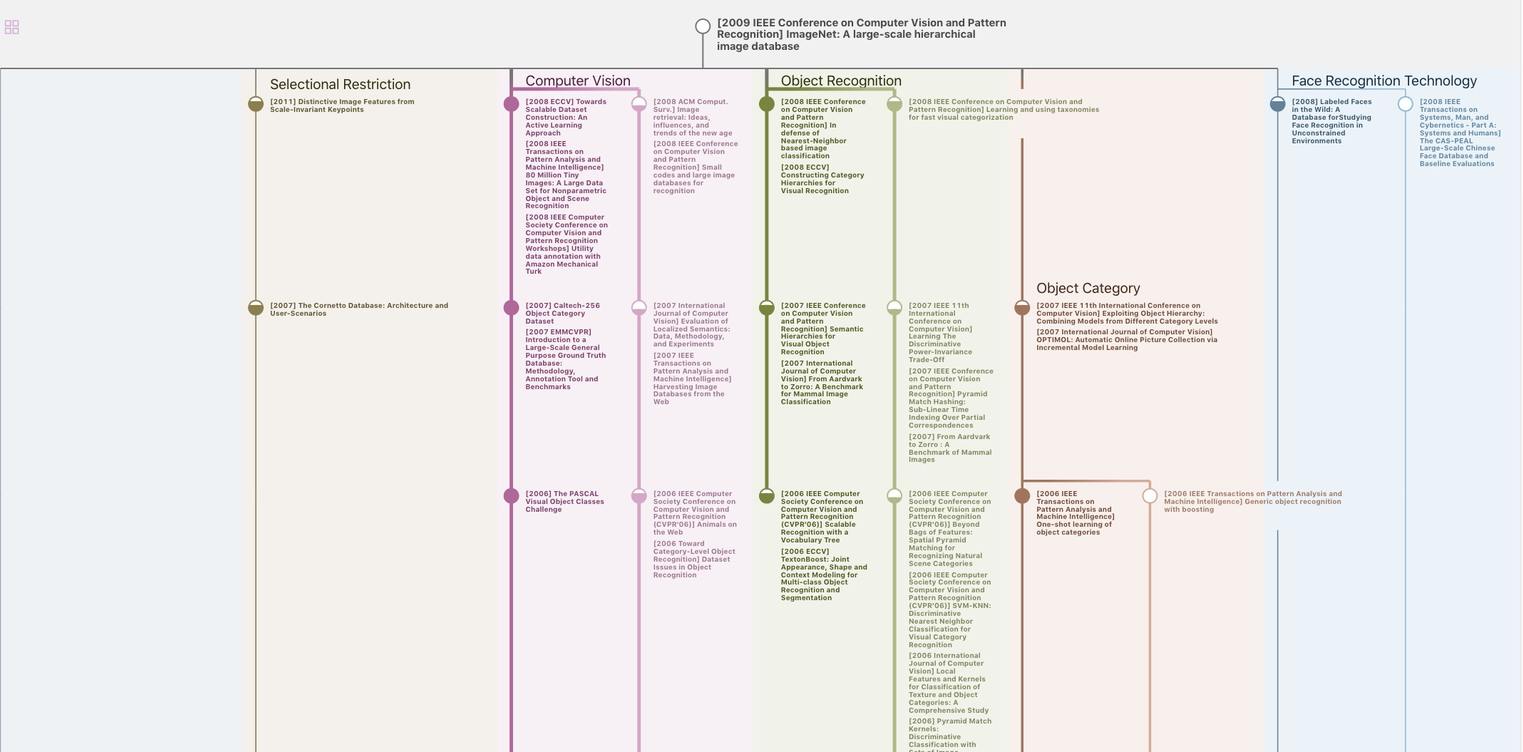
Generate MRT to find the research sequence of this paper
Chat Paper
Summary is being generated by the instructions you defined