Link load balancing scheme for elephant flow in SDN data center.
Parallel and Distributed Processing with Applications(2023)
摘要
In recent years, the rapid emergence of Internet industries such as big data and cloud computing has led to significant growth in data centers. The integration of Software Defined Network(SDN) technology with data centers empowers the controller to manage the network globally and regulate traffic forwarding. This seamless integration significantly enhances the overall operational efficiency of data centers. Nevertheless, as data center traffic surges, a substantial volume of traffic floods the links, potentially leading to load imbalances and severe link congestion under traditional traffic scheduling modes. The concept of “mice flow and elephant flow” introduces a novel idea to enhance load balancing in data centers. In this paper, we propose a novel data center link load balancing method called EDDQN (Elephant Detection Deep Q-learning Network). EDDQN involves a dynamic, two-stage detection process for identifying elephant flow at the edge. Subsequently, the DQN algorithm is utilized to reroute the detected elephant flow, optimizing the load distribution in the data center network. With the advantages of SDN, the link utilization and throughput of data center networks can be improved. Additionally, the packet loss rate and transmission delay can be reduced, thereby improving the network performance.
更多查看译文
关键词
Software Defined Network,Load balancing,Elephant flow,Dynamic periodic sampling,Deep reinforcement learning
AI 理解论文
溯源树
样例
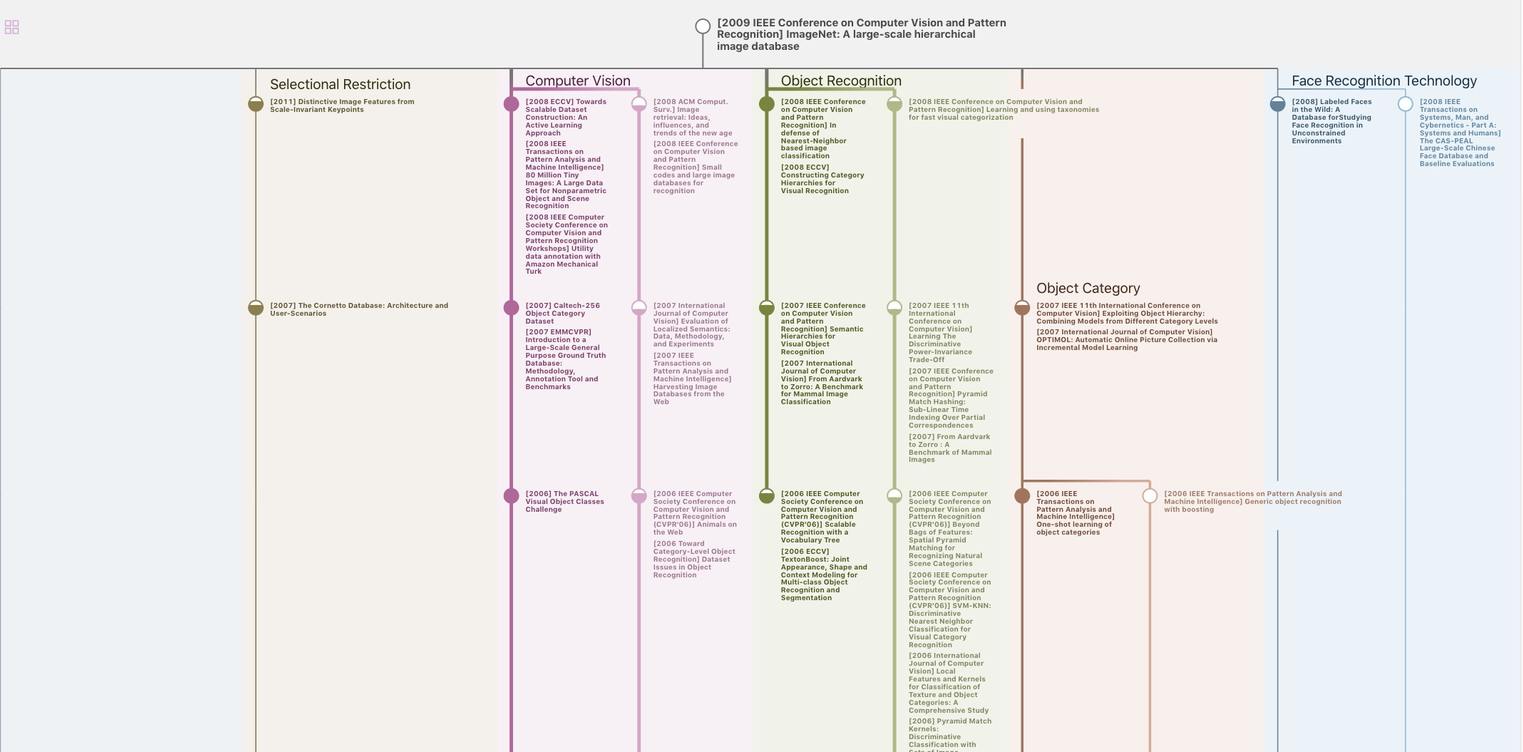
生成溯源树,研究论文发展脉络
Chat Paper
正在生成论文摘要