Controller Synthesis for Autonomous Systems with Deep-Learning Perception Components
IEEE Transactions on Software Engineering(2024)
Abstract
We present DeepDECS, a new method for the synthesis of correct-by-construction software controllers for autonomous systems that use deep neural network (DNN) classifiers for the perception step of their decision-making processes. Despite major advances in deep learning in recent years, providing safety guarantees for these systems remains very challenging. Our controller synthesis method addresses this challenge by integrating DNN verification with the synthesis of verified Markov models. The synthesised models correspond to discrete-event software controllers guaranteed to satisfy the safety, dependability and performance requirements of the autonomous system, and to be Pareto optimal with respect to a set of optimisation objectives. We evaluate the method in simulation by using it to synthesise controllers for mobile-robot collision limitation, and for maintaining driver attentiveness in shared-control autonomous driving.
MoreTranslated text
Key words
discrete-event controller synthesis,Markov model,deep neural network,uncertainty quantification,neuro-symbolic AI
AI Read Science
Must-Reading Tree
Example
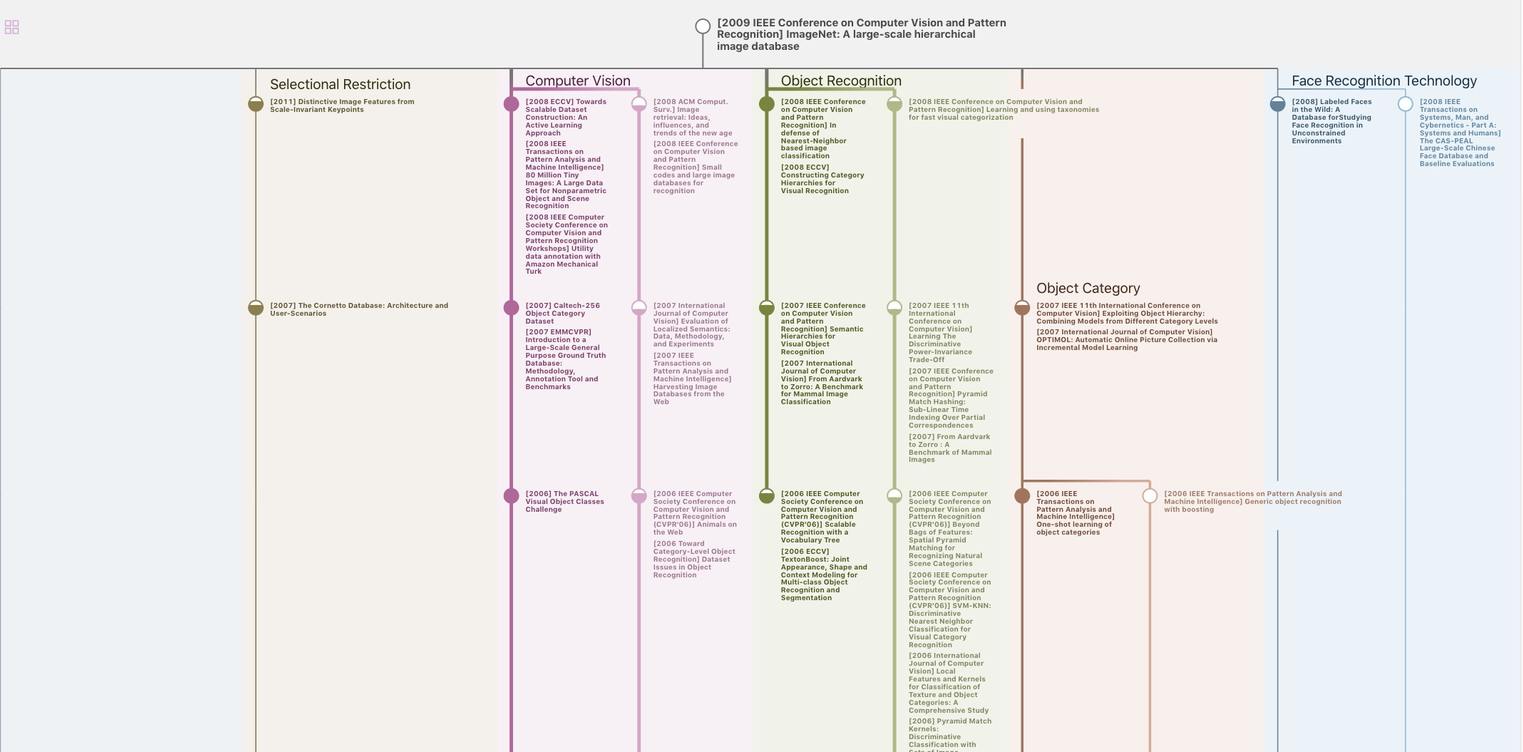
Generate MRT to find the research sequence of this paper
Chat Paper
Summary is being generated by the instructions you defined