CMACF: Transformer-based Cross-Modal Attention Cross-Fusion model for systemic lupus erythematosus diagnosis combining Raman spectroscopy, FTIR spectroscopy, and metabolomics
crossref(2024)
摘要
Diseases contain different amounts of information under different omics perspectives, and multiple omics are complementary. In order to accurately understand the disease mechanism, multimodal signals should be considered. Spectroomics is widely used in medical research as a fast, simple and non-invasive optical analysis technology. It is a new “omics” emerging after genomics, transcriptomics and proteomics. Effectively fusing the information carried by different omics can better predict diseases. In this paper, we design a novel Cross-modal Cross-fusion network (CMACF) based on Transformer self-attention mechanism. Importantly, we observe that the interaction between any two pairwise modalities is different and does not contribute equally to the final disease prediction. Through multiple interaction stages, the features of Raman-infrared spectroscopy, Raman-metabolomics and infrared spectrum-metabolomics were reasonably focused, and the cross-fusion strategy and long sequence decision model were used to obtain the overall correlation of multi-omics features. We conduct extensive experiments on the systemic lupus erythematosus disease multi-omics dataset, and the accuracy and AUC values are 99.44% and 99.98%, respectively, which have the best classification effect. The results show that CMACF has outstanding and stable prediction performance, which is of great reference value for the diagnosis of SLE patients and can explain the contribution of multimodal fusion of multi-omics data.
更多查看译文
AI 理解论文
溯源树
样例
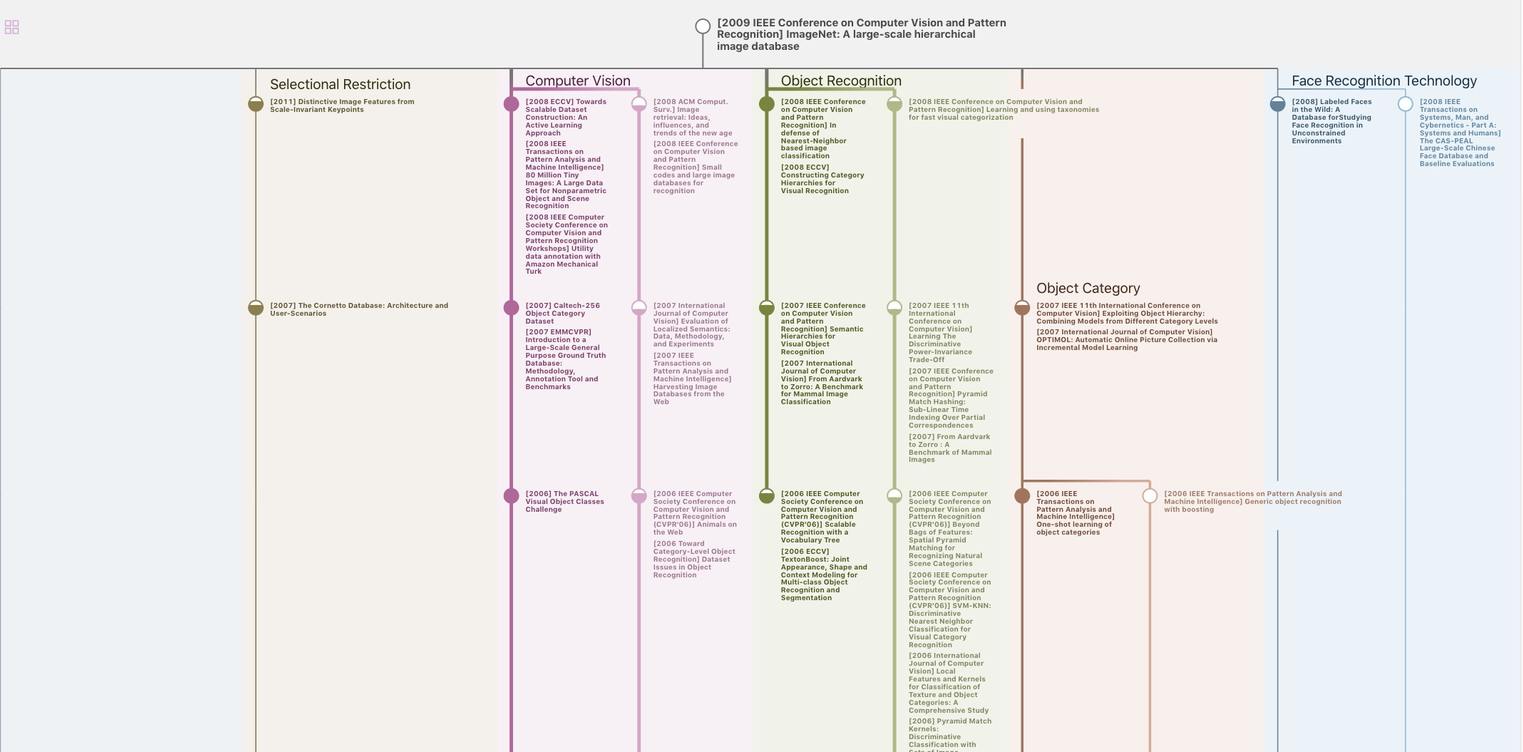
生成溯源树,研究论文发展脉络
Chat Paper
正在生成论文摘要