Temporal Heart Rhythm Clusters and Physiomorphic Age Mapping: A Deep Learning Approach to Cardiovascular Risk Stratification
crossref(2024)
摘要
Purpose Understanding the intricate relationships between sleep quality and cardiovascular outcomes can potentially offer new avenues in risk stratification for cardiovascular diseases (CVD). This study aimed to evaluate the significance of biological age predicted through the analysis of sleep stages and nocturnal heart rhythms as a marker for cardiovascular risk.
Methods We leveraged an unsupervised learning approach to generate time-series clusters utilizing whole-night sleep data from N = 900 patients, focusing on identifying shifts and consistencies in nocturnal heart rhythms that may indicate variations in cardiac health. Following this, a deep learning model was applied to the time-series clusters to estimate the biological age of the individuals, thereby delineating potential relationships between predicted age, biological age, sleep patterns, and heart rhythms.
Results In a distinct test set of 736 individuals, the predicted age based on this experiment showcased a higher association with mortality (Hazard Ratio (HR) 2.27, p < 0.05) and CVD risk (HR 3.56, p < 0.001). Conversely, the age estimated through only nocturnal heart rhythms demonstrated a HR of 2.29 (p < 0.05) for all-cause mortality and 3.13 (p < 0.01) for CVD risk.
Conclusion Our findings underscore the high prognostic potential of sleep and electrocardiography data in predicting cardiovascular risks. The method of utilizing predicted biological age derived from sleep stages and nocturnal heart rhythms stands as a significant metric in risk stratification for CVD. Further research in this area might foster novel strategies for early interventions based on sleep quality and cardiac health markers, potentially saving numerous lives through early detection and intervention.
Author summary This study conducted on a large database of sleep data containing physiological signals such as Electrocardiograms, Sleep Stages, anonymized patient information among others shows that the heart behaviour during sleep is indicative of future cardiovascular (CVD) risk and all-cause mortality. This study employs deep learning to predict biological age which is in turn mapped to CVD risk. Through this study, we can see that while heart rhythms during sleep and different stages of sleep (REM, light sleep, etc) does show an association with CVD risk (this exists in previous literature), the more reliable association is found in heart behaviour during specific sleep stages (which is the novelty of our work). We use deep learning to map ECG into different clusters (n=50) using self-supervised learning, and also to find correlation between these clusters and sleep stages while mapping them to their biological age.
### Competing Interest Statement
The authors have declared no competing interest.
### Funding Statement
Yes
### Author Declarations
I confirm all relevant ethical guidelines have been followed, and any necessary IRB and/or ethics committee approvals have been obtained.
Not Applicable
The details of the IRB/oversight body that provided approval or exemption for the research described are given below:
All ethical guidelines have been adhered.
I confirm that all necessary patient/participant consent has been obtained and the appropriate institutional forms have been archived, and that any patient/participant/sample identifiers included were not known to anyone (e.g., hospital staff, patients or participants themselves) outside the research group so cannot be used to identify individuals.
Not Applicable
I understand that all clinical trials and any other prospective interventional studies must be registered with an ICMJE-approved registry, such as ClinicalTrials.gov. I confirm that any such study reported in the manuscript has been registered and the trial registration ID is provided (note: if posting a prospective study registered retrospectively, please provide a statement in the trial ID field explaining why the study was not registered in advance).
Not Applicable
I have followed all appropriate research reporting guidelines, such as any relevant EQUATOR Network research reporting checklist(s) and other pertinent material, if applicable.
Not Applicable
The SHHS dataset used in this study has been archived by the National Sleep Research Resource with appropriate de-identification. Permissions and access for these datasets were obtained via the online portal: [www.sleepdata.org][1]
* CVD
: Cardiovascular Diseases
ECG
: Electrocardiogram
HR
: Hazard Ratio
AFib
: Atrial Fibrillation
SVT
: Supraventricular Tachycardia
REM
: Rapid Eye Movement
[1]: http://www.sleepdata.org
更多查看译文
AI 理解论文
溯源树
样例
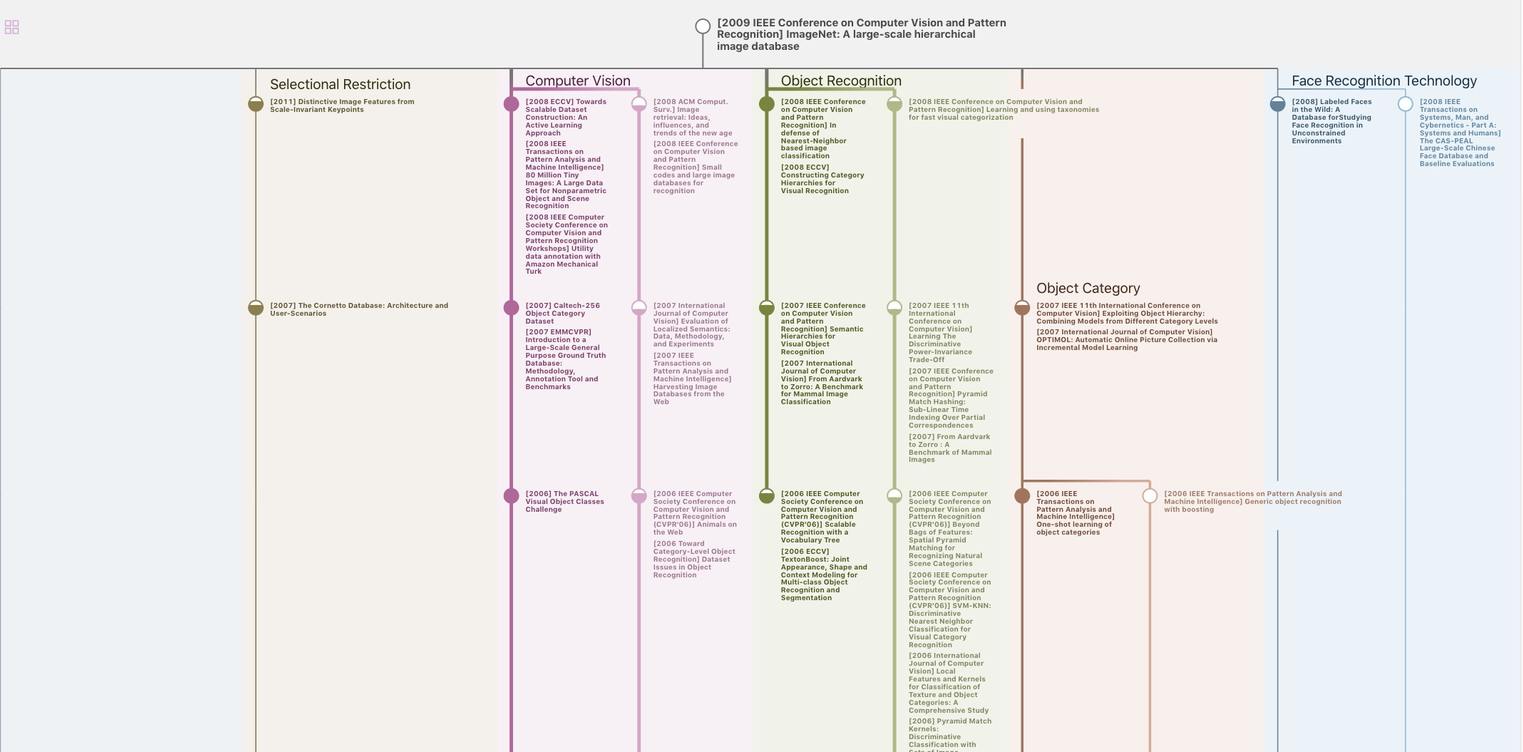
生成溯源树,研究论文发展脉络
Chat Paper
正在生成论文摘要