Evaluating the Impact of Common Clinical Confounders on Performance of Deep-Learning based Sepsis Risk Assessment
crossref(2024)
摘要
Abstract Machine learning based early identification of sepsis in the Emergency department remains a challenging problem, primarily due to the lack of a gold standard for sepsis diagnosis and the corresponding labels required as part of training. In this work, we present a deep learning based predictive model to enable early identification of patients at risk of developing sepsis based on data from the first 24 hours of admission. The predictive model is based on the existing routine blood test results commonly performed on patients CBC (Complete Blood Count), CMP (Comprehensive Metabolic Panel), and Lipid panel, together with vitals, age and sex. To address the challenge of label uncertainty as a part of training process, we explore two different definitions, namely, Sepsis-3 and Adult Sepsis Event and analyze the advantages and drawbacks of each in the context of patient clinical parameters and comorbidities. We particularly analyze the influence of the ground truth label quality on the performance of the overall deep learning system. We see that the model is able to identify patients in the first 24 hours with 83.7% sensitivity and 80% specificity. Our findings underscore the limitations of using individual labels for sepsis and emphasize the superiority of ensemble classifiers based on multiple heterogeneous labels, in the identification of patients with sepsis. The variable performance of the model across different sub-cohorts with specific clinical conditions underscores the need for tailored approaches in sepsis diagnosis, particularly when dealing with patients with confounding comorbidities.
更多查看译文
AI 理解论文
溯源树
样例
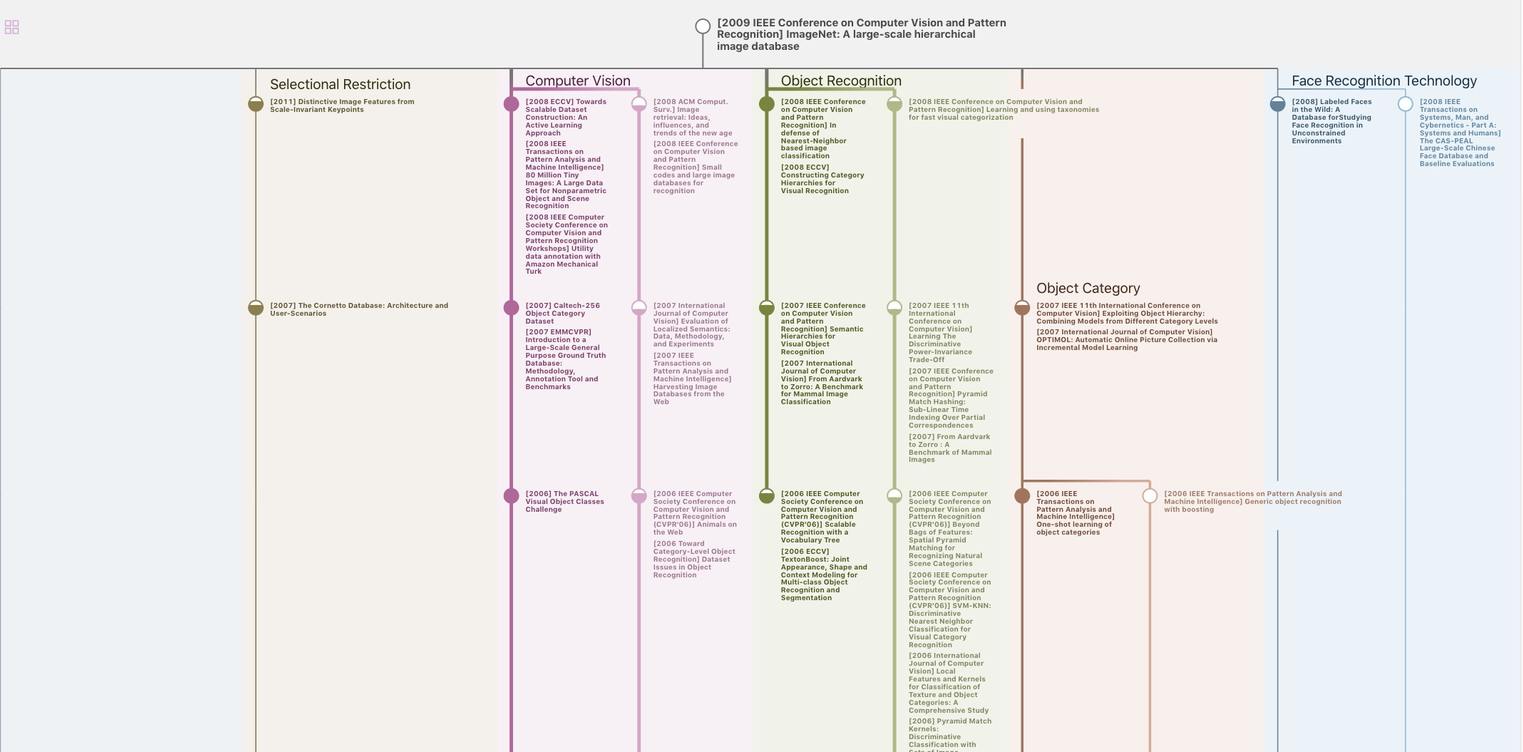
生成溯源树,研究论文发展脉络
Chat Paper
正在生成论文摘要