Causal Discovery from Abundant but Noisy Fuzzy Cognitive Map Set
IEEE Transactions on Fuzzy Systems(2024)
摘要
Fuzzy cognitive maps (FCMs) play a significant role in inferring causal relationships and modeling complex systems. In recent years, numerous FCM-based causal discovery methods have emerged for diverse causal inference scenarios. However, determining the causal relations that best represent the ground truth in a novel scenario remains a challenge, resulting in an abundant but noisy set of candidate causal relations. Addressing this challenge, we introduce a parameter-free model grounded in Bayesian and fuzzy theory to estimate the accurate causal relations of the real system from candidate causal relation datasets. We initially fuzzify the edge weights within candidate FCMs into distinct causal states. Assuming a probabilistic data model linking observed data (causal relations in candidate FCMs) to true causality, we employ Bayesian methods to transform model parameter solving into a maximum posterior probability estimation task. This approach yields an accurate probabilistic data model, facilitating precise estimation of genuine causality from datasets containing multiple candidate relations. This model aims to derive accurate causal relations from datasets populated with multiple candidate relations. Our method validated on 15 diverse causal datasets, constructed from real and synthetic gene regulatory data, exhibits superior accuracy in discerning causal relationships. These results also demonstrate the efficacy of our proposal: 1) stabilizing performance amidst noisy data; 2) resolving algorithmic diversity for precise causal inferences; 3) mitigating fluctuations from hyperparameters. The source code and dataset are available at
https://github.com/IngeTeng/Abundant-but-Noisy-FCMs
.
更多查看译文
关键词
Fuzzy cognitive maps,causal inference,fuzzy set,gene regulatory network,Bayesian theory
AI 理解论文
溯源树
样例
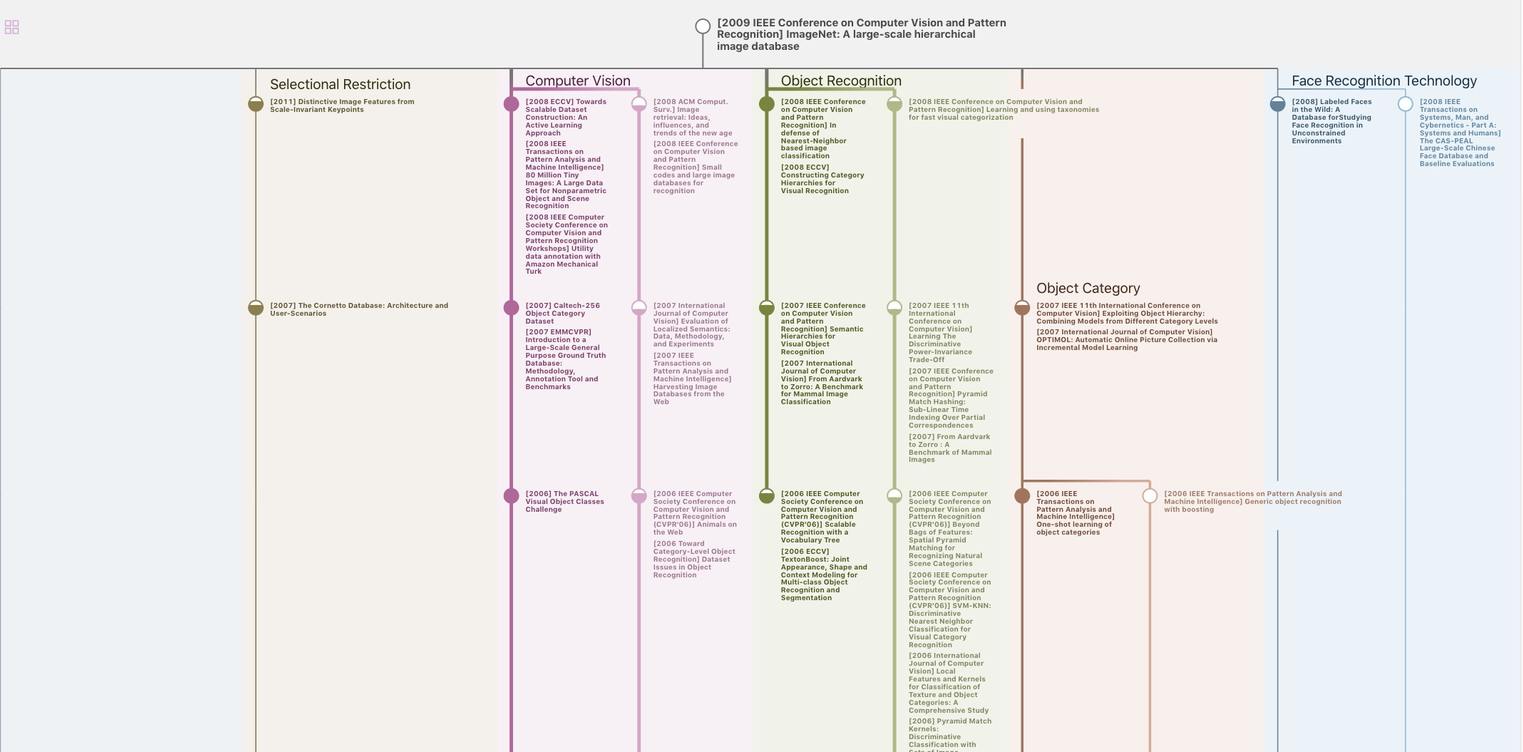
生成溯源树,研究论文发展脉络
Chat Paper
正在生成论文摘要