NeRFEditor: Differentiable Style Decomposition for 3D Scene Editing.
IEEE/CVF Winter Conference on Applications of Computer Vision(2024)
摘要
We present NeRFEditor, an efficient learning framework for 3D scene editing, which takes a video as input and outputs a high-quality, identity-preserving stylized 3D scene. Our goal is to bridge the gap between 2D and 3D editing, catering to a wide array of creative modifications such as reference-guided alterations, text-based prompts, and user interactions. We achieve this by encouraging a pre-trained StyleGAN model and a NeRF model to learn mutually consistent renderings. Specifically, we use NeRF to generate numerous (image, camera pose)-pairs to train an adjustor module, which adapts the StyleGAN latent code for generating high-fidelity stylized images from any given viewing angle. To extrapolate edits to novel views, i.e., those not seen by StyleGAN pre-training, while maintaining 360° consistency, we propose a second self-supervised module that maps these views into the hidden space of StyleGAN. Together these two modules produce sufficient guidance for NeRF to learn consistent stylization effects across the full range of views. Experiments show that NeRFEditor outperforms prior work on benchmark and real-world scenes with better editability, fidelity, and identity preservation.
更多查看译文
关键词
Applications,Arts / games / social media,Algorithms,3D computer vision,Algorithms,Computational photography,image and video synthesis
AI 理解论文
溯源树
样例
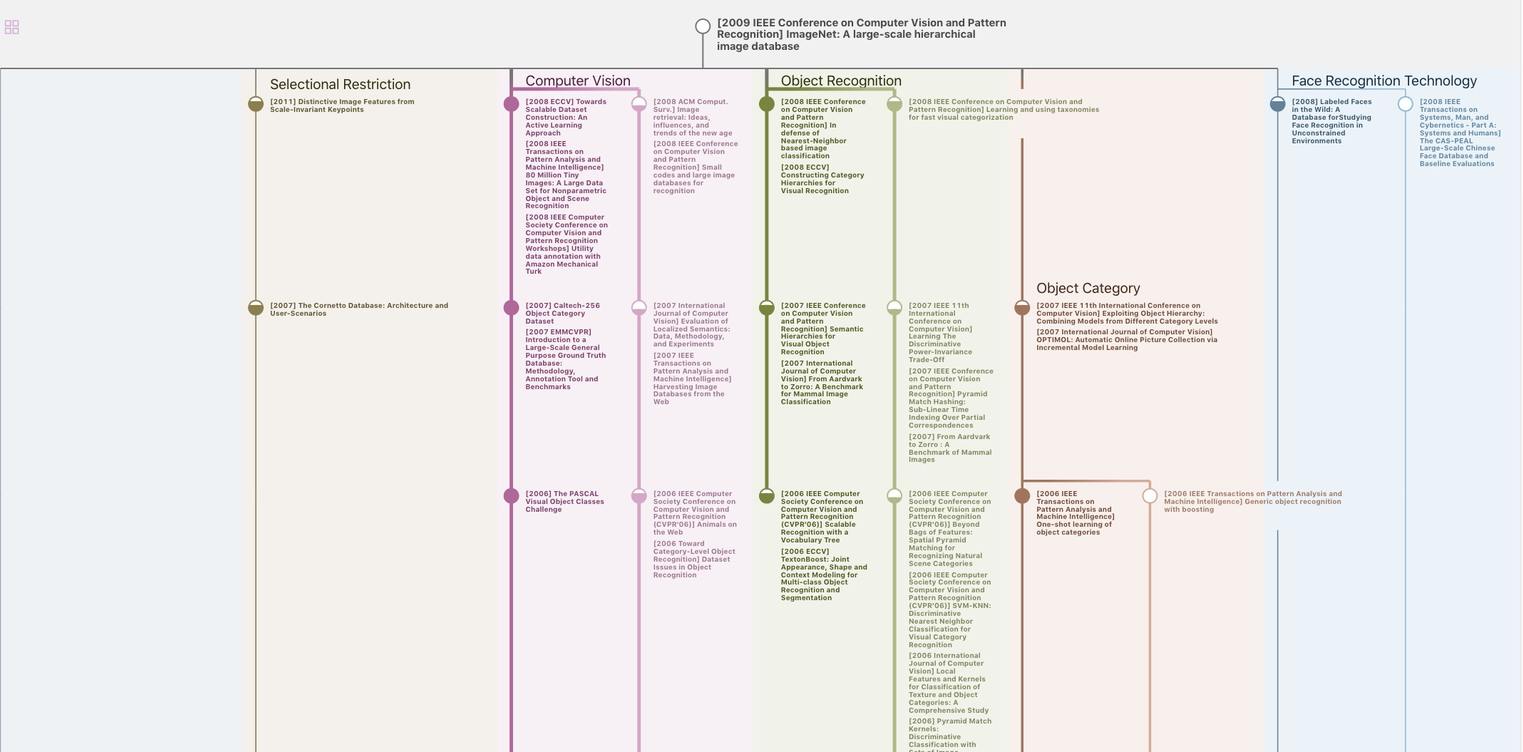
生成溯源树,研究论文发展脉络
Chat Paper
正在生成论文摘要