Few-shot cow identification via meta-learning
Information Processing in Agriculture(2024)
摘要
Cow identification is a prerequisite for precision livestock farming. Biometric-based methods have made significant progress in cow identification. However, substantial labelling costs and frequent identification task changes are still hamper model application. In this work, a novel method called “MFCI” was proposed to achieve accurate cow identification under few-shot and task-changing conditions. Specifically, the proposed method comprises two components: cow location and cow identification. First, an improved YOLOv5n with Ghost module was adopted to quickly detect cow locations in images. Then, the Model-Agnostic Meta-Learning (MAML) framework was introduced for accurate identification under few-shot conditions and for fast adaptation to frequent changes in individual cows. Moreover, an autoencoder was adopted to allow Base-Learner learn more generalized features by combining both supervised and unsupervised approaches. The experimental results showed that the proposed cow location model achieved a mAP of 99.5 %. The proposed cow identification model attained an accuracy of 90.43 % with only five samples per cow for 20 cows, outperforming other state-of-the-art methods. The results demonstrate the broad applicability and significant value of the proposed method.
更多查看译文
关键词
Precision livestock,Cattle identification,Few-shot,Task-driven,Autoencoder
AI 理解论文
溯源树
样例
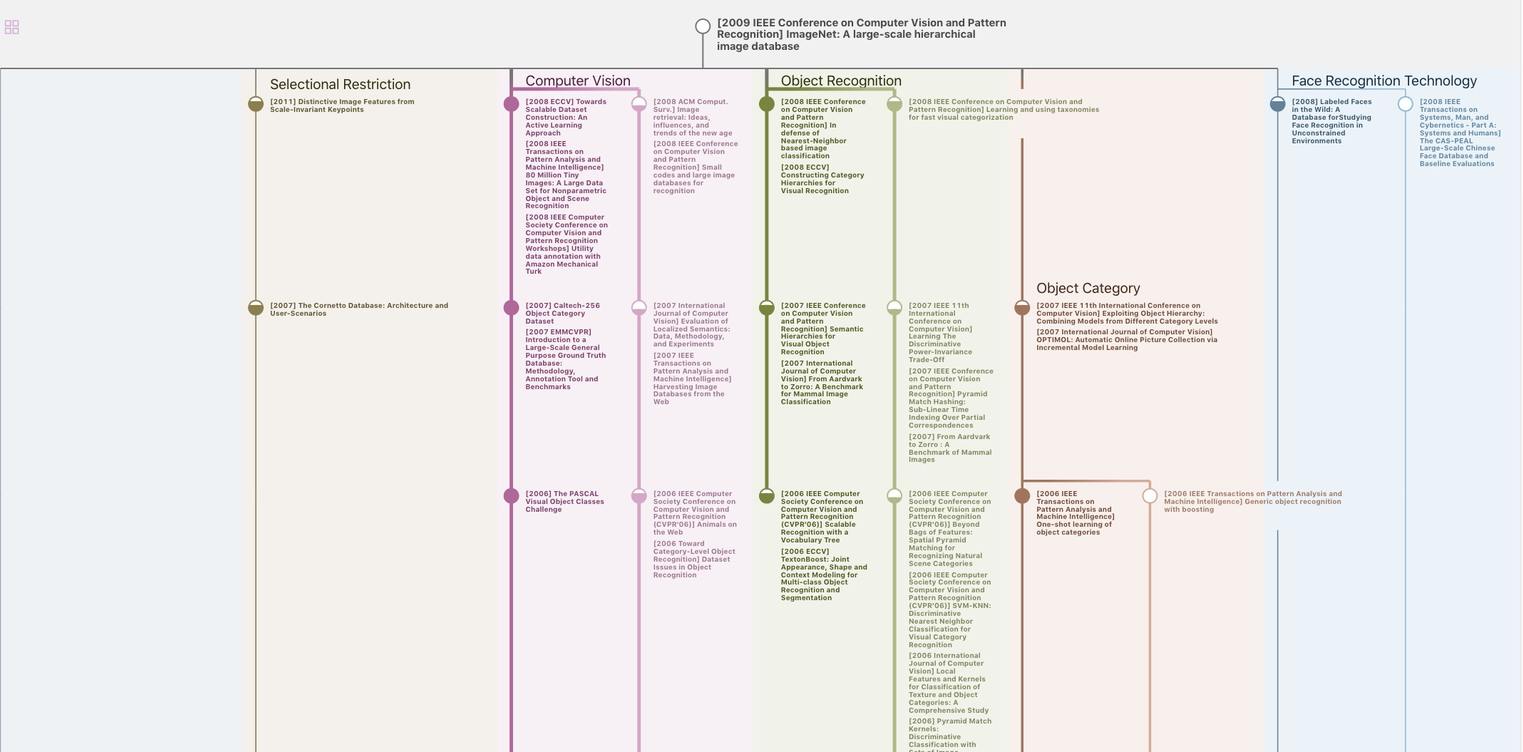
生成溯源树,研究论文发展脉络
Chat Paper
正在生成论文摘要