An Efficient Sparse Neural Network Accelerator for Low-Cost Edge Systems
2023 Congress in Computer Science, Computer Engineering, & Applied Computing (CSCE)(2023)
摘要
It is difficult to achieve real-time performance for a deep neural networks (DNN) in low-profile systems such as edge computing or Internet of Things (IoT) due to the large amount of computations that are required. A commonly used activation function is ReLU (Rectified Linear Unit) due to its simplicity and good performance. A key characteristic of the ReLU function is that it produces sparse vectors within the neural network. In this paper, we propose an optimized DNN accelerator for sparse vector multiplications. In particular, we propose a squeezer unit to detect zeros and then skip feeding that data to the processing elements. In addition, we design a dynamic scheduler to efficiently allocate multiple neuron computations to the processing elements. With our architecture, we achieve reduced hardware resources compared to prior work due to a 62% reduction in the number of required index bits. We also achieve 8.6% better processing speed than for a system without the acceleration.
更多查看译文
关键词
Deep neural networks,sparse data multiplication,DNN accelerator,low-cost,IoT
AI 理解论文
溯源树
样例
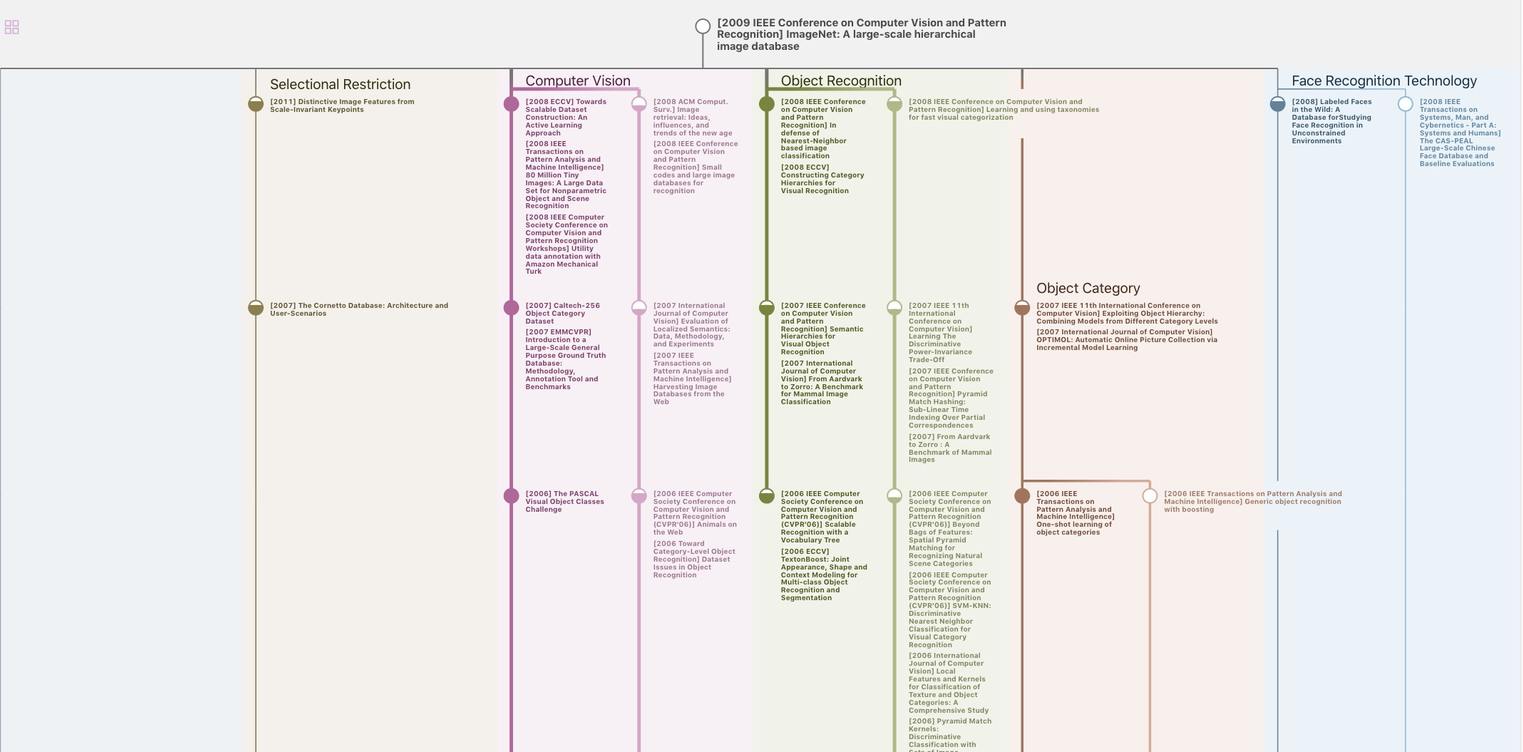
生成溯源树,研究论文发展脉络
Chat Paper
正在生成论文摘要