MarsLS-Net: Martian Landslides Segmentation Network and Benchmark Dataset.
IEEE/CVF Winter Conference on Applications of Computer Vision(2024)
摘要
Martian landslide segmentation is a challenging task compared to the same task on Earth. One of the reasons is that vegetation is typically lost or significantly less compared to its surroundings in the regions of landslide on Earth. In contrast, Mars is a desert planet, and there is no vegetation to aid landslide detection and segmentation. Recent work has demonstrated the strength of vision transformer (ViT) based deep learning models for various computer vision tasks. Inspired by the multi-head attention mechanism in ViT, which can model the global longrange spatial correlation between local regions in the input image, we hypothesize self-attention mechanism can effectively capture pertinent contextual information for the Martian landslide segmentation task. Furthermore, considering parameter efficiency or model size is another important factor for deep learning algorithms, we construct a new feature representation block, namely Progressively Expanded Neuron Attention (PEN-Attention), to extract more relevant features with significantly fewer trainable parameters. Overall, we refer to our deep learning architecture as the Martian landslide segmentation network (MarsLS-Net). In addition to the new architecture, we introduce a new multi-modal Martian landslide segmentation dataset for the first time, which will be made publicly available at https://github.com/MAIN-Lab/Multimodal-Martian-Landslides-Dataset
更多查看译文
关键词
Applications,Remote Sensing,Applications,Environmental monitoring / climate change / ecology
AI 理解论文
溯源树
样例
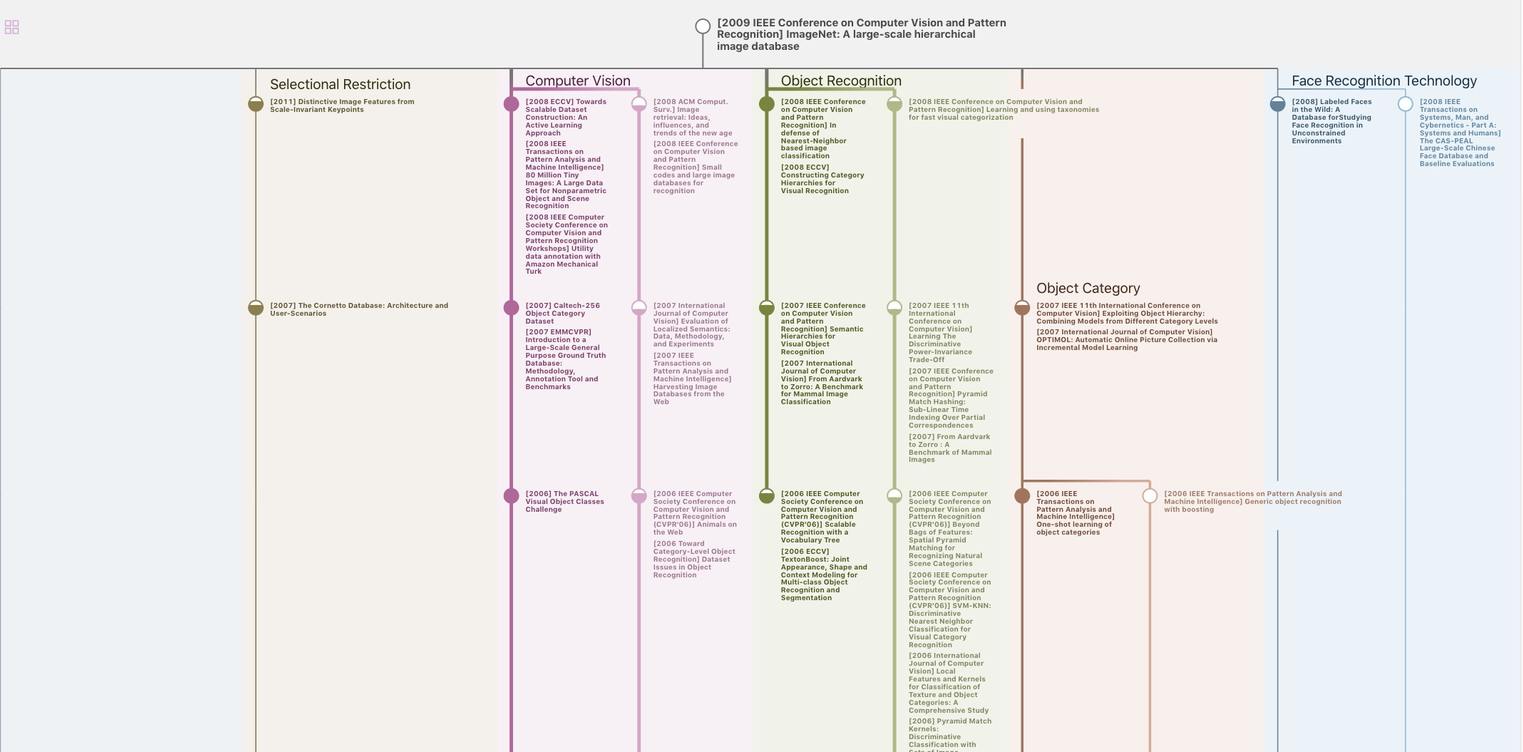
生成溯源树,研究论文发展脉络
Chat Paper
正在生成论文摘要