Tracking Tiny Insects in Cluttered Natural Environments using Refinable Recurrent Neural Networks.
IEEE/CVF Winter Conference on Applications of Computer Vision(2024)
摘要
Visual tracking of tiny and low-contrast objects such as insects in cluttered natural environments is a very challenging computer vision task. This is particularly true for machine learning algorithms, which usually require distinct visual foreground features to reliably identify the object of interest. Here, we propose a novel deep learning-based tracking framework capable of detecting tiny and visually camouflaged ants (covering only a few pixels) in complex and dynamic high-resolution videos. In particular, we introduce refinable recurrent Hourglass Networks, which combine color and temporal information to continuously detect insects recorded using a freely moving camera. Moreover, this architecture provides comprehensible heatmaps of positional estimations and a seamless integration of optional user-input to further refine the tracking results if necessary. We evaluated our algorithm on an extremely challenging wildlife ant dataset with a resolution of 1024 × 1024 and report a mean deviation of 19 pixels from the ground truth (object ≈ 30 px) without any user input. By providing only 0.6% manual locations this accuracy can be improved to a mean deviation of 9 pixels. A comparison to a well known deep learning-based single frame detection algorithm (YOLOv7), two state-of-the-art tracking methods (ToMP and KeepTrack), a probabilistic tracking framework and a comprehensive ablation study reveal superior performances in all our experiments. Our tracking framework therefore provides a foundation for challenging tiny singleobject tracking scenarios and a practical and interactive solution for biologists and ecologists.
更多查看译文
关键词
Applications,Animals / Insects,Algorithms,Video recognition and understanding,Applications,Environmental monitoring / climate change / ecology
AI 理解论文
溯源树
样例
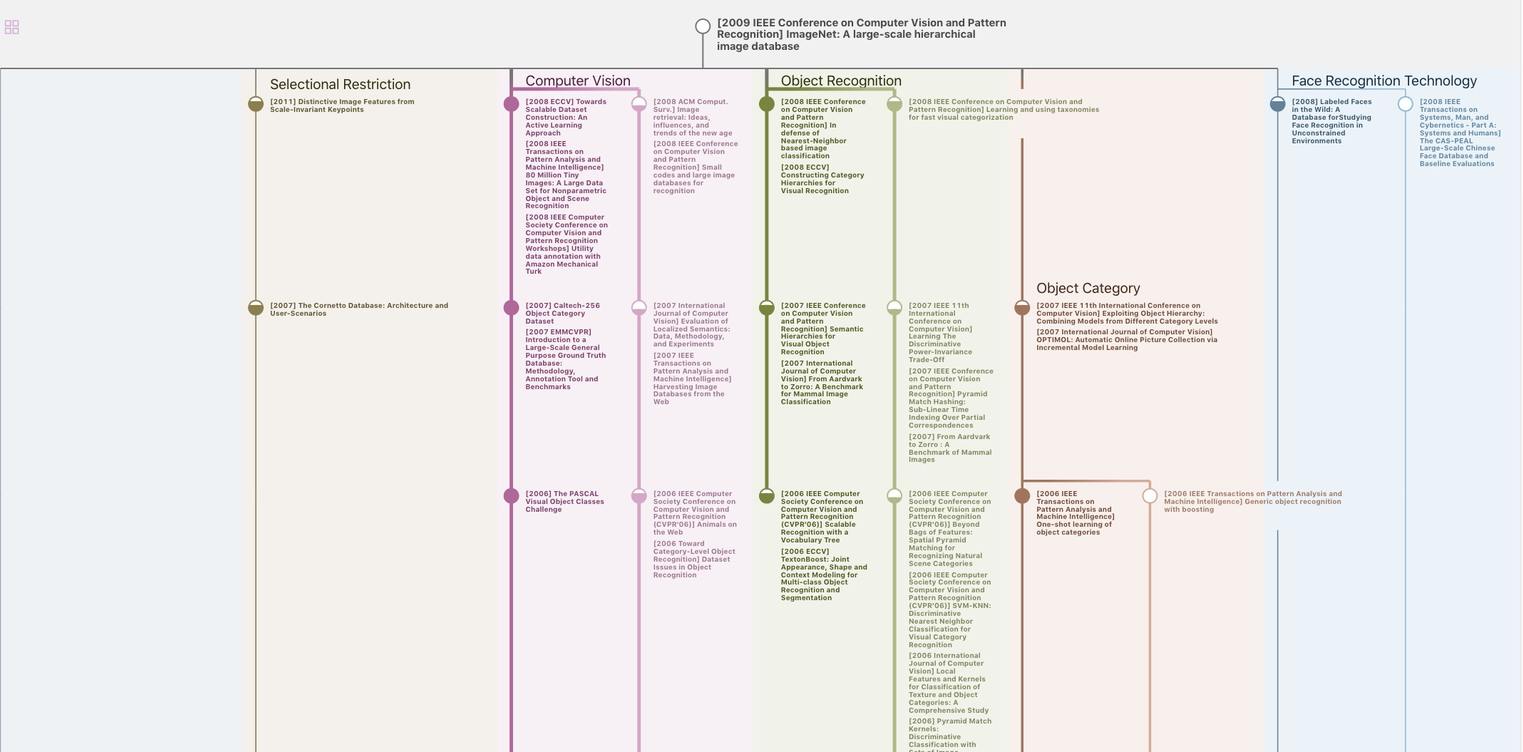
生成溯源树,研究论文发展脉络
Chat Paper
正在生成论文摘要