Online/Offline Learning to Enable Robust Beamforming: Limited Feedback Meets Deep Generative Models
arxiv(2024)
摘要
Robust beamforming is a pivotal technique in massive multiple-input
multiple-output (MIMO) systems as it mitigates interference among user
equipment (UE). One current risk-neutral approach to robust beamforming is the
stochastic weighted minimum mean square error method (WMMSE). However, this
method necessitates statistical channel information, which is typically
inaccessible, particularly in fifth-generation new radio frequency division
duplex cellular systems with limited feedback. To tackle this challenge, we
propose a novel approach that leverages a channel variational auto-encoder
(CVAE) to simulate channel behaviors using limited feedback, eliminating the
need for specific distribution assumptions present in existing methods. To
seamlessly integrate model learning into practical wireless communication
systems, this paper introduces two learning strategies to prepare the CVAE
model for practical deployment. Firstly, motivated by the digital twin
technology, we advocate employing a high-performance channel simulator to
generate training data, enabling pretraining of the proposed CVAE while
ensuring non-disruption to the practical wireless communication system.
Moreover, we present an alternative online method for CVAE learning, where
online training data is sourced based on channel estimations using Type II
codebook. Numerical results demonstrate the effectiveness of these strategies,
highlighting their exceptional performance in channel generation and robust
beamforming applications.
更多查看译文
AI 理解论文
溯源树
样例
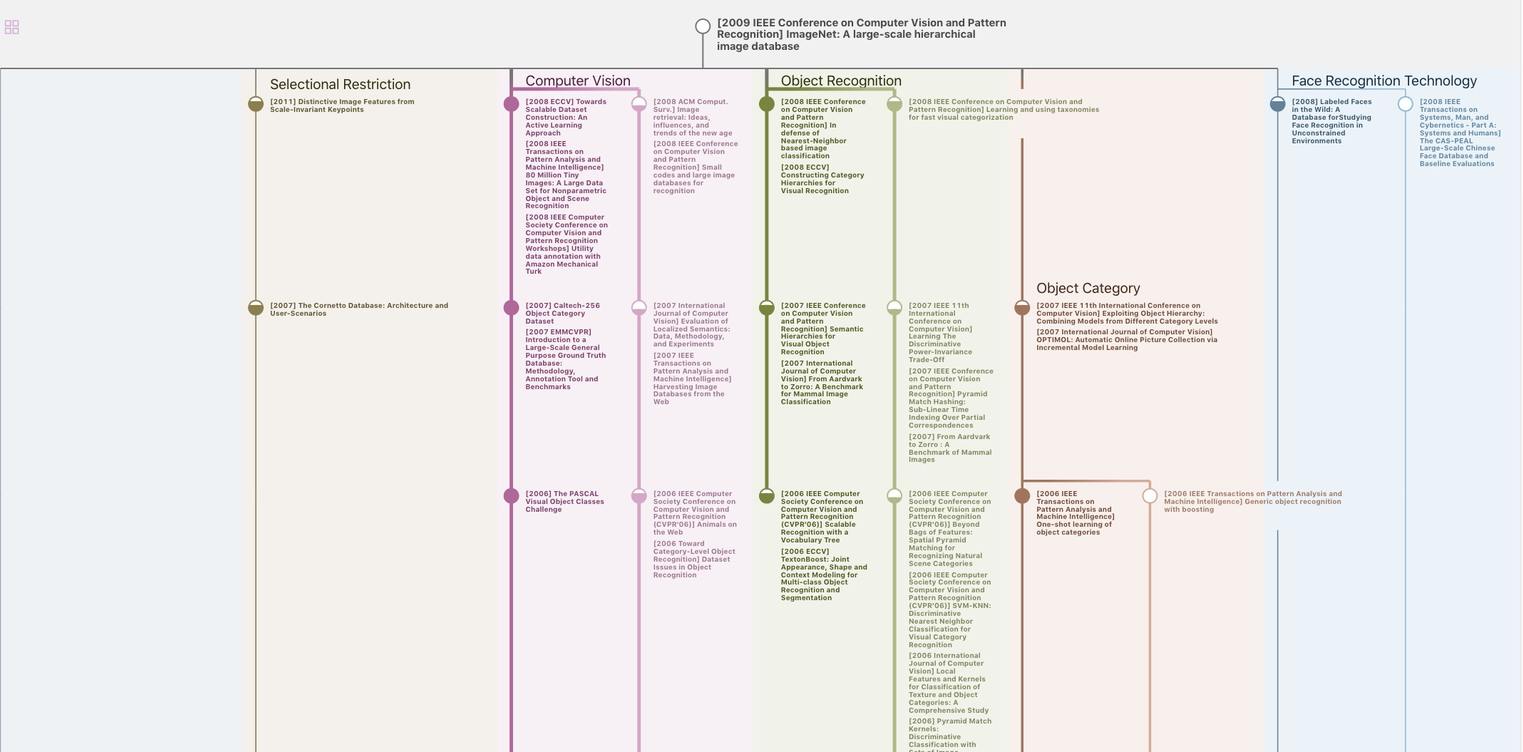
生成溯源树,研究论文发展脉络
Chat Paper
正在生成论文摘要