Semi-Supervised Scene Change Detection by Distillation from Feature-metric Alignment.
IEEE/CVF Winter Conference on Applications of Computer Vision(2024)
摘要
Scene change detection (SCD) is a critical task for various applications, such as visual surveillance, anomaly detection, and mobile robotics. Recently, supervised methods for SCD have been developed for urban and indoor environments where input image pairs are typically unaligned due to differences in camera viewpoints. However, supervised SCD methods require pixel-wise change labels and alignment labels for the target domain, which can be both time-consuming and expensive to collect. To tackle this issue, we design an unsupervised loss with regularization methods based on the feature-metric alignment of input image pairs. The proposed unsupervised loss enables the SCD model to jointly learn the flow and the change maps on the target domain. In addition, we propose a semi-supervised learning method based on a distillation loss for the robustness of the SCD model. The proposed learning method is based on the student-teacher structure and incorporates the unsupervised loss of the unlabeled target data and the supervised loss of the labeled synthetic data. Our method achieves considerable performance improvement on the target domain through the proposed unsupervised and distillation loss, using only 10% of the target training dataset without using any labels of the target data.
更多查看译文
关键词
Algorithms,Image recognition and understanding
AI 理解论文
溯源树
样例
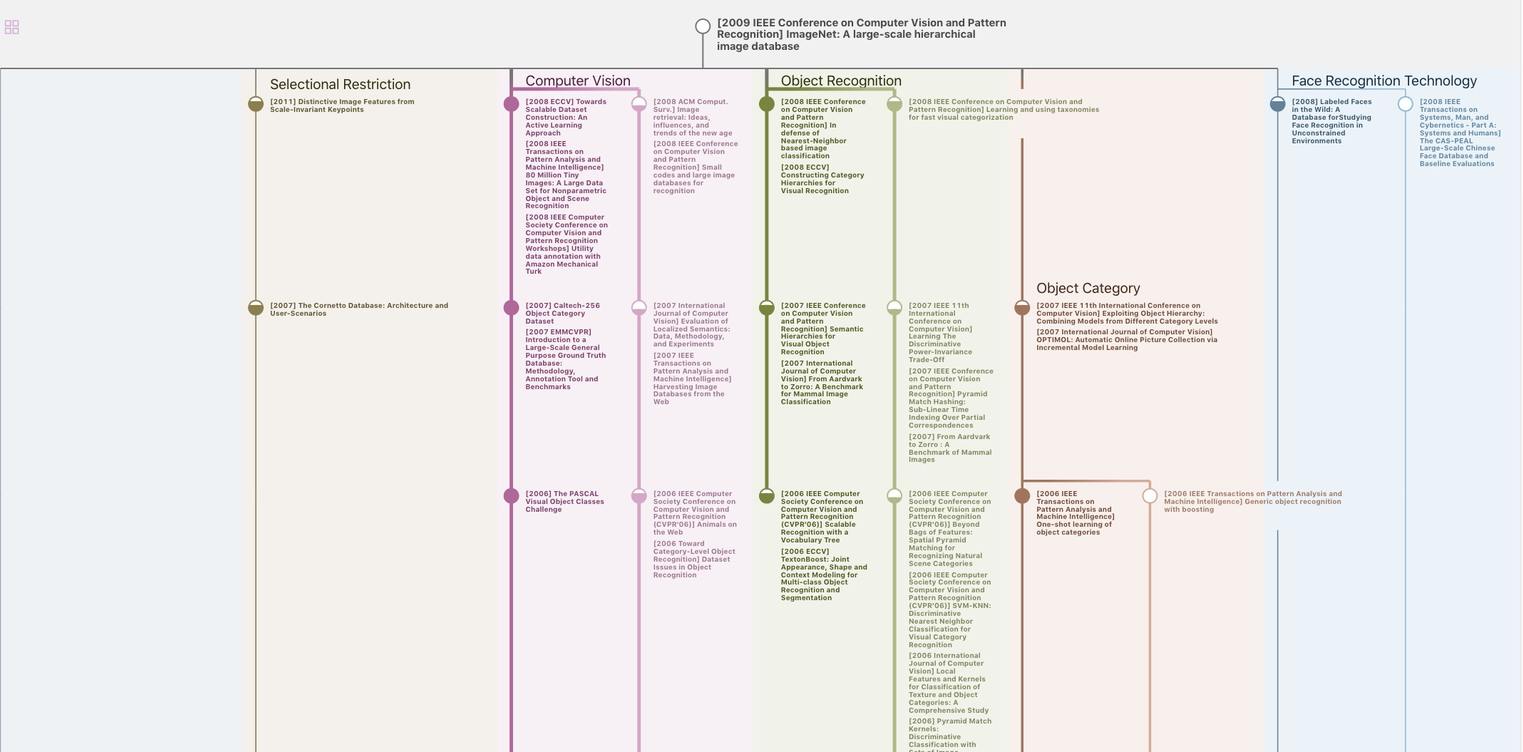
生成溯源树,研究论文发展脉络
Chat Paper
正在生成论文摘要