D4: Detection of Adversarial Diffusion Deepfakes Using Disjoint Ensembles.
IEEE/CVF Winter Conference on Applications of Computer Vision(2024)
摘要
Detecting diffusion-generated deepfake images remains an open problem. Current detection methods fail against an adversary who adds imperceptible adversarial perturbations to the deepfake to evade detection. In this work, we propose Disjoint Diffusion Deepfake Detection (D4), a deepfake detector designed to improve black-box adversarial robustness beyond de facto solutions such as adversarial training. D4 uses an ensemble of models over disjoint subsets of the frequency spectrum to significantly improve adversarial robustness. Our key insight is to leverage a redundancy in the frequency domain and apply a saliency partitioning technique to disjointly distribute frequency components across multiple models. We formally prove that these disjoint ensembles lead to a reduction in the dimensionality of the input subspace where adversarial deepfakes lie, thereby making adversarial deepfakes harder to find for black-box attacks. We then empirically validate the D4 method against several black-box attacks and find that D4 significantly outperforms existing state-of-the-art defenses applied to diffusion-generated deepfake detection. We also demonstrate that D4 provides robustness against adversarial deepfakes from unseen data distributions as well as unseen generative techniques.
更多查看译文
关键词
Algorithms,Adversarial learning,adversarial attack and defense methods,Algorithms,Generative models for image,video,3D,etc
AI 理解论文
溯源树
样例
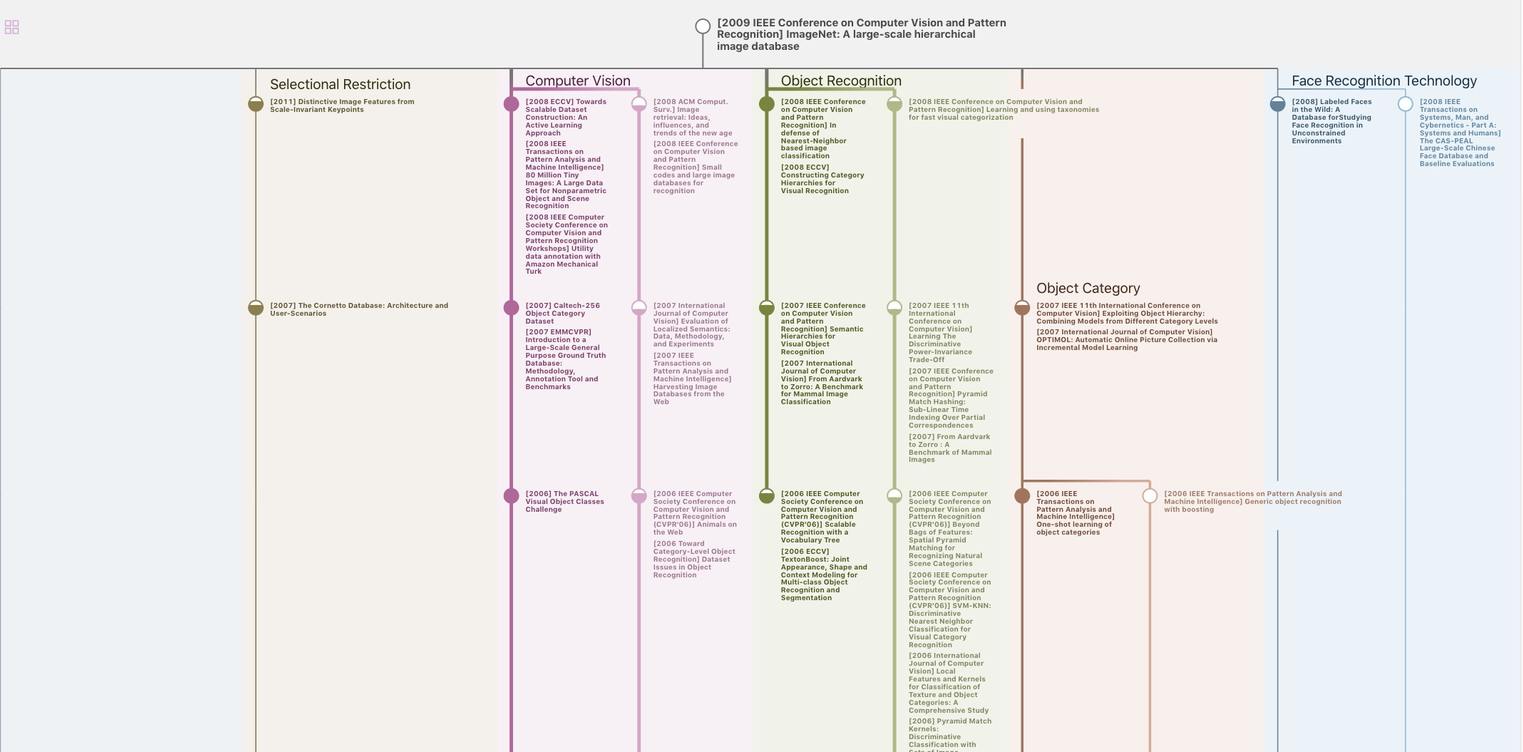
生成溯源树,研究论文发展脉络
Chat Paper
正在生成论文摘要