A Novel MADRL with Spatial-Temporal Pattern Capturing Ability for Robust Decentralized Control of Multiple Microgrids under Anomalous Measurements
IEEE Transactions on Sustainable Energy(2024)
摘要
The anomalous measurements pose significant challenges for the secure and economical operation of multiple microgrids (MMGs). However, existing works still cannot effectively address this problem. Therefore, this paper proposes a robust decentralized multi-agent deep reinforcement learning (MADRL) control approach by developing a novel actor network on the basis of the centralized training and decentralized execution framework (CTDEF). To achieve robust control of MMG systems against anomalous data, this approach extracts the variation patterns of measurements from both temporal and spatial perspectives. From the spatial perspective, the measurements are first cast to a graph, and a multi-head graph attention (MGAT) network is employed to extract the structural correlations among these measurements. From the temporal perspective, the measurements feature extracted by MGAT along with the state and historical actions are processed by two recurrent networks to obtain the trajectory history feature of each MG. The confederate image technology is developed therein for each agent to infer the intentions of other agents in order to better extract the trajectory history. To more fully express the structural correlations between nodes and decision intentions of other agents, a node cognition regularizer and a mutual information-based regularization term are designed for optimizing MGAT and the confederate image network, respectively. By integrating temporal and spatial perspectives, the proposed approach achieves greater robustness to outliers than approaches that consider only one perspective. The experimental results confirm the effectiveness of the proposed approach.
更多查看译文
关键词
Multi-agent deep reinforcement learning,Anomalous measurements,Coordination of the multiple microgrids
AI 理解论文
溯源树
样例
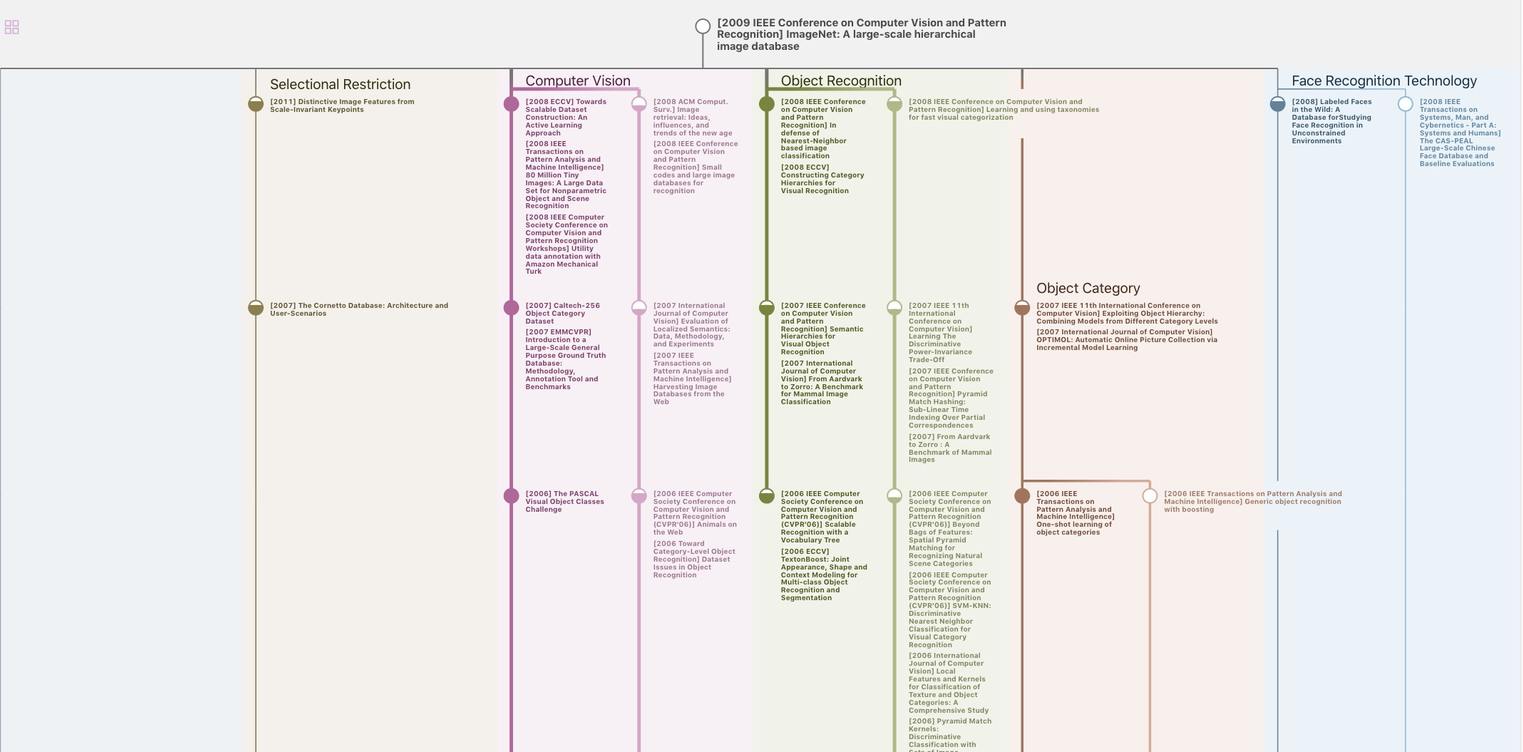
生成溯源树,研究论文发展脉络
Chat Paper
正在生成论文摘要