RIA-CSM2: A Real-Time Impact-Aware Correlative Scan Matching Algorithm Using Heterogeneous Multi-Core SoC for Low-Cost Wheeled Robots
IEEE Transactions on Instrumentation and Measurement(2024)
摘要
As a classic scan-to-map matching method, the correlative scan matching (CSM) algorithm may not be applicable if low-cost wheeled robots (like robot cleaners) are impacted. The first open issue is heavy dependence on trustworthy initial poses. Side slips caused by impacts are unobservable for rotary encoders mounted on wheels, leading to huge localization errors. The second open issue is the efficient processing of global localization using the CSM algorithm, which is essential for impacted robots. The state-of-the-art hardware designs for large-scale multi-resolution CSM have low energy efficiency. These two open issues are properly addressed in this work, namely RIA-CSM2. Based on lightweight deep neural networks, we perform reliable impact detection in unforeseen environments using only low-cost proprioceptive sensors. To bind the rapid error growth of conventional wheel-aided inertial navigation systems (INS), delayed out-of-sequence measurements from CSM algorithms are integrated into an Extended Kalman Filter (EKF). The lightweight impact detection networks and INS are generally applicable for most embedded robotic systems with stringent energy, computing, and memory cost limitations. Once impacts are detected, large-scale multi-resolution CSM algorithms will be performed on an energy-efficient hardware accelerator. Extensive experiments based on public datasets show our work achieves high real-time performance and energy efficiency. The frame rate of local-scale high-resolution CSM can reach up to 96.42 fps. Field experiments on wheeled robot platforms demonstrate the effectiveness of our impact detection network, which outperforms our preliminary work in precision and false-alarm rate by a significant margin, with precision and recall rates reaching 100% and 97.8% respectively. The presented impact detection datasets are now publicly available.
更多查看译文
关键词
deep learning,impact-aware,inertial measurement units,Kalman filter,multi-sensor fusion,side slip
AI 理解论文
溯源树
样例
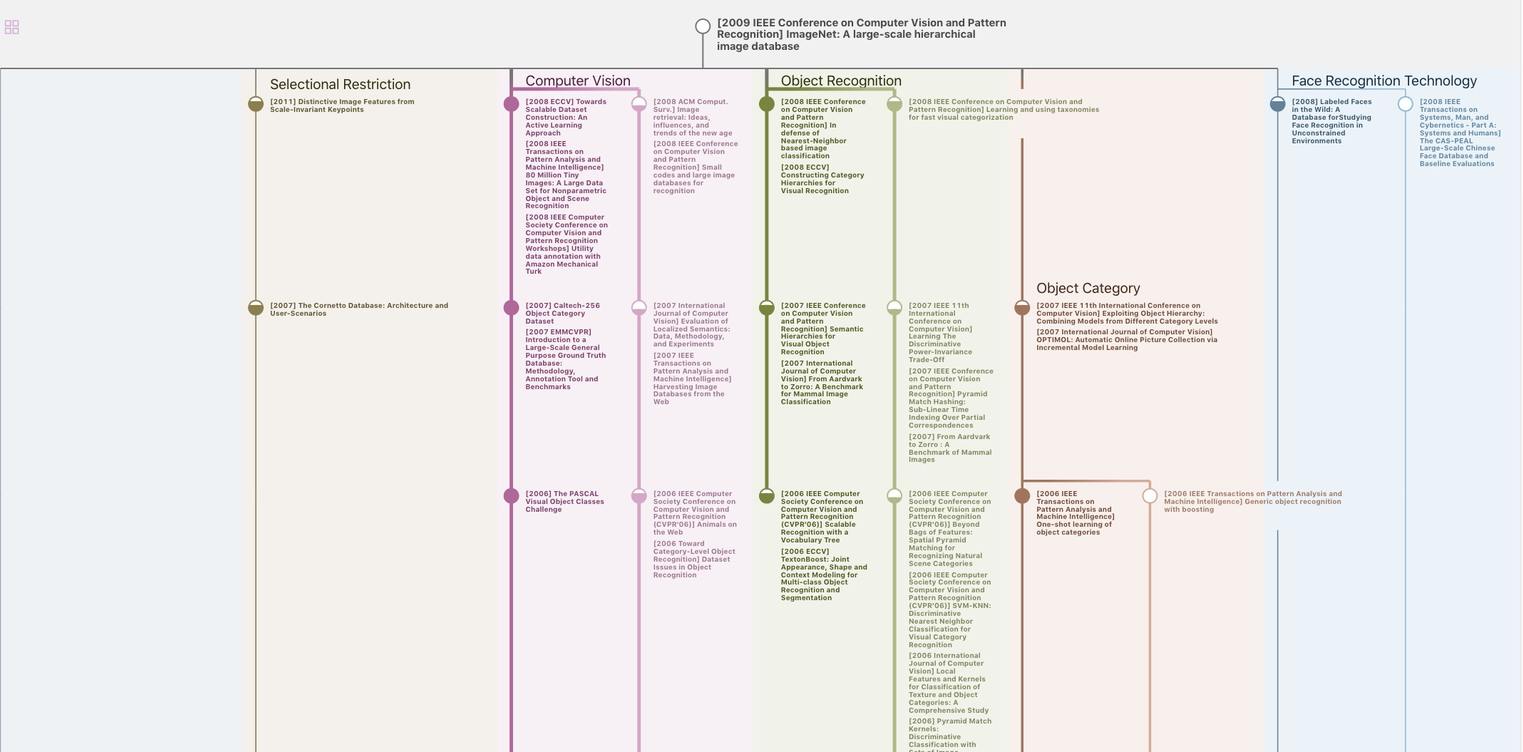
生成溯源树,研究论文发展脉络
Chat Paper
正在生成论文摘要