Partial Label Learning with a Partner
AAAI 2024(2024)
摘要
In partial label learning (PLL), each instance is associated with a set of candidate labels among which only one is ground-truth. The majority of the existing works focuses on constructing robust classifiers to estimate the labeling confidence of candidate labels in order to identify the correct one. However, these methods usually struggle to rectify mislabeled samples. To help existing PLL methods identify and rectify mislabeled samples, in this paper, we introduce a novel partner classifier and propose a novel ``mutual supervision'' paradigm. Specifically, we instantiate the partner classifier predicated on the implicit fact that non-candidate labels of a sample should not be assigned to it, which is inherently accurate and has not been fully investigated in PLL. Furthermore, a novel collaborative term is formulated to link the base classifier and the partner one. During each stage of mutual supervision, both classifiers will blur each other's predictions through a blurring mechanism to prevent overconfidence in a specific label. Extensive experiments demonstrate that the performance and disambiguation ability of several well-established stand-alone and deep-learning based PLL approaches can be significantly improved by coupling with this learning paradigm.
更多查看译文
关键词
ML: Classification and Regression,ML: Multi-class/Multi-label Learning & Extreme Classification
AI 理解论文
溯源树
样例
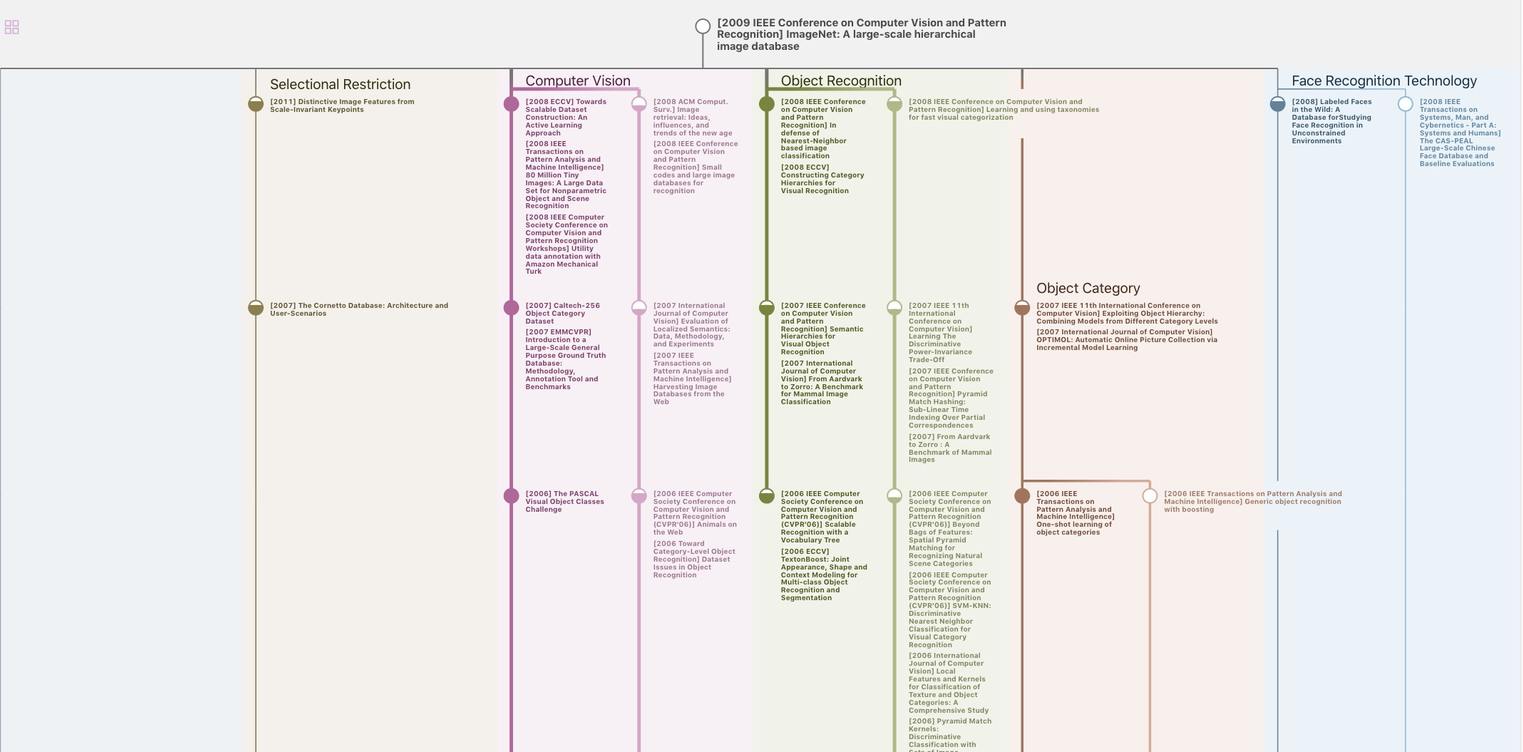
生成溯源树,研究论文发展脉络
Chat Paper
正在生成论文摘要