PSTNet: Transformer for aggregating neighborhood features in 3D point cloud semantic segmentation of eggplant plants
Scientia Horticulturae(2024)
摘要
Improving the quality of plant point cloud data and achieving precise segmentation is essential for effective plant phenotyping analysis and plant breeding. To ensure data quality and accuracy of segmentation, we employed a self-developed three-dimensional scanning device based on binocular vision to acquire eggplant plant point clouds with high spatial resolution. And we improved down-sampling algorithm Octree Farthest Point Sampling (OFPS) to process the original point cloud data. Furthermore, we proposed a neural network called Plant Segmentation Transformer Network (PSTNet) to achieve semantic segmentation of high spatial resolution eggplant plant datasets. PSTNet comprises the following components: (i) a Neighborhood Feature Aggregator (NPA) for storing and aggregating local neighborhood features of input points, and (ii) a cascaded Point Self-Attention module (PSA) for capturing contextual information. Experimental results demonstrate the remarkable performance of PSTNet in semantic segmentation tasks, with IoU, Precision, Recall, F1-score, and Accuracy values of 92.20 %, 95.30 %, 95.57 %, 95.43 %, and 95.15 %, respectively. Additionally, compared to the Point Cloud Transformer (PCT), which exhibited the second-best performance, PSTNet achieved improvements of 4.37, 1.47, 2.87, 2.17, and 4.22 percentage points in the abovementioned metrics. This method achieves high-precision segmentation of plant point clouds, expands the semantic segmentation method of plants, and lays a solid foundation for plant phenotypic analysis.
更多查看译文
关键词
Sementic segmentation,Plant phenotyping,Point cloud,3D scanning
AI 理解论文
溯源树
样例
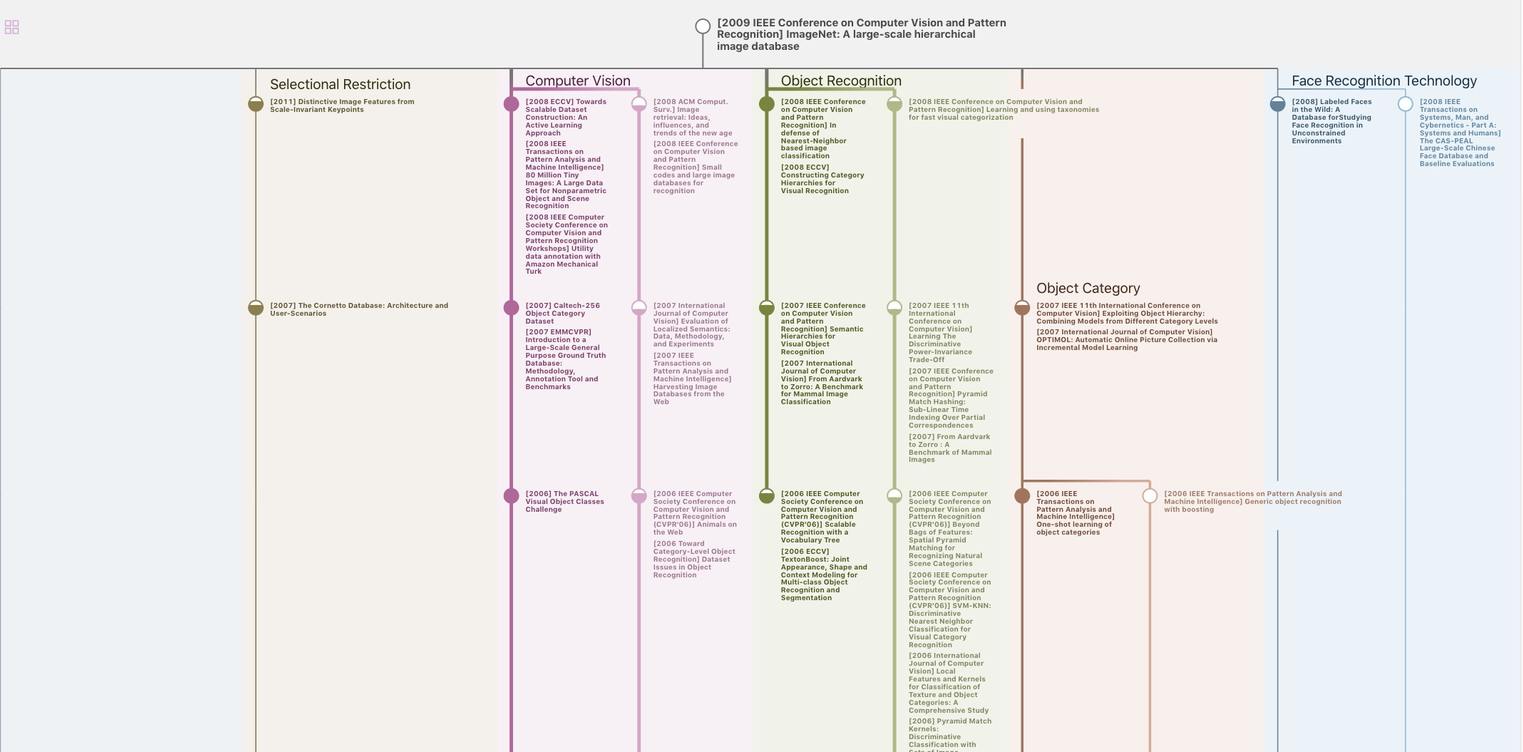
生成溯源树,研究论文发展脉络
Chat Paper
正在生成论文摘要