1. Wildlife and Ecosystem Health
mag(2012)
Key words
agricultural sciences,veterinary science
AI Read Science
Must-Reading Tree
Example
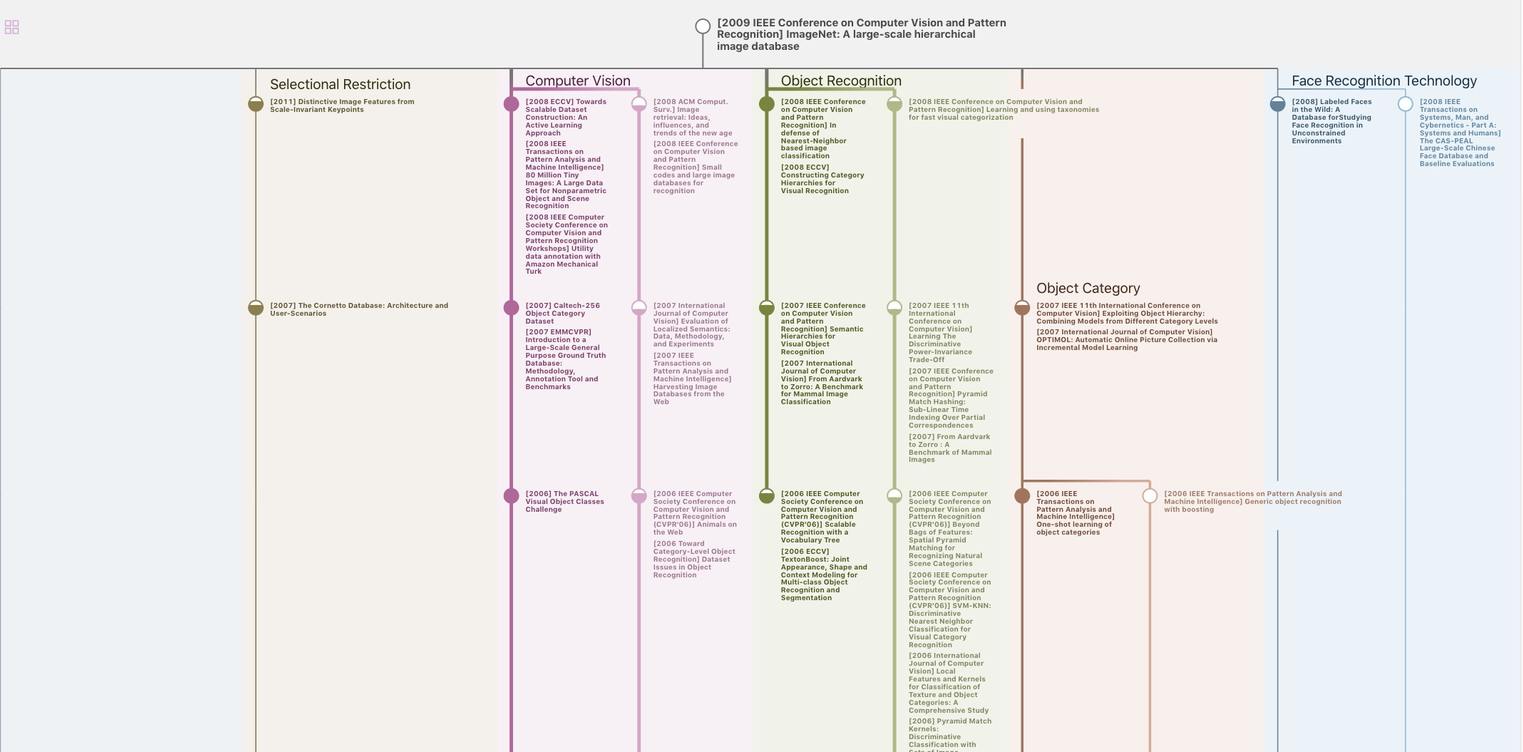
Generate MRT to find the research sequence of this paper
Chat Paper
Summary is being generated by the instructions you defined