Uncertainty-Aware Suction Grasping for Cluttered Scenes
IEEE Robotics and Automation Letters(2024)
摘要
In this work, we present a multi-stage pipeline that aims to accurately predict suction grasps for objects with varying properties in cluttered and complex scenes. Existing methods face difficulties in generalizing to unseen objects and effectively handling noisy depth/point cloud data, which often leads to inaccurate grasp predictions. To address these challenges, we utilize the Unseen Object Instance Segmentation (UOIS) technique to segment all objects and extract their instance-level point clouds. Additionally, we introduce the Uncertainty-aware Instance-level Scoring Network (UISN) to generate point-wise suction scores with uncertainties for each object. By calibrating the predicted scores using the estimated uncertainties, we further enhance their reliability for unseen objects and noisy data. Finally, the grasping candidates are ranked based on the calibrated scores, then the most promising grasps can be executed. Our approach achieves state-of-the-art performance on the SuctionNet-1Billion benchmark and demonstrates real robotic grasping, showcasing its accuracy and robustness in cluttered scenes. The code, models, and datasets for training and evaluation are accessible at
https://github.com/rcao-hk/UISN
.
更多查看译文
关键词
Deep learning in grasping and manipulation,perception for grasping and manipulation,computer vision for automation
AI 理解论文
溯源树
样例
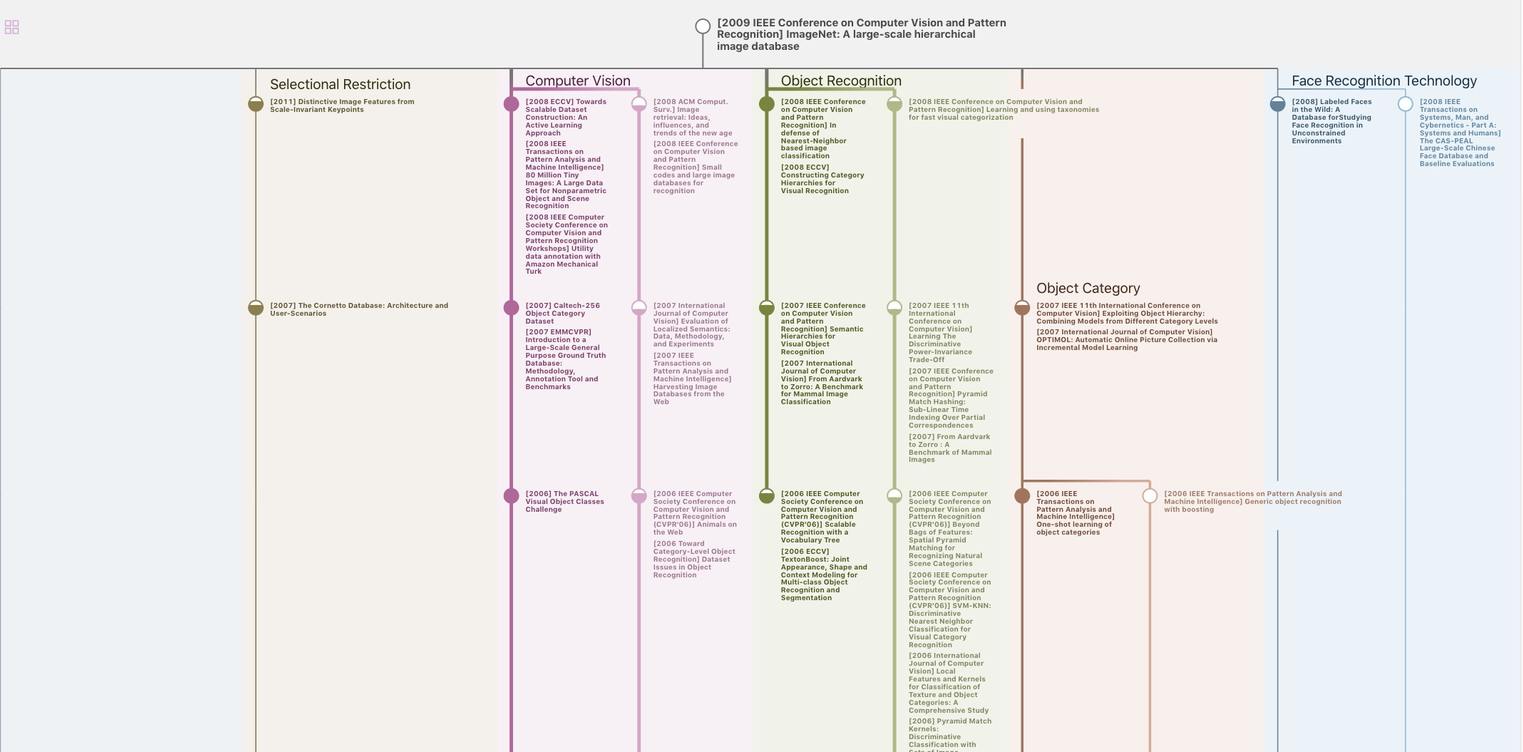
生成溯源树,研究论文发展脉络
Chat Paper
正在生成论文摘要