A machine learning-response surface optimization approach to enhance the performance of diesel engine using novel blends of aloe vera biodiesel with MWCNT nanoparticles and hydrogen
Process Safety and Environmental Protection(2024)
摘要
This study aims to optimize the performance and exhaust emission characteristics of a dual-fuel diesel engine using wastewater-derived oxyhydrogen and nanoparticle-dispersed biodiesel/diesel blends. A Box Behnken Design (BBD) with L46 orthogonal arrays is employed to experiment with five factors and three levels of parameters: engine load, compression ratio, blend ratio, nanoparticle concentration, and oxyhydrogen (HHO) flow rate. In addition, six different machine learning algorithms (DTR, ABR, ETR, GBR, LGBM, and XGBR) are used to develop a prognostic model based on the acquired experimental data. These models estimate key performance indicators such as BTE, BSFC, CO, HC, and NOx. Based on the evaluation of performance indicators, including R2 (0.9729–0.9991), RMSE (0.0021–4.5765), MAE (0.0014–3.0015), and MAPE (2.37–4.83), the XGBR model demonstrates the most accurate predictions. Furthermore, the RSM-based desirability approach evaluates the trade-off between performance and emissions, thereby identifying the optimal engine operating condition. This condition includes a load of 7.17kg, a compression ratio of 18:1, a blend ratio of 10%, a nanoparticle concentration of 143 ppm, and an HHO flow rate of 5.57L/min. The findings from the RSM analysis are validated through experimental investigation, demonstrating an error margin of 7%. Overall, the introduction of oxyhydrogen improves combustion in a nanoparticle-dispersed biodiesel-diesel blend-powered diesel engine, resulting in increased engine performance and reduced carbon-based emissions, except for nitrogen oxide emissions.
更多查看译文
关键词
Oxyhydrogen,Nanoparticle,Aloe Vera Biodiesel,Performance and emission analysis,Machine learning
AI 理解论文
溯源树
样例
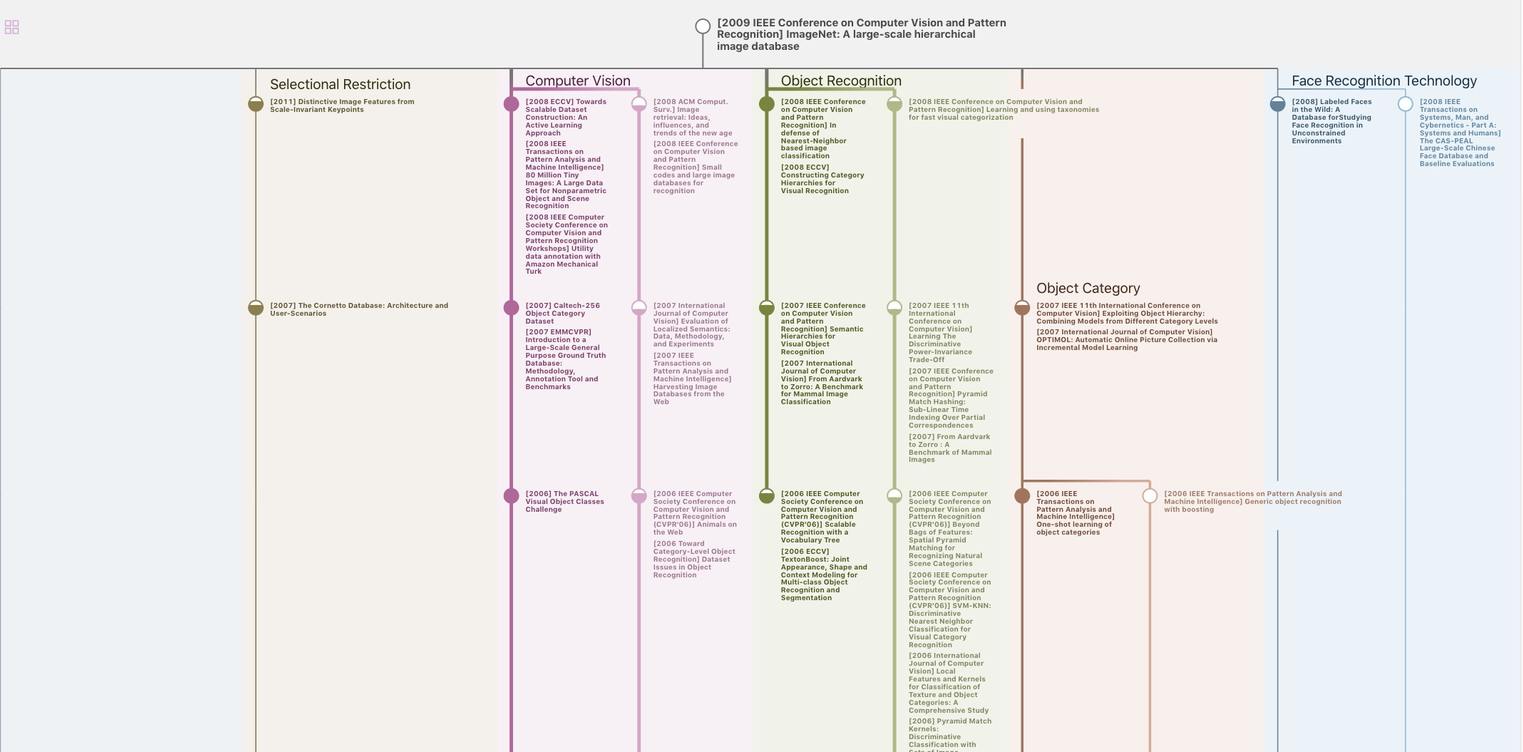
生成溯源树,研究论文发展脉络
Chat Paper
正在生成论文摘要