Continual Cross-domain Image Compression via Entropy Prior Guided Knowledge Distillation and Scalable Decoding
IEEE Transactions on Circuits and Systems for Video Technology(2024)
摘要
Learning based image compression has achieved impressive rate-distortion performance in recent years. However, due to the disposable learning strategy and rigid network architecture, existing methods perform poorly for compressing the images of different domains when they emerge with the expanding real-world applications, such as, natural, oil painting, medical images and so on. To cope with this open-world challenge, this paper proposes a continual cross-domain image compression method based on entropy prior guided knowledge distillation and scalable decoding network, which perform well in balancing the plasticity, stability and compatibility. Firstly, we generate pseudo-samples of old domains by reusing their entropy priors. These pseudo-samples serve as guides for knowledge distillation in the old domains, ensuring that the bit rate and reconstruction of the new model align with those of the old model. This approach assists the updated model in retaining its capability to compress and reconstruct old images. Secondly, we develop a scalable decoding network via dynamic pruning and masked recovery, which could effectively infer an old entropy decoder from the latestly updated model. It ensures that the updated model could decode image features from binary strings encoded by old entropy encoders. Experiments on five image datasets with different domains demonstrate the effectiveness of the proposed method and its superiority over representative continual learning methods. Code of the proposed method is available at https://github.com/wuchenhaoo/Continual_Cross-domain_Image_Compression/.
更多查看译文
关键词
Cross-domain image compression,continual learning,knowledge distillation,scalable decoding
AI 理解论文
溯源树
样例
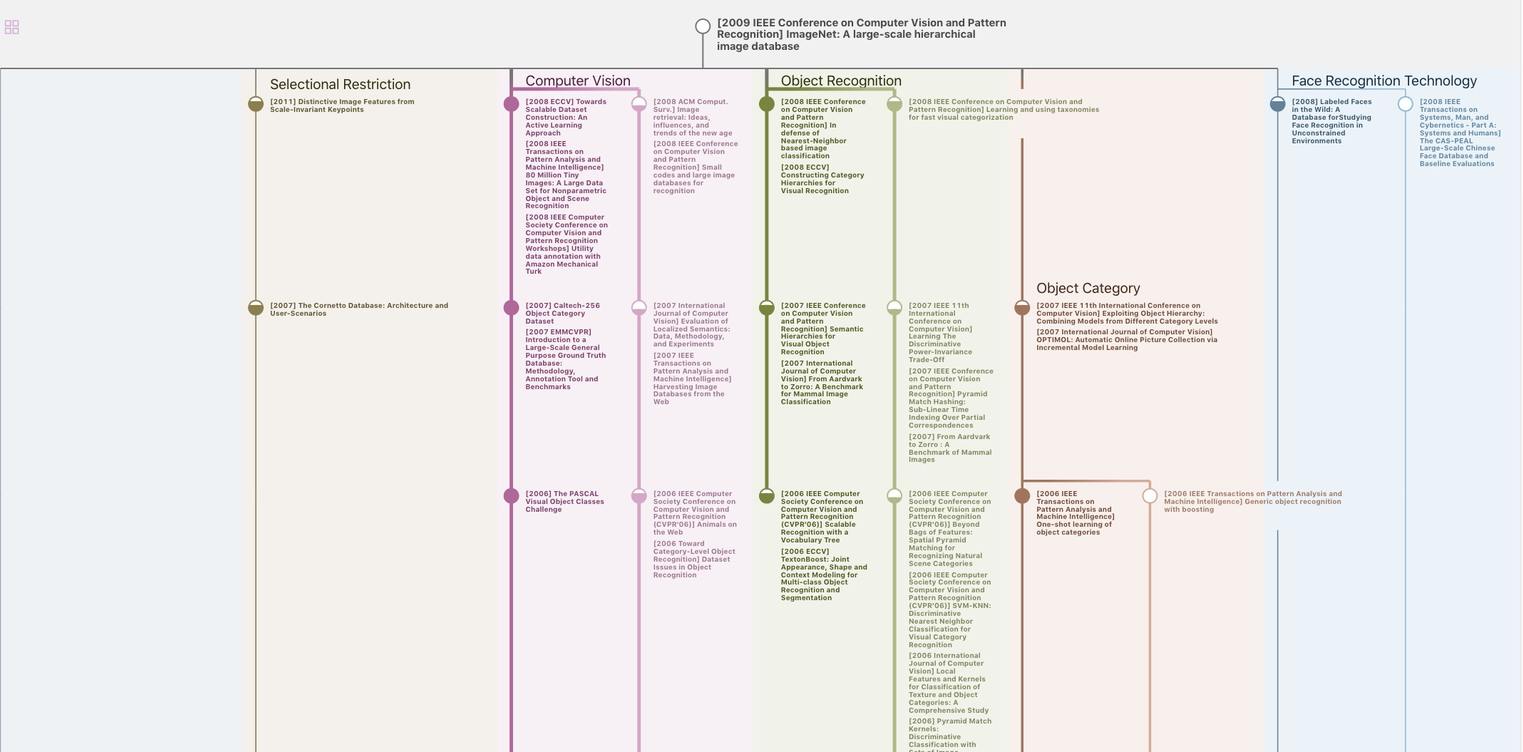
生成溯源树,研究论文发展脉络
Chat Paper
正在生成论文摘要