Correlation-based feature selection and parallel spatiotemporal networks for efficient passenger flow forecasting in metro systems
TRANSPORTMETRICA A-TRANSPORT SCIENCE(2024)
摘要
This paper presents a novel framework for predicting metro passenger flow that is both interpretable and computationally efficient. The proposed method first uses a correlation-based spatiotemporal feature selection strategy (Cor-STFS) to identify the optimal input scheme for the prediction model, effectively reducing unnecessary interference. The framework then introduces a new multivariate passenger flow prediction architecture called STA-PTCN-BiGRU, which combines a spatiotemporal attention (STA) mechanism, parallel temporal convolutional networks (PTCN), and bidirectional gated recurrent units (BiGRU) to capture the dynamic internal patterns of passenger flow. By utilising parallel computing, this architecture significantly reduces resource consumption. The effectiveness of the proposed approach is evaluated using four datasets from the Shanghai Metro. Experimental results show that the new method outperforms baseline approaches in terms of root mean square error (RMSE), mean absolute error (MAE), and symmetric mean absolute percentage error (SMAPE), achieving average reductions of 9.98%, 8.08%, and 13.29% in these metrics, respectively.
更多查看译文
关键词
Traffic forecasting,Deep learning,Spatiotemporal dependence,Feature extraction,Metro domain knowledge
AI 理解论文
溯源树
样例
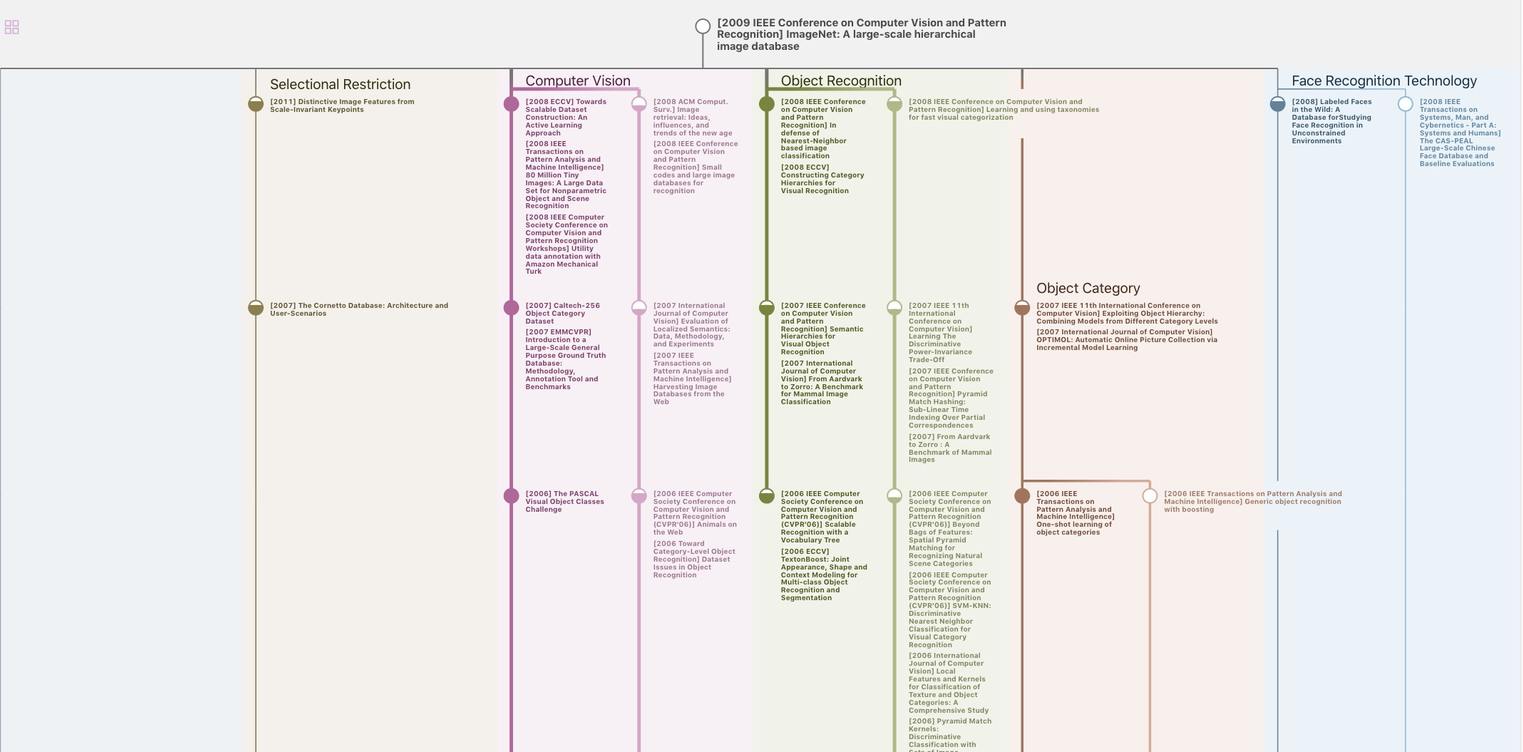
生成溯源树,研究论文发展脉络
Chat Paper
正在生成论文摘要