A bias-corrected GEMS geostationary satellite product for nitrogen dioxide using machine learning to enforce consistency with the TROPOMI satellite instrument
crossref(2024)
摘要
Abstract. The Geostationary Environment Monitoring Spectrometer (GEMS) launched in February 2020 is now providing continuous daytime hourly observations of nitrogen dioxide (NO2) columns over East Asia (5° S–45° N, 75° E–145° E) with 3.5 × 7.7 km2 pixel resolution. These data provide unique information to improve understanding of the sources, chemistry, and transport of nitrogen oxides (NOx) with implications for atmospheric chemistry and air quality, but opportunities for direct validation are very limited. Here we correct the operational level-2 (L2) NO2 vertical column densities (VCDs) from GEMS with a machine learning (ML) model to match the much sparser but more mature observations from the low Earth orbit TROPOspheric Monitoring Instrument (TROPOMI), preserving the data density of GEMS but making them consistent with TROPOMI. We first reprocess the GEMS and TROPOMI operational L2 products to use common prior vertical NO2 profiles (shape factors) from the GEOS-Chem chemical transport model. This removes a major inconsistency between the two satellite products and greatly improves their agreement with ground-based Pandora NO2 VCD data in source regions. We then apply the ML model to correct the remaining differences, Δ(GEMS-TROPOMI), using as predictor variables the GEMS NO2 VCDs and retrieval parameters. We train the ML model with collocated GEMS and TROPOMI NO2 VCDs, taking advantage of TROPOMI off-track viewing to cover a wide range of effective zenith angles (EZAs) for the GEMS diurnal profiles. The two most important predictor variables for Δ(GEMS-TROPOMI) are GEMS NO2 VCD and EZA. The corrected GEMS product is unbiased relative to TROPOMI and shows a diurnal variation over source regions more consistent with Pandora than the operational product.
更多查看译文
AI 理解论文
溯源树
样例
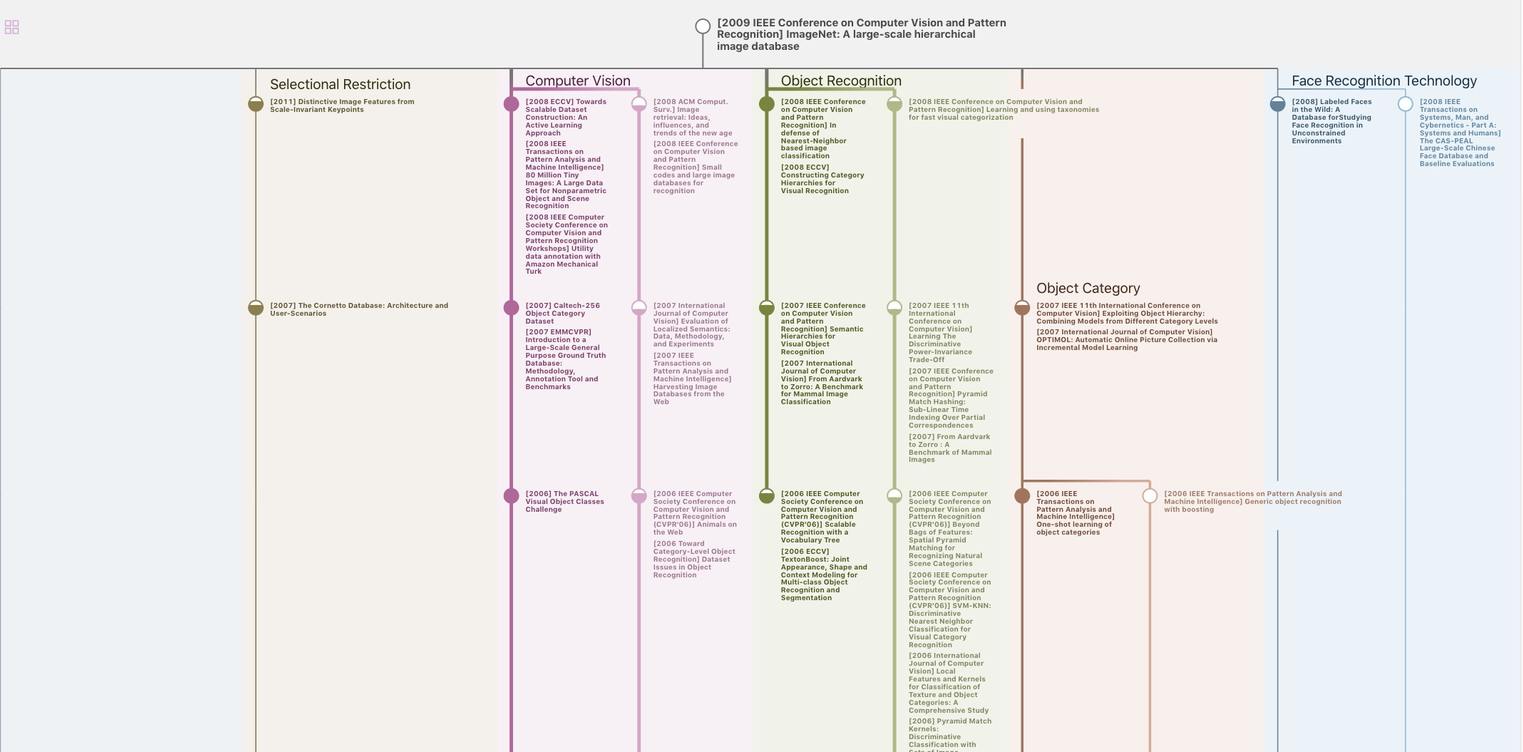
生成溯源树,研究论文发展脉络
Chat Paper
正在生成论文摘要