Cubature particle filtering fusion with descent gradient and maximum correntropy for non-Gaussian noise
Neurocomputing(2024)
摘要
To address the challenges posed by known heavy-tailed non-Gaussian noise characteristics in multi-sensor fusion, such as complex modeling, reduced estimation accuracy, and inadequate real-time performance, a non-Gaussian cubature particle filter sequential fusion method based on Gradient Descent (GD) and Maximum Correntropy Criterion (MCC) is proposed. Firstly, the proposed approach employs the gradient descent technique to minimize the Pseudo-Huber loss function. This optimization aims to approximate a known heavy-tailed non-Gaussian distribution to a Gaussian distribution, thereby reducing the complexity associated with modeling non-Gaussian noise. Furthermore, by incorporating the MCC into the Cubature Particle Filtering (CPF) process, we achieve real-time optimal estimation of the state, leading to a further improvement in the accuracy of the filter. Finally, a sequential fusion approach is employed for multi-sensor fusion, ensuring real-time filtering and fusion of measurement values. The simulation results demonstrate that in the multi-sensor fusion problem under non-Gaussian noise, the proposed fusion method in this paper can effectively reduce the algorithm’s time complexity while ensuring accuracy.
更多查看译文
关键词
Non-Gaussian,Cubature particle filters,Gradient descent,Maximum correntropy criterion,Sequential fusion
AI 理解论文
溯源树
样例
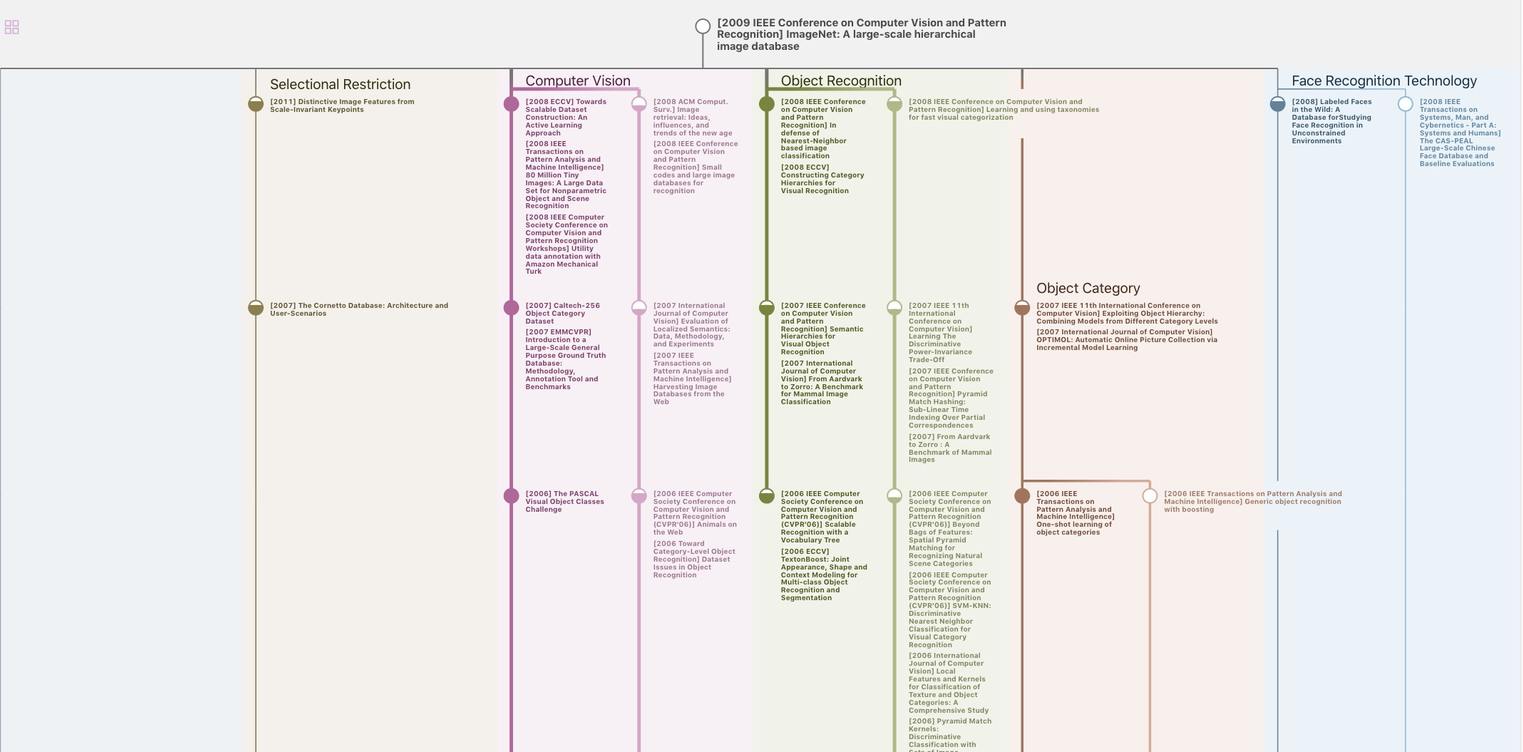
生成溯源树,研究论文发展脉络
Chat Paper
正在生成论文摘要