AI-based macro model learning for high cycle fatigue assessment of welded joints in large-span steel structures
International Journal of Fatigue(2024)
摘要
Welded spherical joints are critical components in large-span bridges and spatial steel structures prone to high cycle fatigue (HCF) loadings. Challenges exist for assessing fatigue life of this joints in small data from physical experiments and no detailed fatigue classification in design codes. This paper investigates the fatigue resistance of welded spherical joints and the real-time pre-damage evolution under non-stationary HCF loadings by proposing AI-based macro model learning from fine FE models and fatigue tests. Firstly, the structural features of welded spherical joints about stress concentration and weld defects were analyzed and quantified, so modified S-N curve was built. Then, based on artificial intelligence (AI-based) learning, stress concentration factors (SCF) were obtained by an artificial neural network with particle swarm optimization and the modified fatigue resistance of welded spherical joints was derived by artificial bee colony algorithms. Finally, the macro linkage element was proposed as a user element subroutine (UEL), by which non-stationary fatigue pre-damage evolution based on the time-domain incremental analytical method was realized. The results not only indicated the damage value close to the ones derived by commonly used rain flow count methods, but also provided the pre-damage evolution curve for real-time monitoring.
更多查看译文
关键词
AI-based model learning,Welded spherical joints,Fatigue resistance,Time-domain incremental method,Pre-damage evolution analysis
AI 理解论文
溯源树
样例
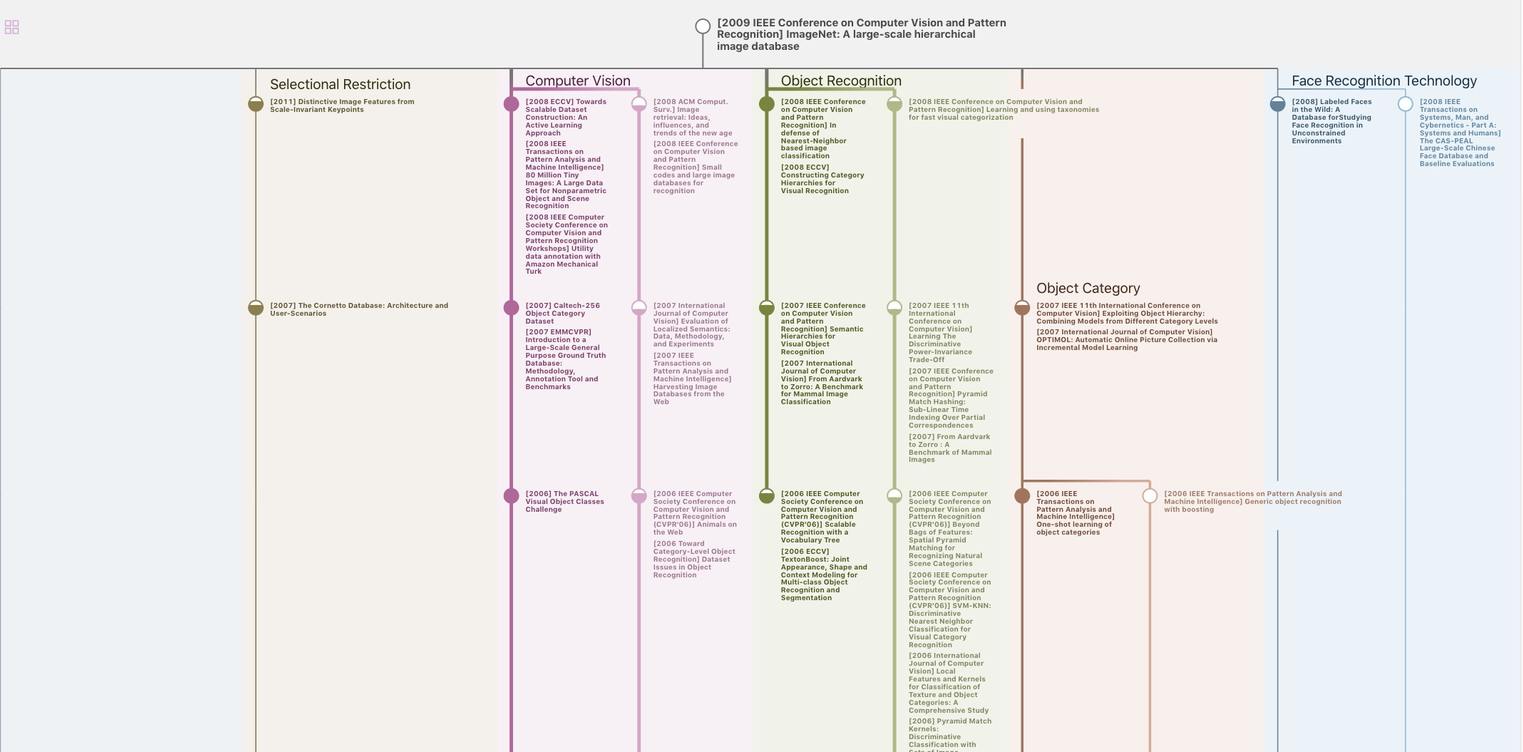
生成溯源树,研究论文发展脉络
Chat Paper
正在生成论文摘要