Mining Area Skyline Objects from Map-based Big Data using Apache Spark Framework
arxiv(2024)
摘要
The computation of the skyline provides a mechanism for utilizing multiple
location-based criteria to identify optimal data points. However, the
efficiency of these computations diminishes and becomes more challenging as the
input data expands. This study presents a novel algorithm aimed at mitigating
this challenge by harnessing the capabilities of Apache Spark, a distributed
processing platform, for conducting area skyline computations. The proposed
algorithm enhances processing speed and scalability. In particular, our
algorithm encompasses three key phases: the computation of distances between
data points, the generation of distance tuples, and the execution of the
skyline operators. Notably, the second phase employs a local partial skyline
extraction technique to minimize the volume of data transmitted from each
executor (a parallel processing procedure) to the driver (a central processing
procedure). Afterwards, the driver processes the received data to determine the
final skyline and creates filters to exclude irrelevant points. Extensive
experimentation on eight datasets reveals that our algorithm significantly
reduces both data size and computation time required for area skyline
computation.
更多查看译文
AI 理解论文
溯源树
样例
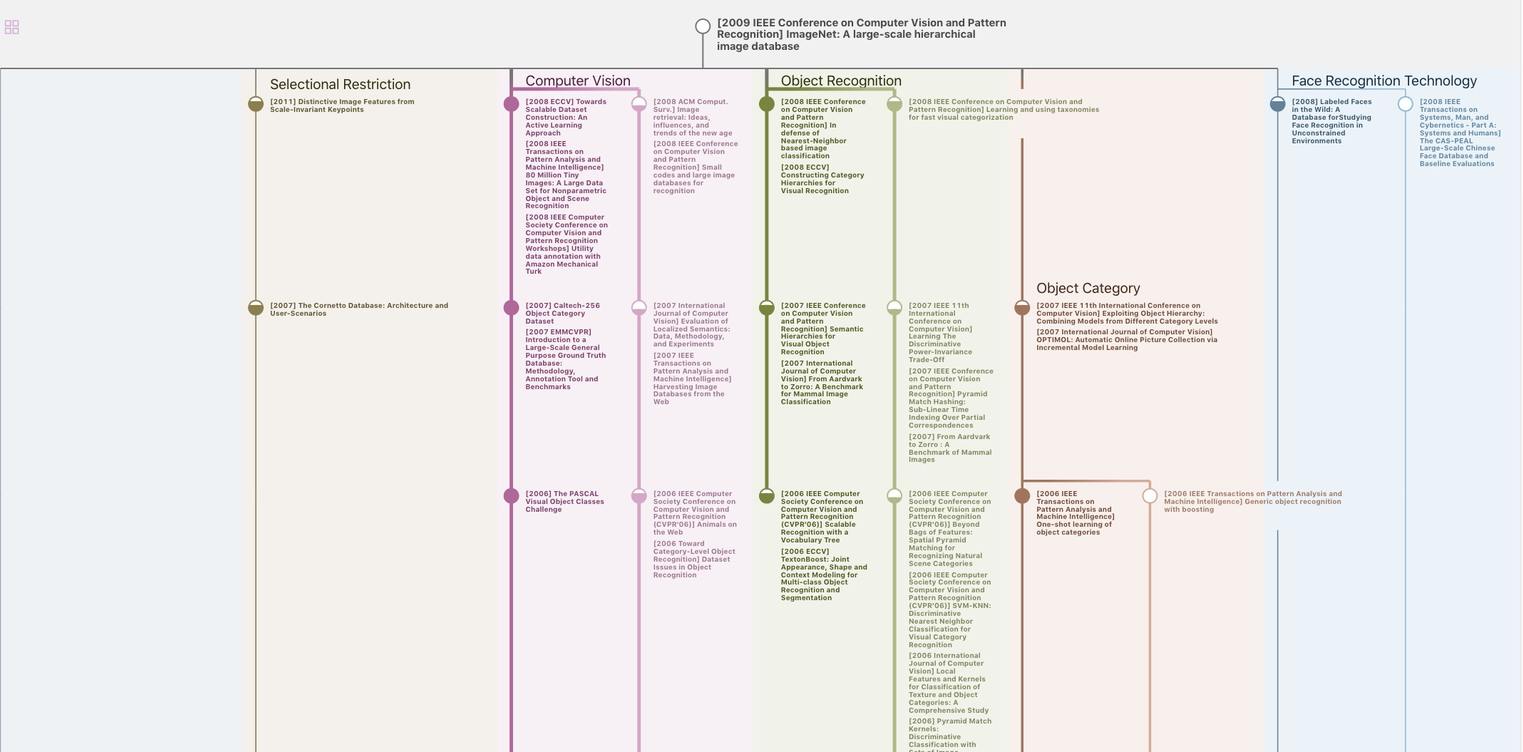
生成溯源树,研究论文发展脉络
Chat Paper
正在生成论文摘要