Wheat Yield Robust Prediction in the Huang-Huai-Hai Plain by Coupling Multi-Source Data with Ensemble Model under Different Irrigation and Extreme Weather Events
REMOTE SENSING(2024)
摘要
The timely and robust prediction of wheat yield is very significant for grain trade and food security. In this study, the yield prediction model was developed by coupling an ensemble model with multi-source data, including vegetation indices (VIs) and meteorological data. The results showed that green chlorophyll vegetation index (GCVI) is the optimal remote sensing (RS) variable for predicting wheat yield compared with other VIs. The accuracy of the adaptive boosting- long short-term memory (AdaBoost-LSTM) ensemble model was higher than the LSTM model. AdaBoost-LSTM coupled with optimal input data had the best performance. The AdaBoost-LSTM model had strong robustness for predicting wheat yield under different irrigation and extreme weather events in general. Additionally, the accuracy of AdaBoost-LSTM for rainfed counties was higher than that for irrigation counties in most years except extreme years. The yield prediction model developed with the characteristic variables of the window from February to April had higher accuracy and smaller data requirements, which was the best prediction window. Therefore, wheat yield can be accurately predicted by the AdaBoost-LSTM model one to two months of lead time before maturity in the HHHP. Overall, the AdaBoost-LSTM model can achieve accurate and robust yield prediction in large-scale regions.
更多查看译文
关键词
wheat,Huang-Huai-Hai Plain,ensemble model,vegetation indices,yield prediction
AI 理解论文
溯源树
样例
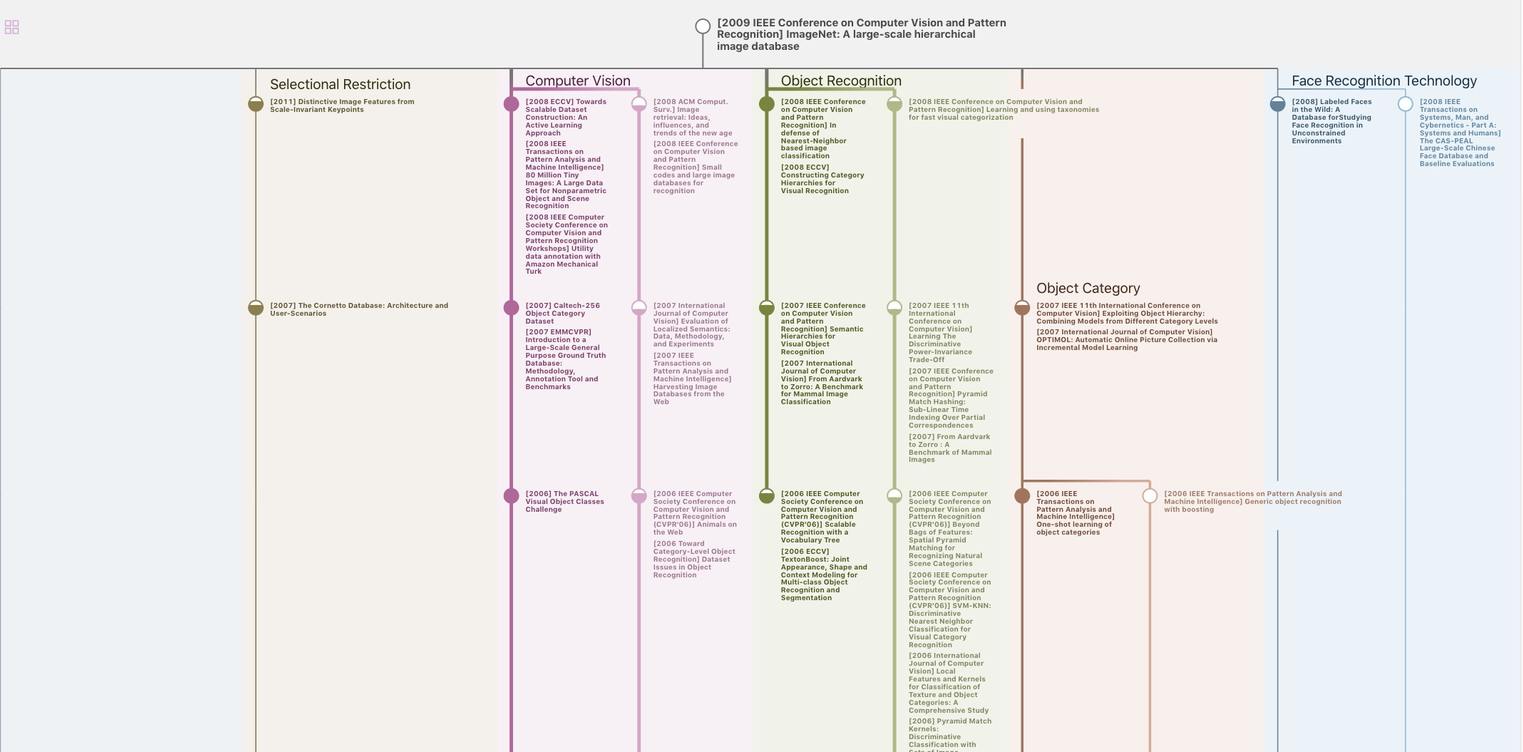
生成溯源树,研究论文发展脉络
Chat Paper
正在生成论文摘要