Efficient Economic Energy Scheduling in Smart Cities Using Distributed Energy Resources
Science and Technology for Energy Transition(2024)
摘要
Machine learning provides a powerful mechanism to enhance the capabilities of the next generation of smart cities. Whether it is healthcare monitoring, building automation, energy management, or traffic management, use cases of capability enhancement using machine learning have been significant in recent years. This paper aims to propose a modeling approach for scheduling energy consumption within smart homes, based on a non-dominated sorting genetic algorithm (NSGA). Distributed energy management plays a significant role in reducing energy consumption and carbon emissions as com- pared to centralized energy generation. Multiple energy consumers can schedule energy-consuming household tasks using home energy management systems in coordination to reduce economic costs and greenhouse gas emissions. In this work, such a home energy management system is used to collect energy price data from the electricity company via an embedded device-enabled smart meter and schedule energy consumption tasks based on this data. We schedule daily power consumption tasks based on a multi-objective optimization method that considers environmental and economic sustainability. There are two conflicting objectives: minimizing daily energy costs and reducing carbon dioxide emissions. Based on electricity tariffs, CO2 intensity, and the window of time during which electricity is consumed, energy consumption tasks involving distributed energy resources (DERs) and electricity consumption are scheduled. An implementation of the proposed model is undertaken in a model smart building consisting of thirty homes under three pricing schemes. A Pareto curve illustrates the trade-off between cost and CO2 emissions
更多查看译文
AI 理解论文
溯源树
样例
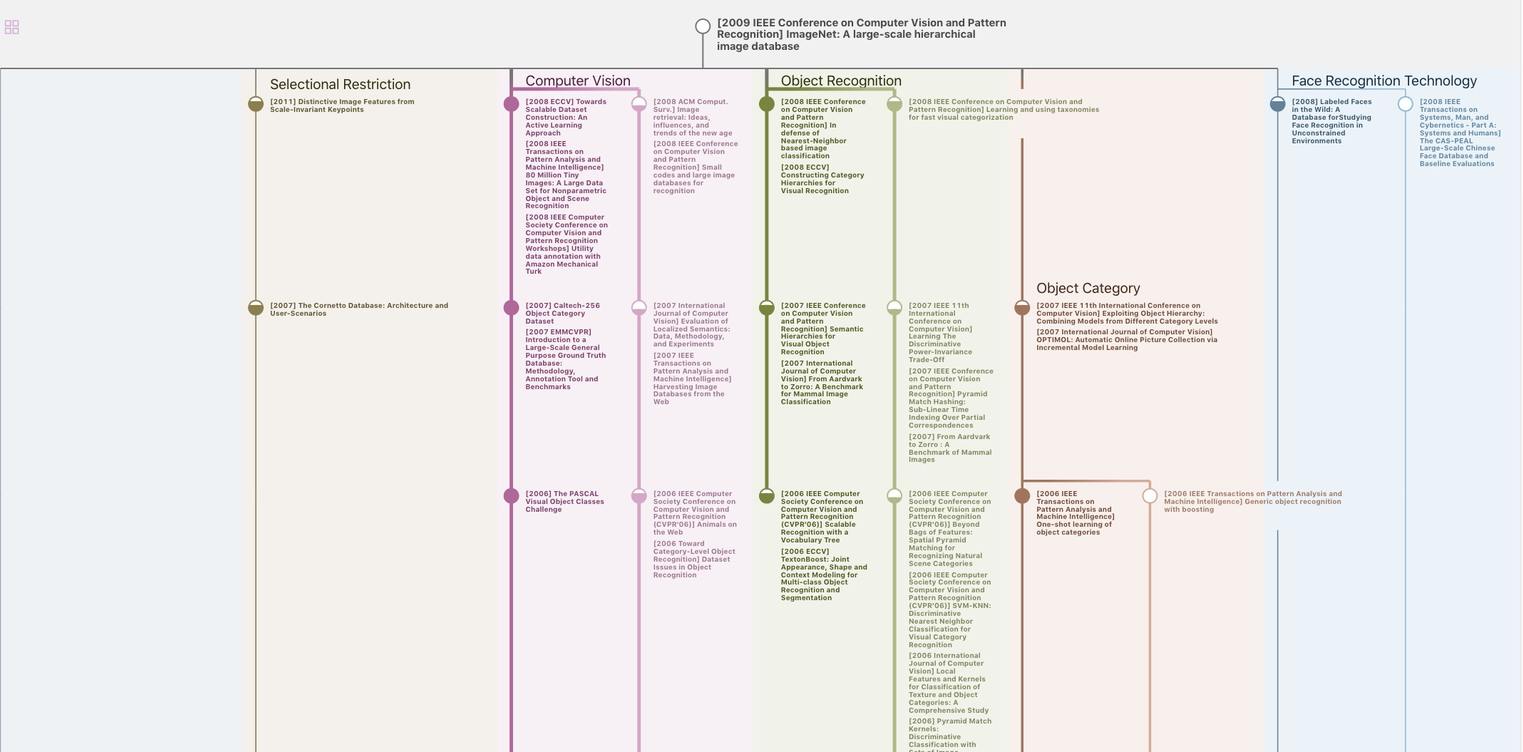
生成溯源树,研究论文发展脉络
Chat Paper
正在生成论文摘要