One Size Does Not Fit All: Idiographic Computational Models Reveal Individual Differences in Learning and Meta-Learning Strategies
TOPICS IN COGNITIVE SCIENCE(2024)
摘要
Complex skill learning depends on the joint contribution of multiple interacting systems: working memory (WM), declarative long-term memory (LTM) and reinforcement learning (RL). The present study aims to understand individual differences in the relative contributions of these systems during learning. We built four idiographic, ACT-R models of performance on the stimulus-response learning, Reinforcement Learning Working Memory task. The task consisted of short 3-image, and long 6-image, feedback-based learning blocks. A no-feedback test phase was administered after learning, with an interfering task inserted between learning and test. Our four models included two single-mechanism RL and LTM models, and two integrated RL-LTM models: (a) RL-based meta-learning, which selects RL or LTM to learn based on recent success, and (b) a parameterized RL-LTM selection model at fixed proportions independent of learning success. Each model was the best fit for some proportion of our learners (LTM: 68.7%, RL: 4.8%, Meta-RL: 13.25%, bias-RL:13.25% of participants), suggesting fundamental differences in the way individuals deploy basic learning mechanisms, even for a simple stimulus-response task. Finally, long-term declarative memory seems to be the preferred learning strategy for this task regardless of block length (3- vs 6-image blocks), as determined by the large number of subjects whose learning characteristics were best captured by the LTM only model, and a preference for LTM over RL in both of our integrated-models, owing to the strength of our idiographic approach. Individuals rely on different strategies-combination of declarative memory and procedural memory-to learn new associations. Idiographic computational models were used to capture these differences.
更多查看译文
关键词
Individual differences,Learning,ACT-R,Reinforcement learning,Working memory,Declarative memory
AI 理解论文
溯源树
样例
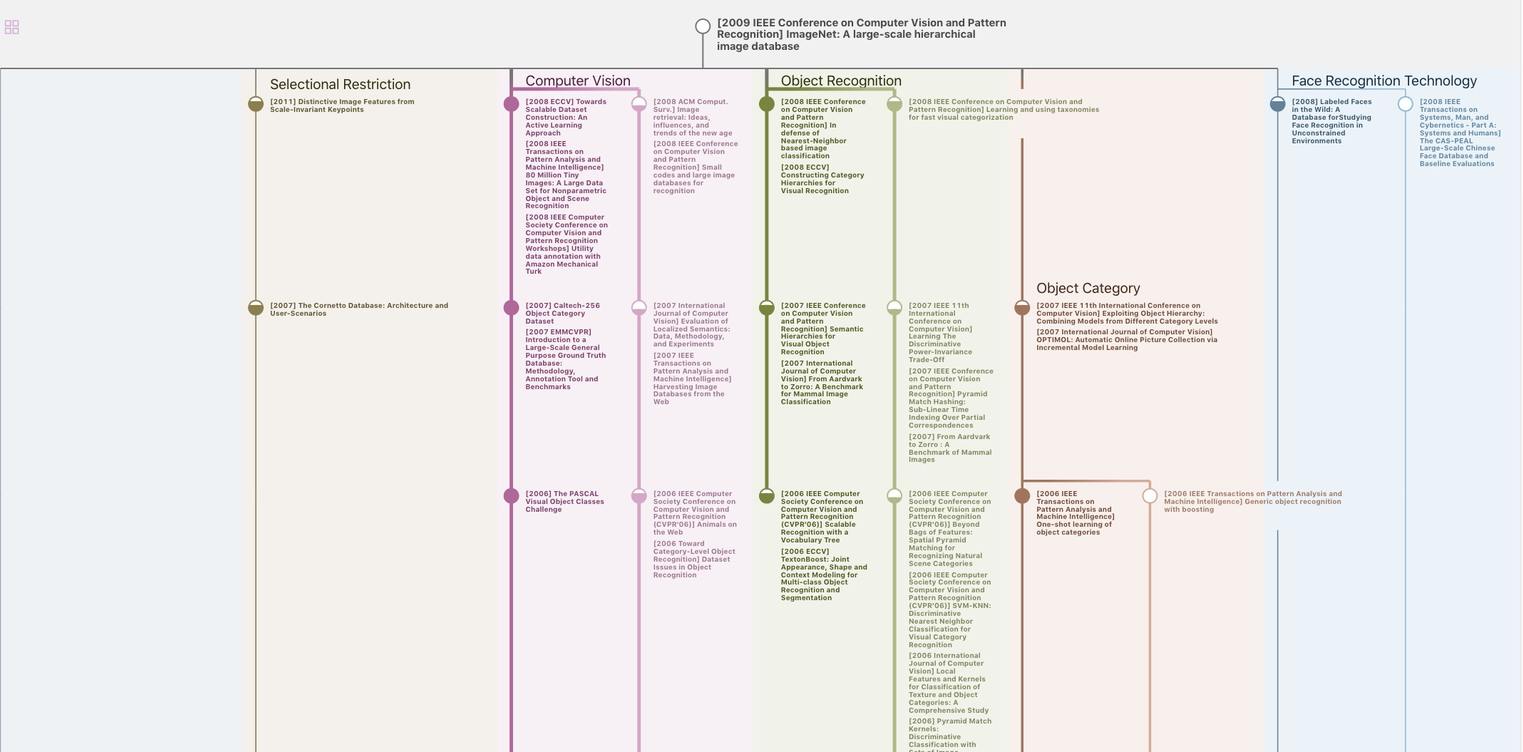
生成溯源树,研究论文发展脉络
Chat Paper
正在生成论文摘要