Deep Precipitation Nowcasting With Dual Regions Displacement Information and Global Spatiotemporal Representations Learning
IEEE GEOSCIENCE AND REMOTE SENSING LETTERS(2024)
摘要
The deep precipitation nowcasting using radar echo map prediction can mitigate the socio-economic impact of extreme precipitation events. Existing methods employ long short-term memory (LSTM) to extract rich precipitation features. However, existing methods often combine the learning and modeling of rain and nonrain regions in a single module, without clearly distinguishing their different features and motion patterns, which impairs the spatial distribution and precipitation intensity prediction of rainfall. Moreover, these LSTMs only capture local spatiotemporal features, while ignoring the global spatiotemporal features, resulting in prediction results lacking structural and strength consistency. Therefore, we propose a dual regions center displacement (DRCD) module, which separately learns and models the spatial information of rainfall and nonrainfall regions and employs this module to estimate the locations and intensity residuals of the future regions. Moreover, we also introduce a novel Global LSTM module (GLSTM) that learns the global spatiotemporal features from the sequences, which can estimate the structure and intensity of dual regions. Extensive experiments demonstrate that our method has superior or competitive performance over the state-of-the-art precipitation nowcasting methods and has the potential to be implemented as an alternative product globally.
更多查看译文
关键词
Rain,Spatiotemporal phenomena,Decoding,Radio frequency,Feature extraction,Kalman filters,Radar,Global spatiotemporal features,locations and intensity residuals,precipitation nowcasting,radar echo map prediction
AI 理解论文
溯源树
样例
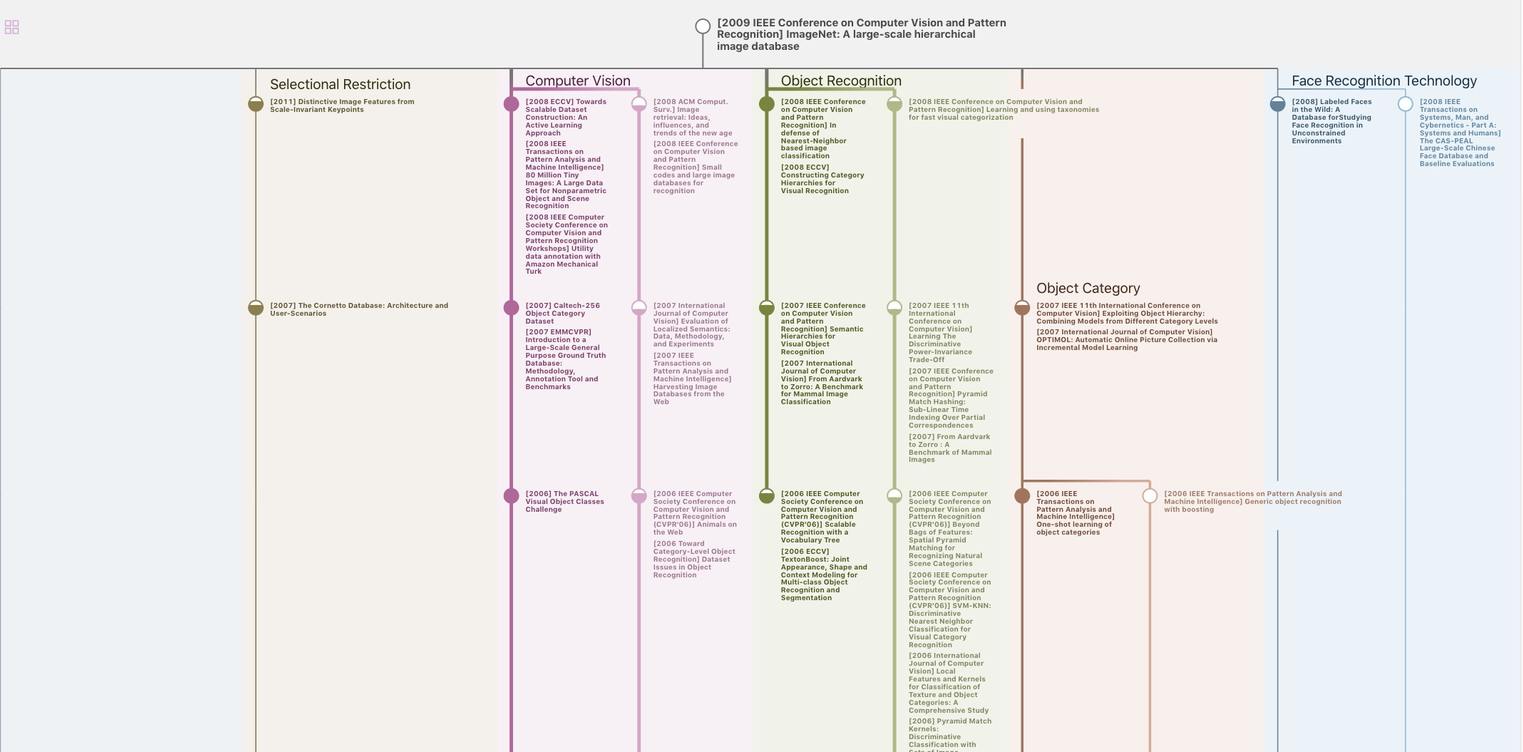
生成溯源树,研究论文发展脉络
Chat Paper
正在生成论文摘要