PHD-NAS: Preserving helpful data to promote Neural Architecture Search
Neurocomputing(2024)
摘要
Neural Architecture Search (NAS) has achieved promising results in many domains. However, the enormous computational burden consumed by the NAS procedure significantly hinders its application. Existing works focus on mitigating the search cost by either designing a more efficient algorithm or searching in an elaborately designed search space, which heavily rely on expert experience and domain knowledge. We notice that few works focus on dataset optimization for NAS, however, the truth is that not all samples are essential for the search process, which can be omitted actually. Therefore, we propose to only preserve helpful data for the supernet training to improve the searching efficiency. Specifically, we compute the forgetting and remembering events for each sample during the supernet training to determine the data importance. Samples that the supernet has predicted correctly in consecutive epochs have low importance and will be gradually removed from the dataset during training. We further formulate our method into a unified cycled-learning framework for jointly optimizing proxy dataset and architecture search. By combining with different search algorithms, we demonstrate that our framework can find architectures with comparable performance using much less training data and search time in various search spaces and benchmark datasets, validating the effectiveness of our method.
更多查看译文
关键词
Neural architecture search,Dataset optimization,Forgetting events and remembering events
AI 理解论文
溯源树
样例
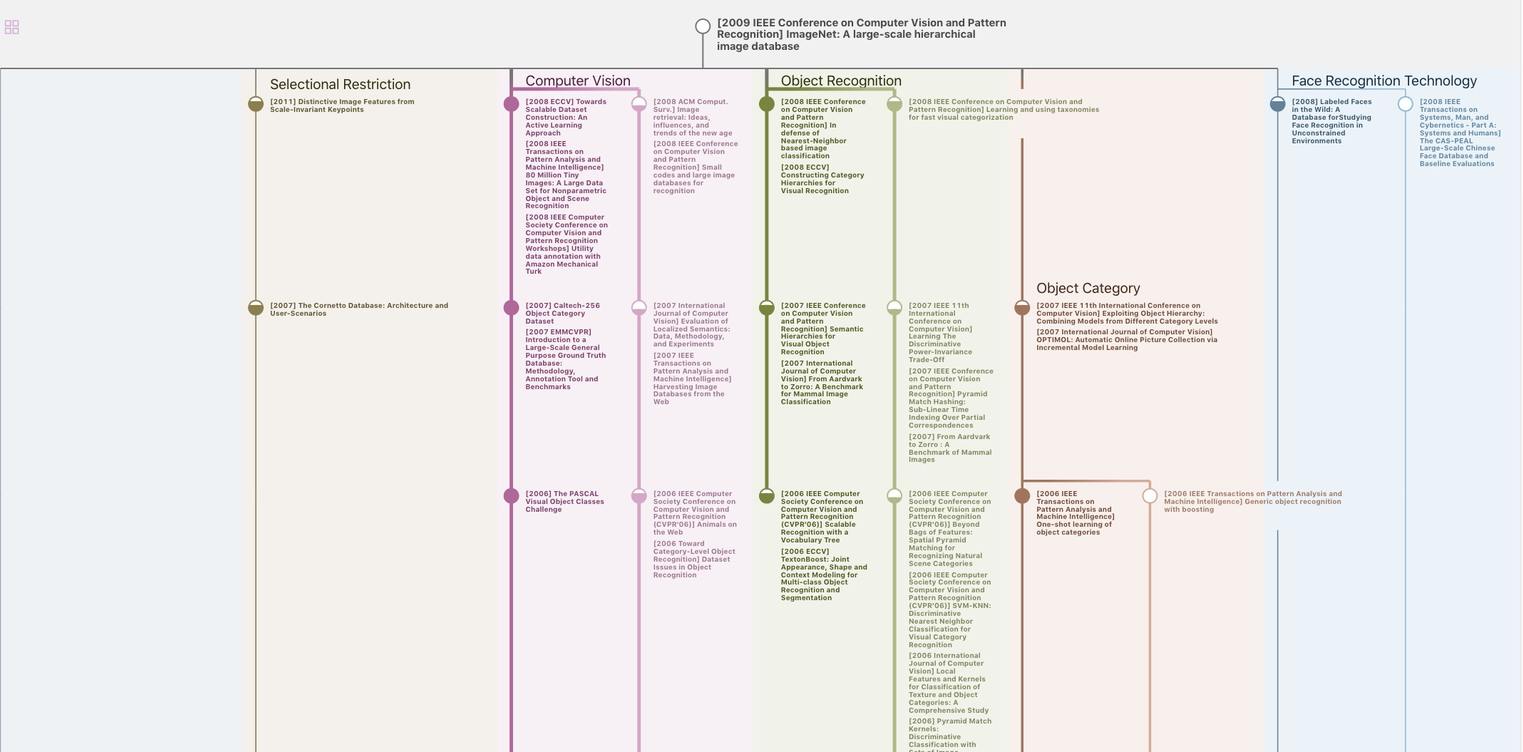
生成溯源树,研究论文发展脉络
Chat Paper
正在生成论文摘要