Alignment and fusion for adaptive domain nighttime semantic segmentation
Image and Vision Computing(2024)
摘要
In the field of autonomous driving technology, both daytime and nighttime scenes are common. However, due to the poor illumination and difficulty in manual annotation of nighttime images, semantic segmentation of nighttime scenes is more challenging compared to daytime scenes. Therefore, achieving significant progress in nighttime semantic segmentation would greatly enhance the effectiveness of the application of autonomous driving scenarios. In this work, we investigate the problem of domain-adaptive nighttime semantic segmentation (DANSS). The problem aims to learn semantic segmentation in nighttime scenes by leveraging a labeled Cityscapes dataset and unlabeled but roughly aligned day-night image pairs. To address this, we propose an Align and Fusion Network (AAFnet), a network for adaptive domain nighttime semantic segmentation. AAFnet utilizes a novel DAFormer as the backbone to separately compute features for dynamic and static objects during the training process. It also employs methods such as small category sampling and image blending to improve learning effectiveness. Additionally, we propose utilizing local image patches to enhance the results during the testing process. Experimental results demonstrate a significant improvement of 10.4% compared to the previous method, DANNet. Extensive experiments conducted on the Dark Zurich dataset and Nightdriving dataset validate the effectiveness of the proposed approach. Our method outperforms previous backbones and achieves top-ranking results.
更多查看译文
关键词
Vision transformer,Small category sampling,Image blending,Local image patches
AI 理解论文
溯源树
样例
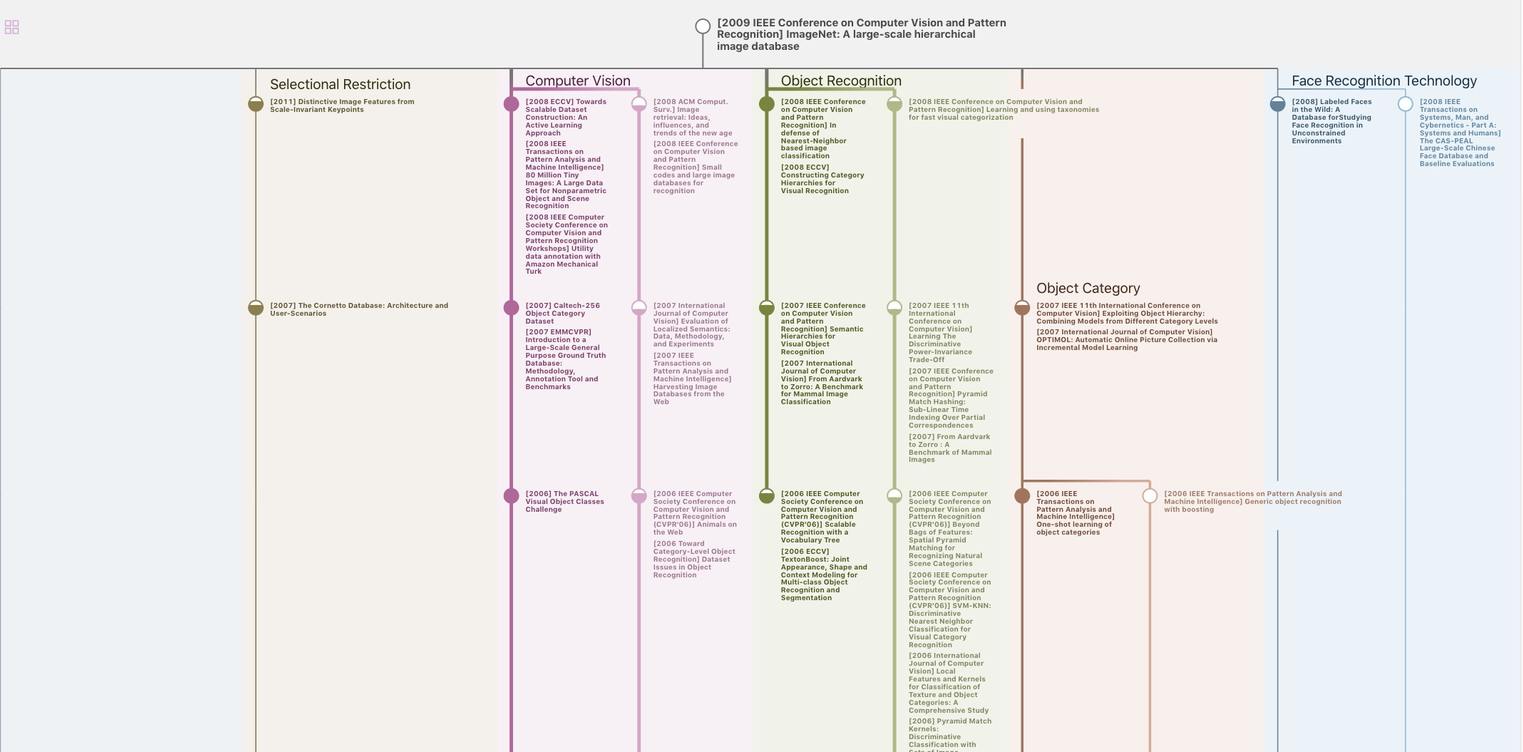
生成溯源树,研究论文发展脉络
Chat Paper
正在生成论文摘要