Estimating Soil Organic Carbon using Sentinel-2 data under Zero Tillage Agriculture: A Machine Learning Approach
crossref(2024)
摘要
Abstract Soil organic carbon (SOC) is the main component of soil organic matter (SOM) and constitutes the crucial ingredient of the soil. It supports key soil functions, stabilizes soil structure, aid in plant nutrients retention and release, and promote water infiltration and storage. Predicting SOC using Sentinel-2 images integrated with machine learning algorithms under zero tillage practice is inadequately documented for developing countries like Zimbabwe. The purpose of this study is to evaluate the integrated use of Sentinel-2 data together with machine learning (ML) algorithms for SOC estimation. The support vector machine (SVM), artificial neural network (ANN), and partial least square regression (PLSR) models were used with a cross-validation to estimate the SOC content based on 50 georeferenced calibration samples under a zero-tillage practice. The ANN model outperformed the other two models by delivering a coefficient of determination (R2) of between 55-60% of SOC variability and RMSE varies between 5.01-8.78%, whereas for the SVM, R2 varies between 0.53-0.57 and RMSE varies between 6.25-11.39%. The least estimates of SOC are provided by the PLSR algorithm (R2 = 0.44–0.49 and RMSE = 7.59-12.42% for the top 15cm depth SOC estimation. Results with RMSE, MAE and R2 for SVM, ANN and PLSR, show that the ANN model is able to capture SOC variability. Although the ANN algorithm provides more accurate SOC estimates than the SVM algorithm, the difference in accuracy is not significant. Results revealed a satisfactory agreement between the SOC and zero tillage practice (R2, coefficient of variation (CV), mean absolute error (MAE), and root mean square error (RMSE) using SVM, ANN and PLSR for the validation dataset using four predictor variables. The calibration results of SOC indicated that the mean SOC was 5.19% and the validation mean SOC was 5.02%. The calibration dataset had higher degree of variation (27.94%) around its mean compared to the validation dataset (24.30%). The accurate map results can be used as an important tool for informed decisions about soil health and productivity by the farmers, land managers and policy makers.
更多查看译文
AI 理解论文
溯源树
样例
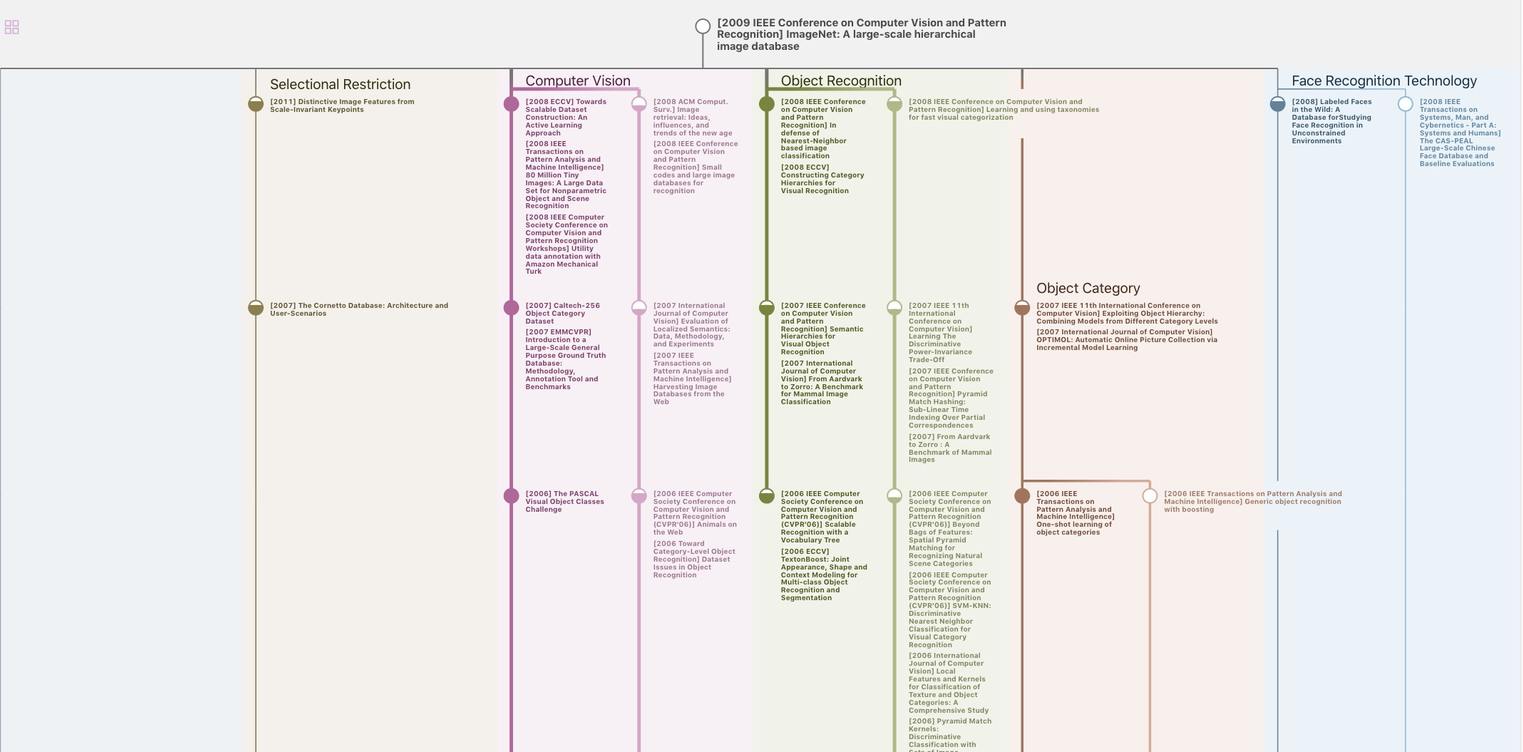
生成溯源树,研究论文发展脉络
Chat Paper
正在生成论文摘要